Behavior sessions and time-aware for multi-target sequential recommendation
Applied Intelligence(2024)
摘要
The sequentiality of sequences plays a crucial role in modeling the dynamic evolution of the user’s interests. Sequential recommendation models have significantly improved with the introduction of neural networks, offering users more personalized experiences. However, most models rely on a single type of behavior data and perform single-class target optimization on that type, overlooking the Click-Favorite-Purchase process that precedes a user’s final interaction with the item, and different behaviors in this process will have a significant impact on users’ interest. In this paper, we propose behavior sessions and time-aware for multi-targets sequential recommendation model (BTMT), which captures users’ interest changes from various behavior information. BTMT learns the influence factors of different behavior sessions as weights for each behavior. These weights introduce behavior information into a temporal attention network to dynamically model user’s interests in conjunction with time information. Furthermore, we distinguish the prediction of different users’ behaviors and perform multi-target joint optimization. Extensive experiments on four datasets demonstrate that BTMT’s prediction performance for each behavior target significantly outperforms various sequence models. These results validate the effectiveness of distinguishing behavior information in improving recommendation performance.
更多查看译文
关键词
Sequential recommendation,Behavior sessions,Multi-target prediction,Absolute time relationship
AI 理解论文
溯源树
样例
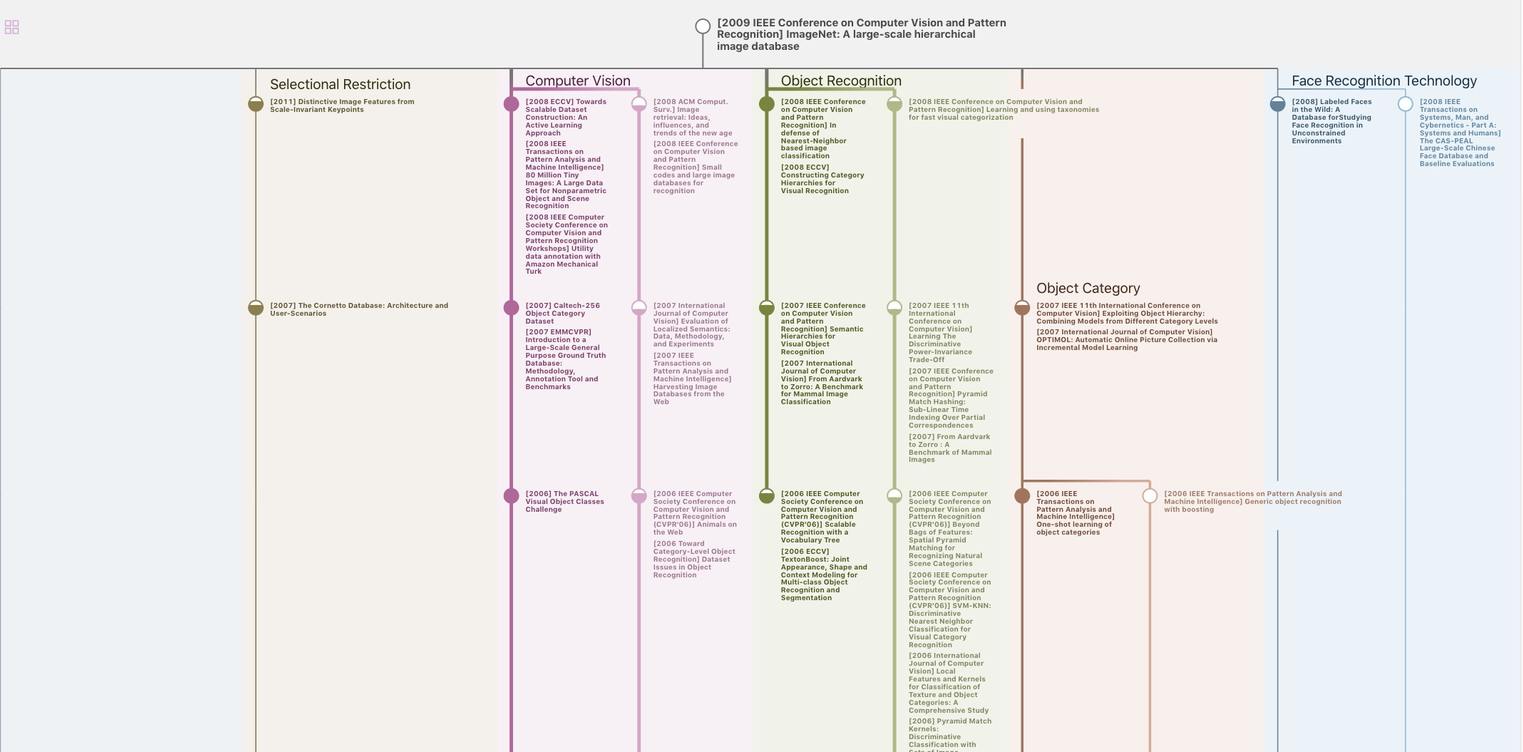
生成溯源树,研究论文发展脉络
Chat Paper
正在生成论文摘要