On Object Detection and Explainability with Sonar Imagery
2024 IEEE 4th International Maghreb Meeting of the Conference on Sciences and Techniques of Automatic Control and Computer Engineering (MI-STA)(2024)
Abstract
The task of detecting objects in sonar imagery is challenging due to low image resolution, significant noise, and the variable nature of the underwater environment. Artificial Intelligence (AI) methods, such as convolutional neural networks and transformers, can be applied to address these challenges. However, the lack of transparent explanations for the results of deep learning models is a major stumbling block to building trust in these models. This paper evaluates You Only Look Once (YOLO-v8) and DEtection TRansformers (DETR) models, as two prominent tools for object detection, using two sonar datasets as a part of the Hybrid Artificial Intelligence eXplainer (HAI-x) project. The selected datasets include a dataset of sonar raw images and another with pre-processed sonar images. Contrary to the claim that sonar raw images do not require preprocessing for efficient object detection using deep learning techniques, the experiments conducted demonstrate that such preparatory steps can indeed improve the performance of object detectors. Furthermore, they can provide an understandable common ground for explaining the detection process to end users.
MoreTranslated text
Key words
Object Detection,SONAR,XAI,YOLOv8,DETR,ViT
AI Read Science
Must-Reading Tree
Example
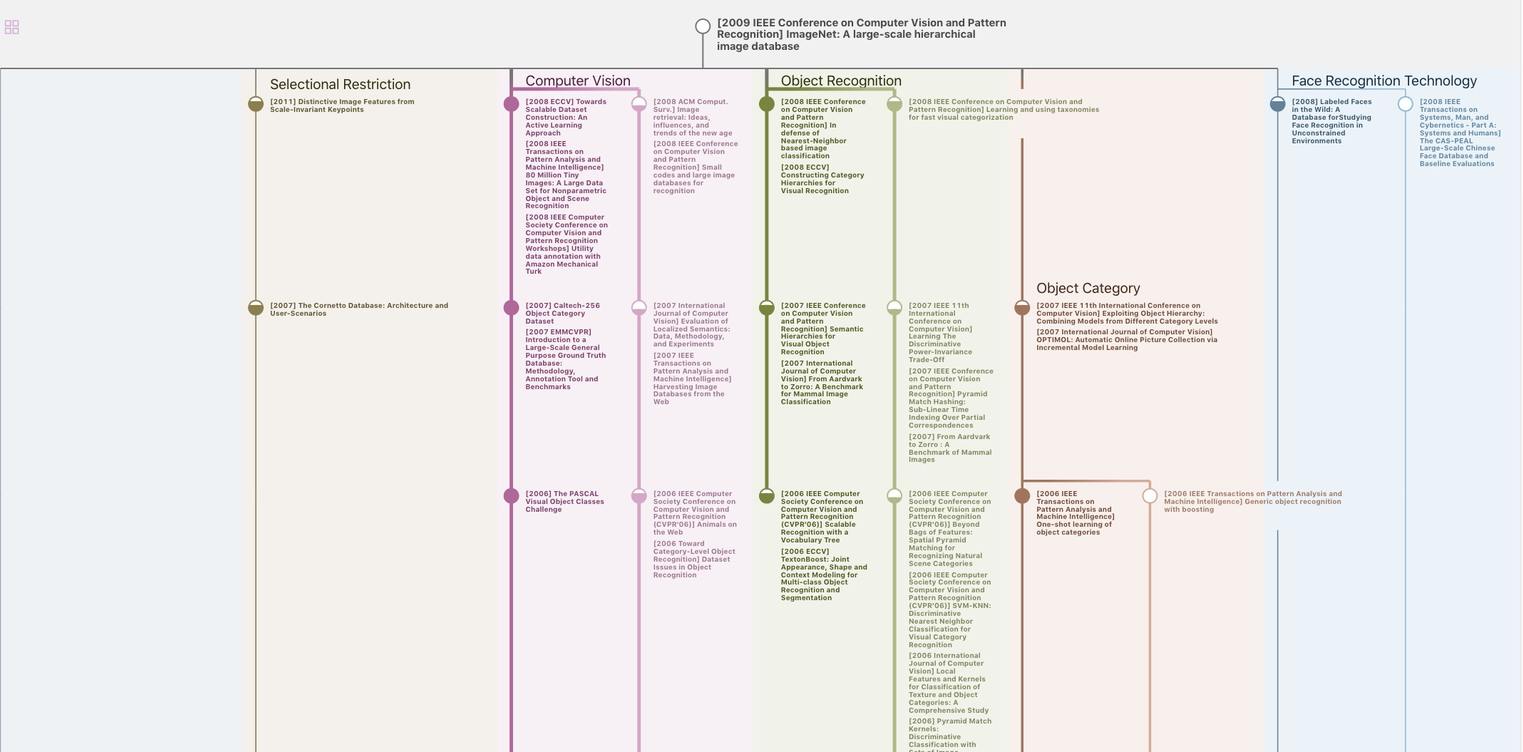
Generate MRT to find the research sequence of this paper
Chat Paper
Summary is being generated by the instructions you defined