Self-supervised reconstructed graph learning for link prediction in bipartite graphs
Neurocomputing(2024)
摘要
Graph Neural Network(GNN) has achieved remarkable performance in classification tasks due to its strong distinctive power of different graph topologies. However, traditional GNNs face great limitations in link prediction tasks since they learn vertex embeddings from fixed input graphs thus the learned embeddings cannot reflect unobserved graph structures. Graph-learning based GNNs have shown better performance by collaborative learning of graph structures and vertex embeddings, but most of them rely on available initial features to refine graphs and almost perform graph learning at once. Recently, some methods utilizes contrastive learning to facilitate link prediction, but their graph augmentation strategies are predefined only on original graphs, and do not introduce unobserved edges into augmented graphs. To this end, a self-supervised reconstructed graph learning (SRGL) method is proposed. The key points of SRGL lie in two folds: Firstly, it generates augmented graphs for contrasting by learning reconstructed graphs and vertex embeddings from each other, which brings unobserved edges into augmented graphs. Secondly, it maximizes the mutual information between edge-level embeddings of reconstructed graphs and the graph-level embedding of an original graph, which guarantees learned reconstructed graphs to be relevant to the original graph.
更多查看译文
关键词
Graph learning,Contrastive learning,Link prediction,Bipartite graphs
AI 理解论文
溯源树
样例
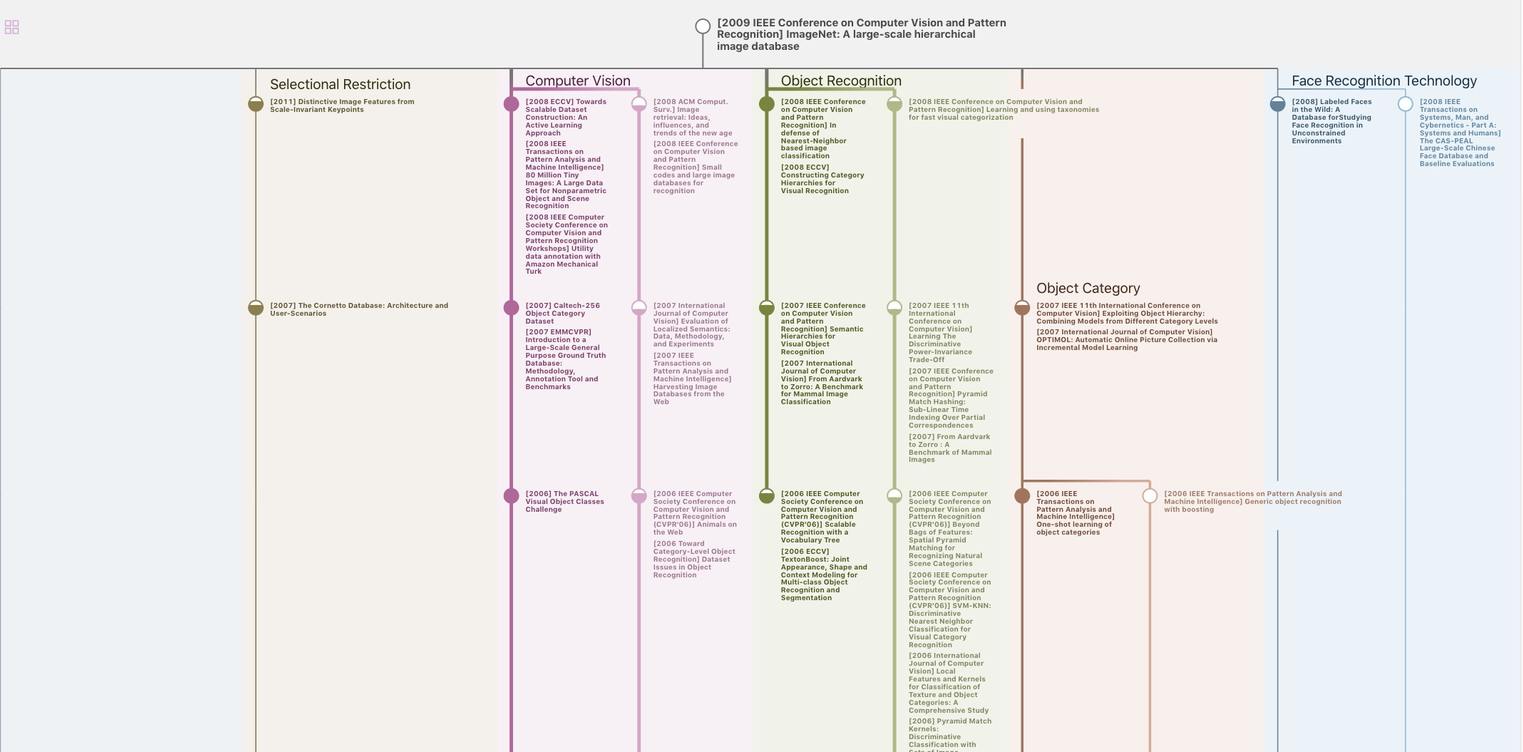
生成溯源树,研究论文发展脉络
Chat Paper
正在生成论文摘要