Complexity-Efficiency Control with ANN-Based CTU Partitioning for Video Encoding
IEEE Access(2024)
摘要
The application of machine learning to video coding is generally studied in two main approaches: end-to-end video coding using deep neural networks and classic hybrid codecs with individual tools implemented using such networks. This work exploits the latter approach, where a trained Artificial Neural Network (ANN) is used for fast implementation of the search for the partitioning of Coding Tree Units (CTU) into Coding Units (CUs) and Prediction Units (PUs). The proposed approach differs from the previous ones, among other factors, by the application of an ANN with probabilistic soft outputs, which allows for assessing the probability of particular division patterns. This is followed by a decision algorithm that selects more than one candidate division pattern from the most probable patterns provided by ANN. Two variants of the ANN model are considered, out of which the extended one involves deep partitioning at the PU-level. The number of selected division pattern candidates is controlled by a single parameter – uncertainty range – that allows for choosing a trade-off between the complexity and the loss of coding efficiency. This feature is particularly important for broadcasting applications, where processing must be adjusted to the current computational load and resource availability. The experiments demonstrate that the proposed approach yields good results using much smaller ANNs than those described in the references. This makes the proposed approach well-suited for the usage on edge servers where GPU may not be available. The source code for the respective HEVC codec software implementation is provided.
更多查看译文
关键词
CTU Partitioning,encoder control,HEVC,neural network,video compression
AI 理解论文
溯源树
样例
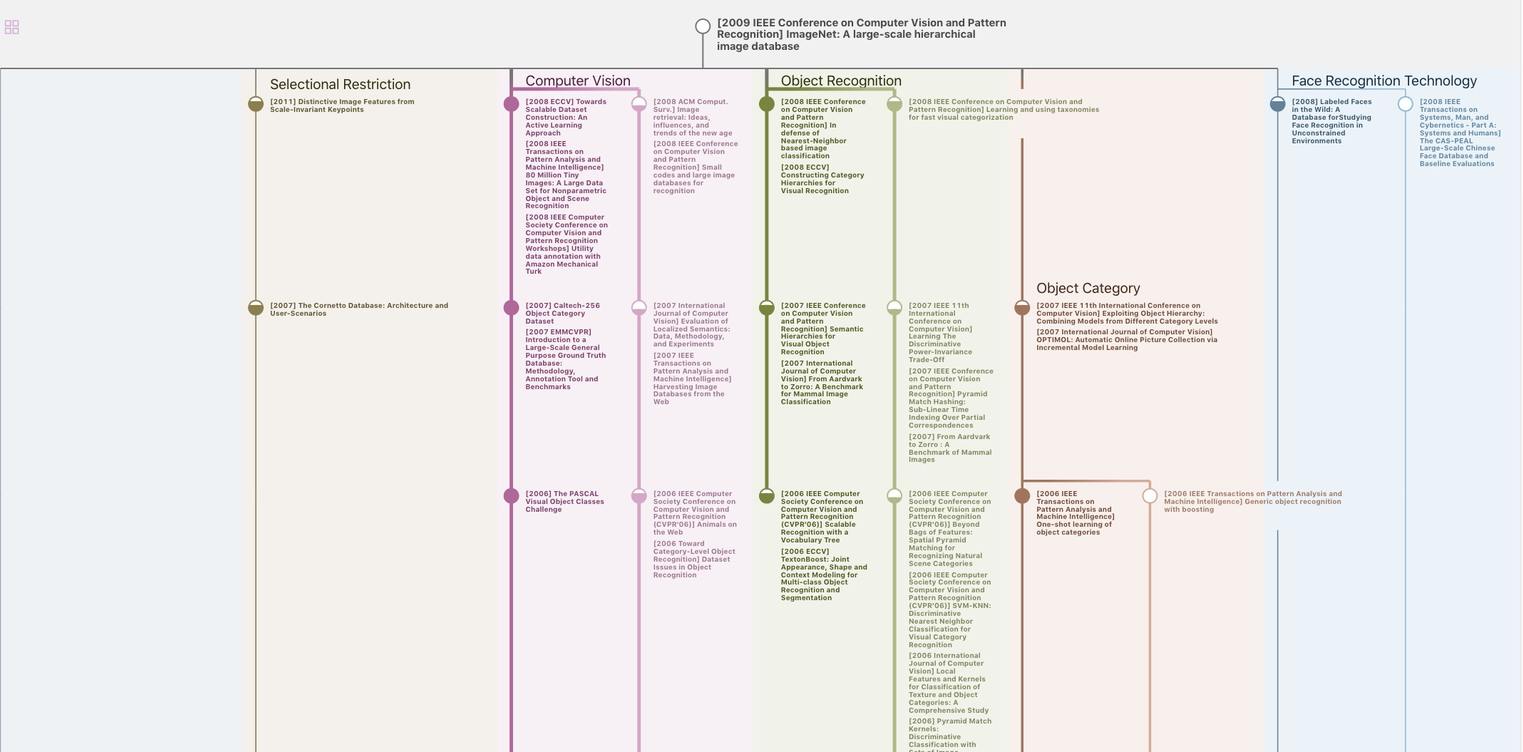
生成溯源树,研究论文发展脉络
Chat Paper
正在生成论文摘要