CCBA-NMS-YD: A Vehicle Pedestrian Detection and Tracking Method Based on Improved YOLOv7 and DeepSort
World Electric Vehicle Journal(2024)
摘要
In this paper, we propose a vehicle pedestrian detection and tracking method based on the improved YOLOv7 and DeepSort algorithms. We aim to improve the quality of vehicle pedestrian detection and tracking, addressing the challenges that current commercially available autonomous driving technologies face in complex and changing road traffic situations. First, the NMS (non-maximum suppression) algorithm in YOLOv7 is replaced with a modified Soft-NMS algorithm to ensure that targets can be accurately detected at high densities, and second, the CCBA (coordinate channel attention module) attention mechanism is incorporated to improve the feature extraction and perception capabilities of the network. Finally, a multi-scale feature network is introduced to extract features of small targets more accurately. Finally, the MobileNetV3 lightweight module is introduced into the feature extraction network of DeepSort, which not only reduces the number of model parameters and network complexity, but also improves the tracking performance of the target. The experimental results show that the improved YOLOv7 algorithm improves the average detection accuracy by 3.77% compared to that of the original algorithm; on the MOT20 dataset, the refined DeepSort model achieves a 1.6% increase in MOTA and a 1.9% improvement in MOTP; in addition, the model volume is one-eighth of the original algorithm. In summary, our model is able to achieve the desired real-time and accuracy, which is more suitable for autonomous driving.
更多查看译文
关键词
vehicle pedestrian,attention mechanism,non-maximum suppression,lightweight
AI 理解论文
溯源树
样例
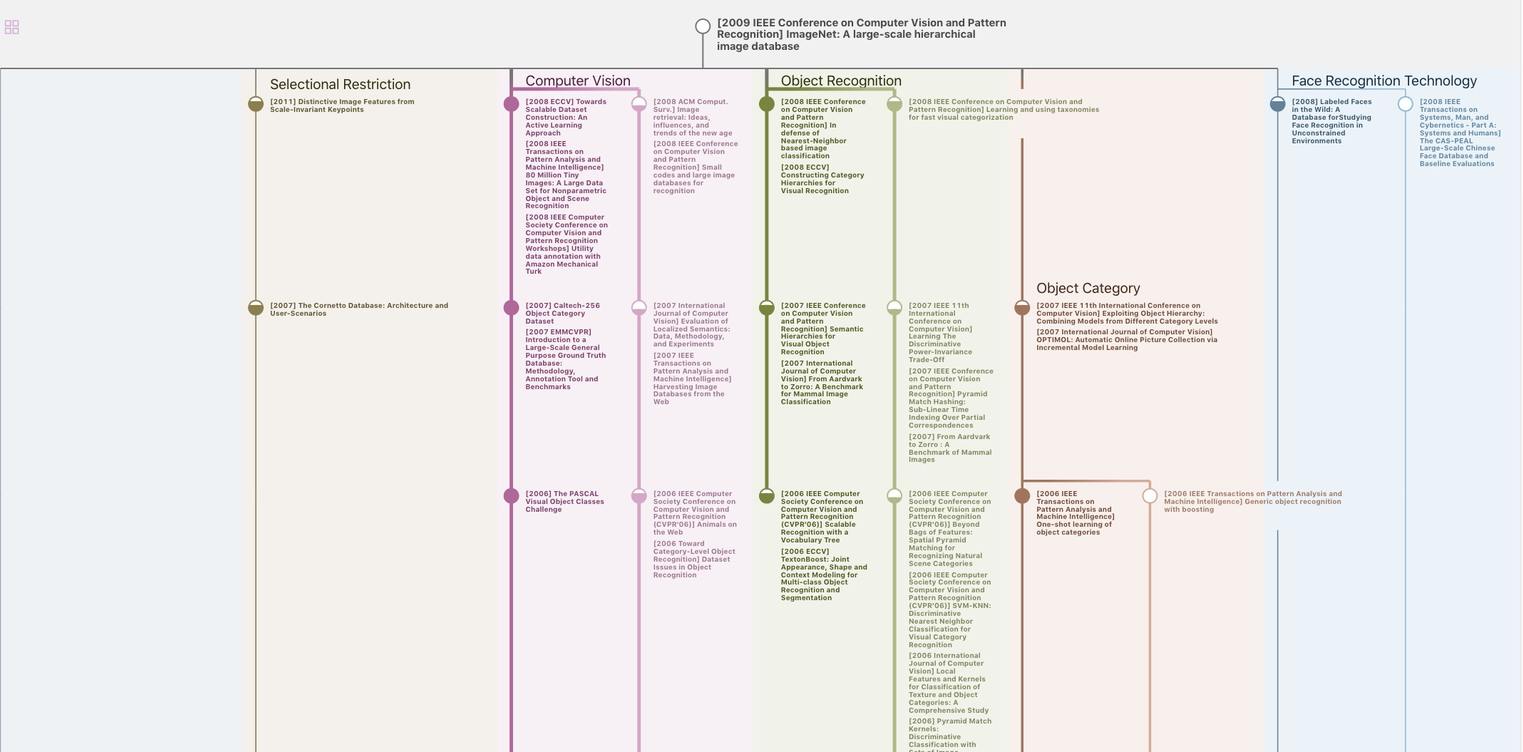
生成溯源树,研究论文发展脉络
Chat Paper
正在生成论文摘要