A generalized grey model with symbolic regression algorithm and its application in predicting aircraft remaining useful life
Engineering Applications of Artificial Intelligence(2024)
摘要
As a sparse data analysis method, a grey model faces challenges in interpretability for its effective application in uncertain systems. This study proposes a generalized grey model (GGM) based on symbolic regression, designed to improve the intelligence and adaptability of grey models. The GGM serves as a unified framework, integrating various grey model families and addresses regression challenges to determine the model structure. Symbolic regression in the GGM identifies symbolic input-output relationships, offering an interpretable approach for structure determination. By leveraging the non-uniqueness principle in grey system theory and employing structural penalty parameters, the model balances complexity and interpretability. A comparative analysis between GGM and conventional grey function models is conducted focusing on the differences in modeling, structure identification, and parameter optimization. Validation on the M3 competition dataset demonstrated the GGM's superior performance, achieving a significant reduction in prediction error compared to other grey forecasting models. Additionally, a rigorous analysis of aircraft lifespan data underscored the robustness and accuracy of GGM in practical engineering applications.
更多查看译文
关键词
Forecasting algorithm,Structure identification,Time series,Model interpretability,Grey system theory
AI 理解论文
溯源树
样例
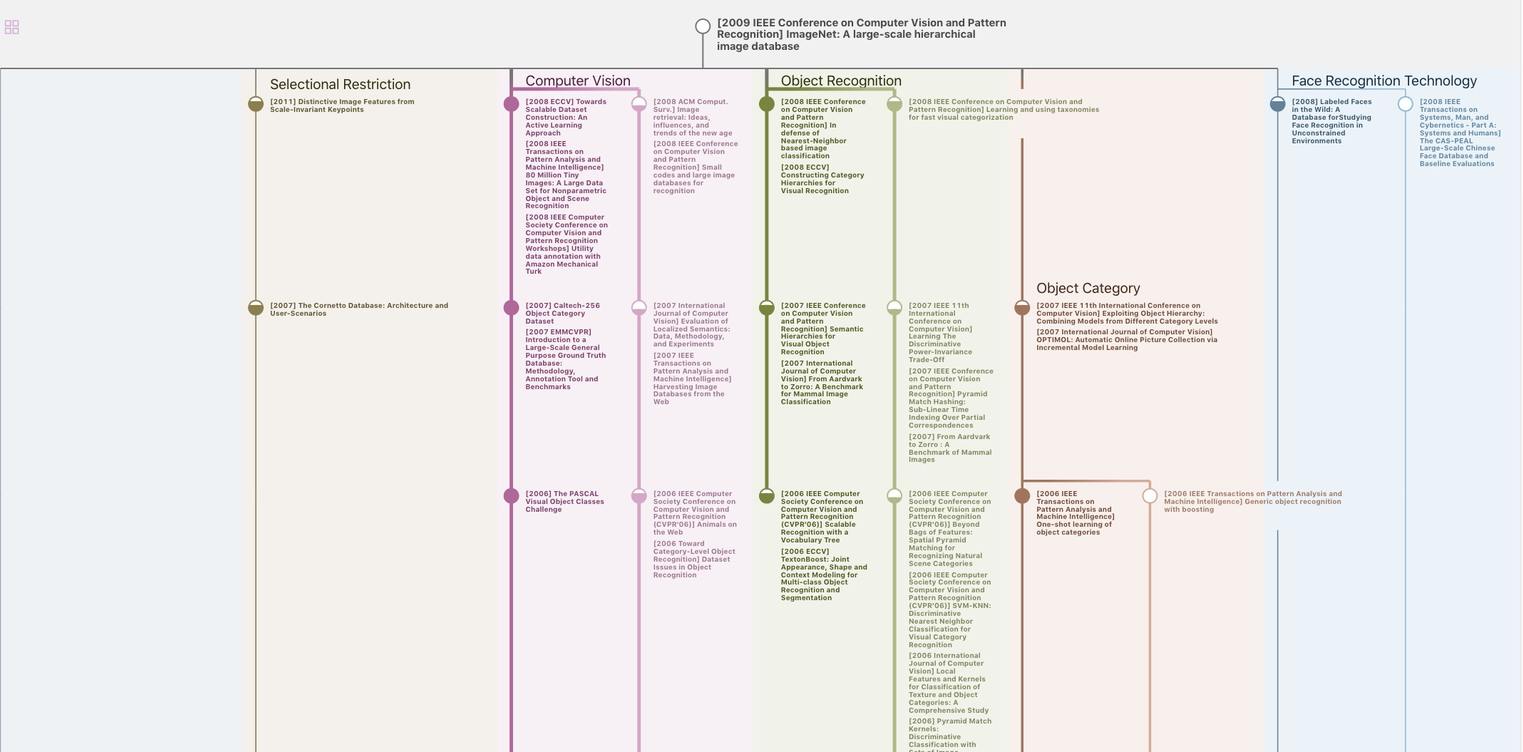
生成溯源树,研究论文发展脉络
Chat Paper
正在生成论文摘要