Enhancing Topic Interpretability for Neural Topic Modeling Through Topic-Wise Contrastive Learning.
IEEE International Conference on Data Engineering(2024)
摘要
Data mining and knowledge discovery are essential aspects of extracting valuable insights from vast datasets. Neural topic models (NTMs) have emerged as a valuable unsupervised tool in this field. However, the predominant objective in NTMs, which aims to discover topics maximizing data likelihood, often lacks alignment with the central goals of data mining and knowledge discovery which is to reveal interpretable insights from large data repositories. Overemphasizing likelihood maximization without incorporating topic regularization can lead to an overly expansive latent space for topic modeling. In this paper, we present an innovative approach to NTMs that addresses this misalignment by introducing contrastive learning measures to assess topic interpretability. We propose a novel NTM framework, named ContraTopic, that integrates a differentiable regularizer capable of evaluating multiple facets of topic interpretability throughout the training process. Our regularizer adopts a unique topic-wise contrastive methodology, fostering both internal coherence within topics and clear external distinctions among them. Comprehensive experiments conducted on three diverse datasets demonstrate that our approach consistently produces topics with superior interpretability compared to state-of-the-art NTMs.
更多查看译文
关键词
Data mining,Topic modeling,Contrastive learning
AI 理解论文
溯源树
样例
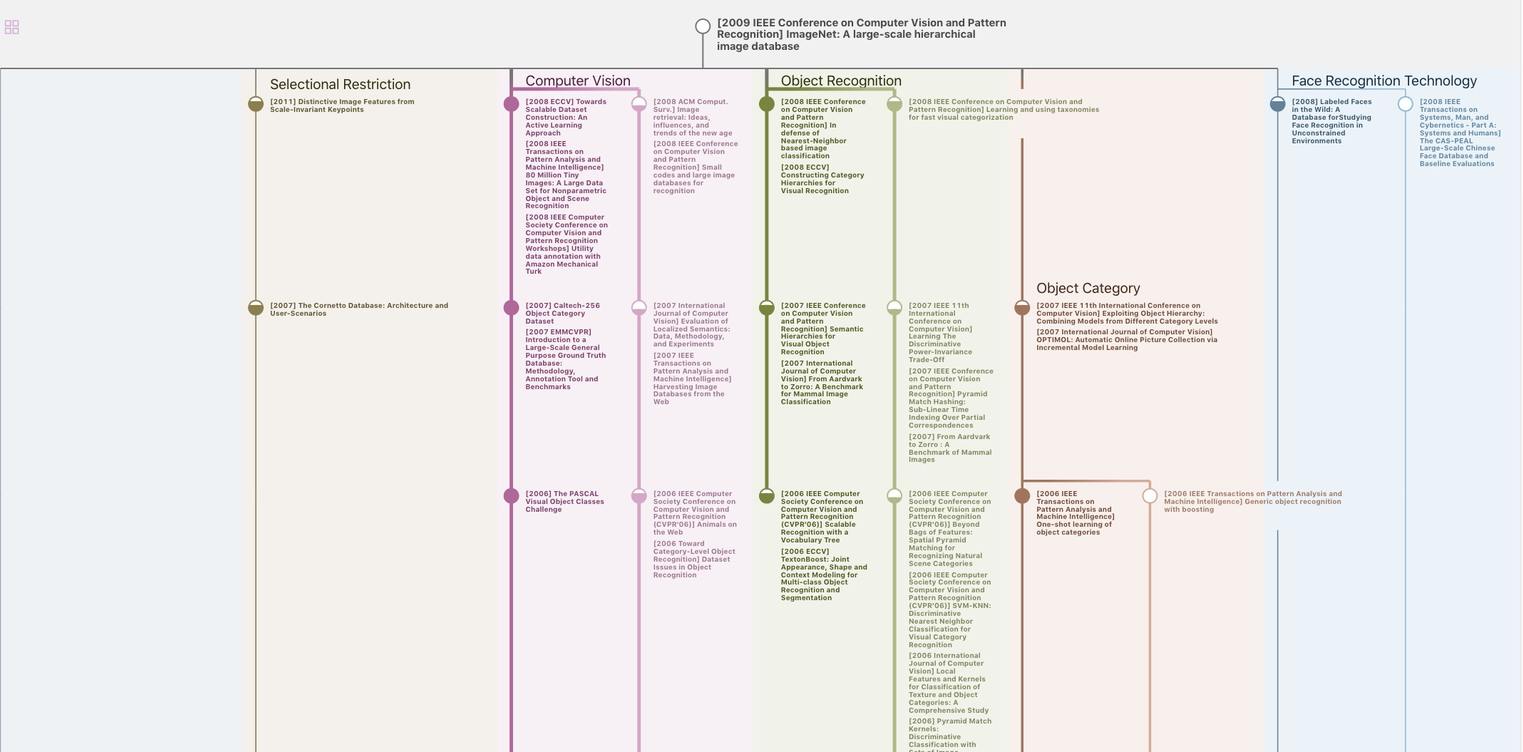
生成溯源树,研究论文发展脉络
Chat Paper
正在生成论文摘要