Drug repositioning via Multi-view Representation Learning with Heterogeneous Graph Neural Network.
IEEE journal of biomedical and health informatics(2024)
Abstract
Exploring simple and efficient computational methods for drug repositioning has emerged as a popular and compelling topic in the realm of comprehensive drug development. The crux of this technology lies in identifying potential drug-disease associations, which can effectively mitigate the burdens caused by the exorbitant costs and lengthy periods of conventional drugs development. However, current computational drug repositioning methods face challenges in accurately predicting drug-disease associations. These challenges include only considering drugs and diseases to construct a heterogeneous graph without including other biological nodes associated with the disease or drug for a more comprehensive heterogeneous graph, as well as not fully utilizing the local structure of heterogeneous graphs and rich semantic features. To address these problems, we propose a Multi-view Representation Learning method (MRLHGNN) with Heterogeneous Graph Neural Network for drug repositioning. This method is based on a collection of data from multiple biological entities associated with drugs or diseases. It consists of a view-specific feature aggregation module with meta-paths and auto multi-view fusion encoder. To better utilize local structural and semantic information from specific views in heterogeneous graph, MRLHGNN employs a feature aggregation model with variable-length meta-paths to expand the local receptive field. Additionally, it utilizes a transformerbased semantic aggregation module to aggregate semantic features across different view-specific graphs. Finally, potential drug-disease associations are obtained through a multi-view fusion decoder with an attention mechanism. Cross-validation experiments demonstrate the effectiveness and interpretability of the MRLHGNN in comparison to nine state-of-the-art approaches. Case studies further reveal that MRLHGNN can serve as a powerful tool for drug repositioning.
MoreTranslated text
Key words
Drug,Disease,Drug repositioning,Multiview Representation Learning,Heterogeneous Graph Neural Network,Meta-path
AI Read Science
Must-Reading Tree
Example
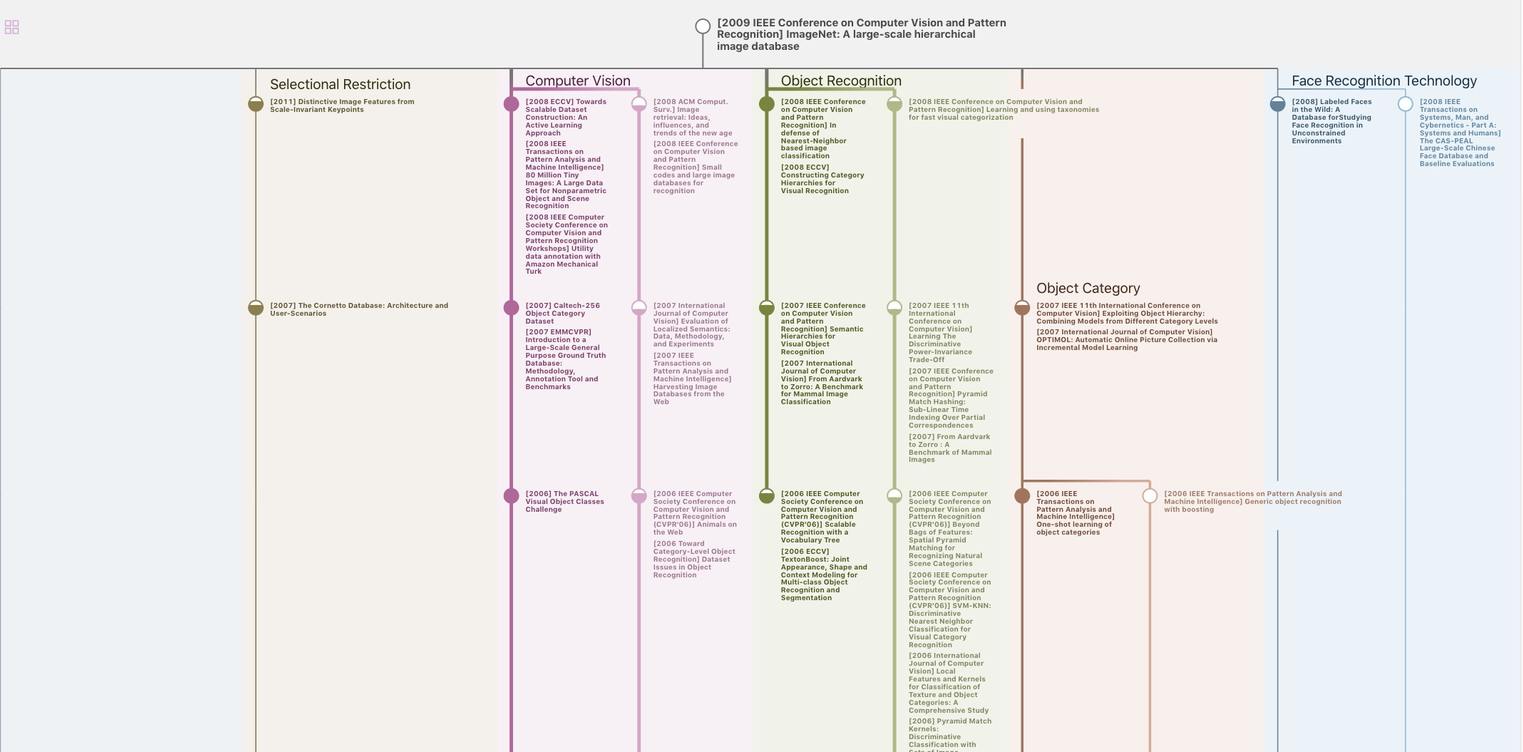
Generate MRT to find the research sequence of this paper
Chat Paper
Summary is being generated by the instructions you defined