M-DIVO: Multiple ToF RGB-D Cameras Enhanced Depth-Inertial-Visual Odometry
IEEE Internet of Things Journal(2024)
摘要
Time of flight (ToF) RGB-D cameras provide a wealth of information for SLAM systems. However, the limited field of view (FOV) of a single ToF RGB-D camera and the small range of its depth measurement module make it prone to degeneracy when relying solely on visual or depth information for SLAM, a problem typical of unimodal SLAM algorithms. To address this issue, this paper presents M-DIVO: an IEKF-based odometry that fuses visual, depth (similar to LiDAR), and inertial modules from multiple ToF RGB-D cameras. It comprises two direct method subsystems: the depth inertial odometry (DIO) subsystem, which constructs point-to-plane constraints from multiple depth modules, and the visual-inertial odometry (VIO) subsystem, which optimizes pose using photometric error constructed by multiple cameras. Additionally, to manage the significant computational load from processing multiple sensors and multimodal information, we introduce a multimodal redundancy scheduling mechanism (MRSM): prioritizing the DIO subsystem with the VIO subsystem as auxiliary, executing the VIO subsystem only when degeneracy occurs in the DIO subsystem. We also propose a “External First, Internal Last" strategy for calibrating multiple external and internal sensors. Experiments demonstrate that compared to unimodal SLAM, our method achieves higher robustness and precision, as well as satisfactory real-time performance. The proposed calibration strategy is demonstrated to be more accurate than the traditional IMU-centric approach. The code is open source.
更多查看译文
关键词
ToF RGB-D camera,SLAM,degeneracy,multimodal,calibration
AI 理解论文
溯源树
样例
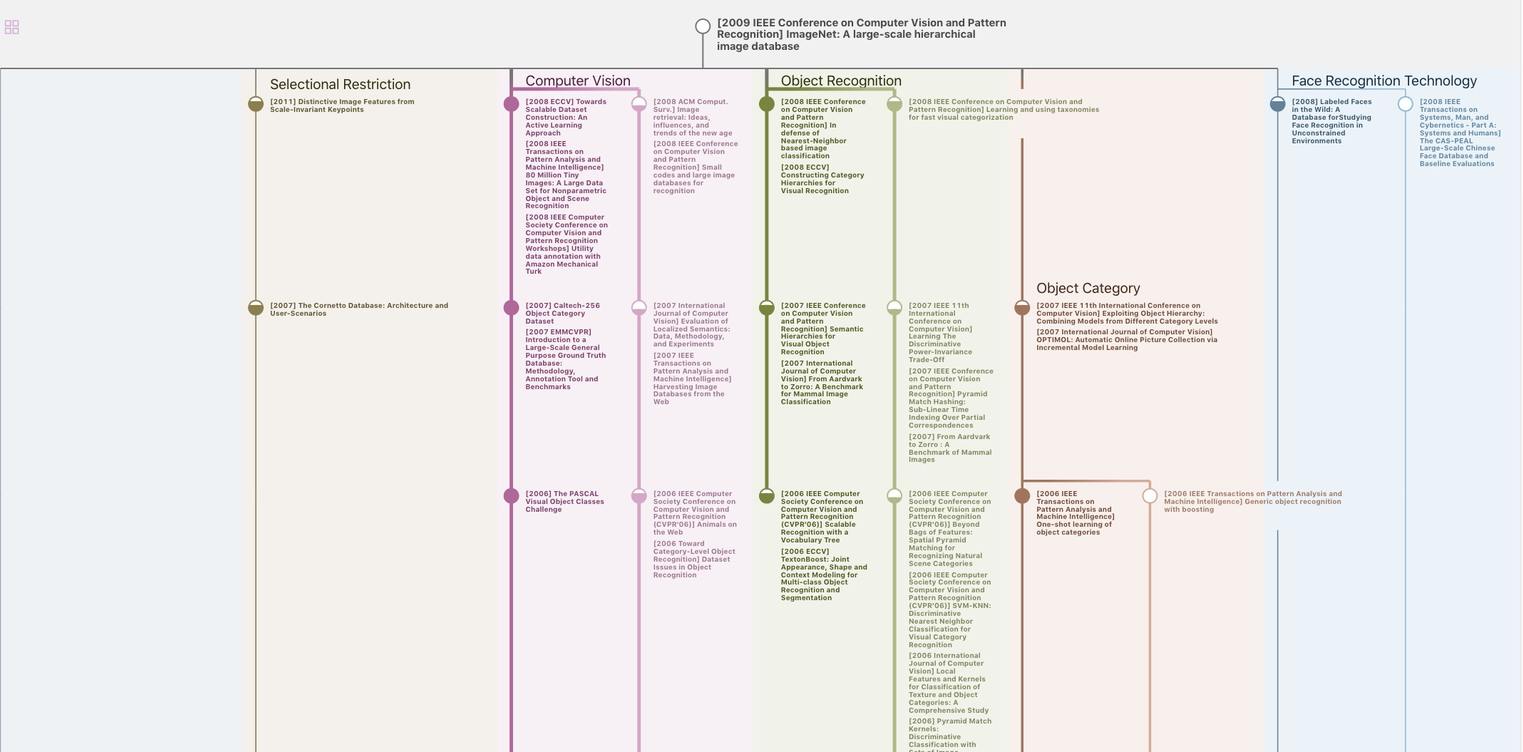
生成溯源树,研究论文发展脉络
Chat Paper
正在生成论文摘要