Energy-saving potential benchmarking method of office buildings based on probabilistic forecast
Journal of Building Engineering(2024)
Abstract
Building energy consumption represents a substantial portion of China's total energy usage profile. Conducting a quantitative assessment of the energy-saving potential in buildings is crucial, as it aids in estimating the investment risk and payback period for energy-efficient projects. This evaluation also informs the development and formulation of policies promoting building retrofits and energy conservation. However, this field's present state of research exhibits a significant gap. This study takes 8 typical office buildings as case objects and conducts relevant research on quantitative evaluation of energy-saving potential. . On the basis of quantile regression, three corresponding probabilistic forecasting model for building energy consumption are established by combining the classic time series prediction algorithm Long Short-Term Memory (LSTM) in data-driven methods, Gradient Boosting Decision Tree (GBDT) in known building energy benchmarking, and a relatively simple linear model. The evaluation results demonstrate that the LSTM-QR model can serve effectively as a probabilistic forecasting model for estimating building energy-saving potential. Based on the LSTM-QR model results, a quantitative evaluation method for building energy-saving potential benchmarking is proposed, utilizing linearized Cumulative Distribution Function (CDF) curves. This method can quantitatively assess the energy-saving potential of the studied buildings. By analyzing and comparing these buildings' hourly energy consumption data over the past three years, it is evident that the quantitative scoring results from this method can provide directional guidance for building renovation projects to enhance energy efficiency.
MoreTranslated text
Key words
Building energy efficiency,Energy-saving potential,Energy benchmarking,Probabilistic forecast,Quantile regression
AI Read Science
Must-Reading Tree
Example
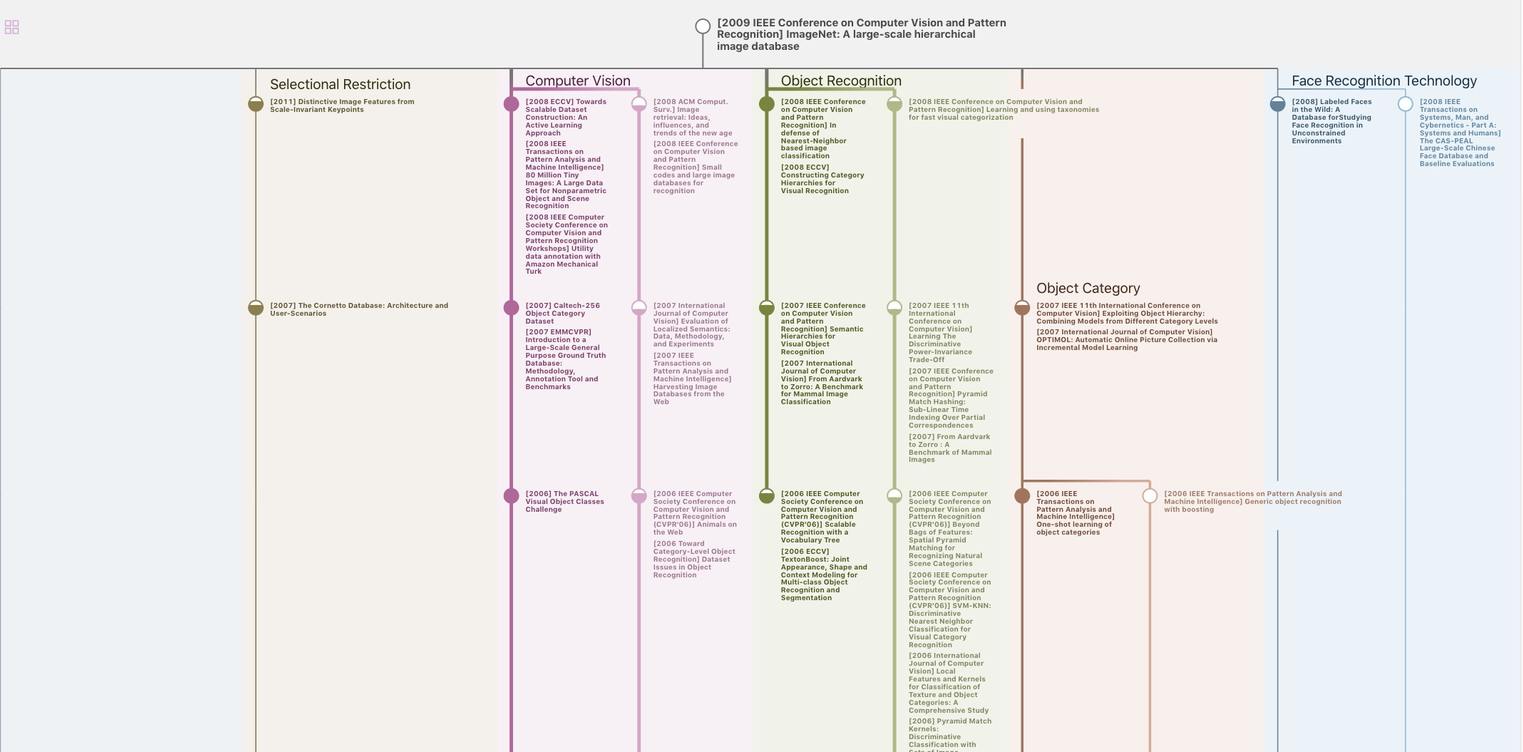
Generate MRT to find the research sequence of this paper
Chat Paper
Summary is being generated by the instructions you defined