Synergistic fusion of physical modeling and data-driven approaches for parameter inference to enzymatic biodiesel production system
Applied Energy(2024)
摘要
Enzymatic biodiesel production systems represent complex chemical dynamical processes that traditional modeling approaches struggle to effectively capture the inherent nonlinearity and dynamics. We propose a novel framework that fuses prior physical knowledge and data-driven methods to enhance parameter inference capabilities for such systems. Grounded in the concept of physics-informed machine learning (PIML), we combine the prior knowledge from traditional physical models with the powerful approximation abilities of data-driven neural networks. This framework enables the physical laws to guide the training of the data-driven model while utilizing data to correct model biases, achieving tight integration of physical constraints and data-driven approaches in the modeling process. To address potential challenges faced by PIML methods in practical applications, such as multiscale features and violations of time causality, we introduce several improved strategies. Evaluating our framework on real experimental data from biodiesel production, we validate its accurate estimation of system parameters. This work provides a promising new approach to enhance the interpretability and generalization of data-driven modeling by incorporating prior physical knowledge, holding potential applications in modeling and control of complex dynamical systems.
更多查看译文
关键词
Parameter inference,Biodiesel production,Physics-informed,Data-driven,Time causality,Multiscale
AI 理解论文
溯源树
样例
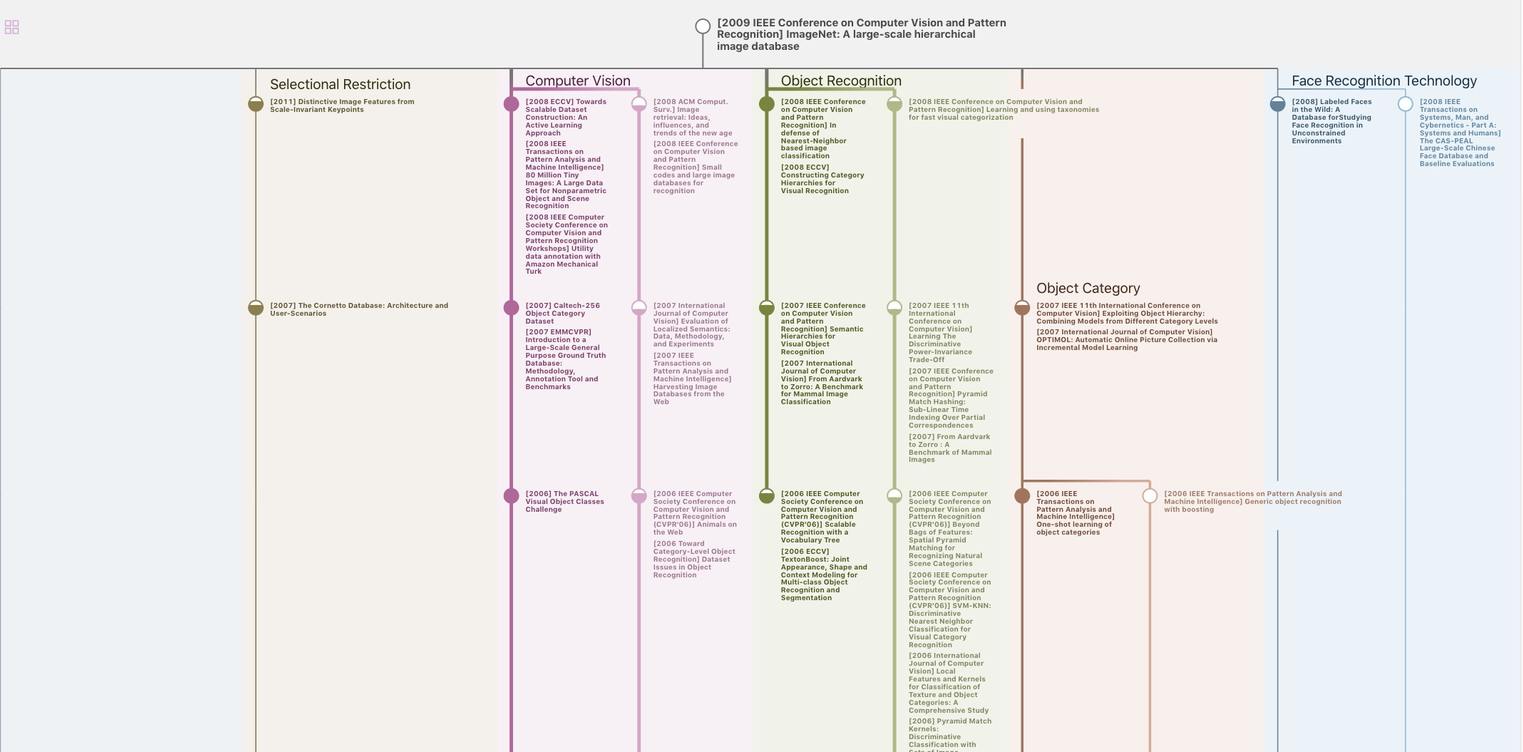
生成溯源树,研究论文发展脉络
Chat Paper
正在生成论文摘要