Benchmarking Current State-of-the-Art Transformer Models on Token Level Language Identification and Language Pair Identification
2023 International Conference on Computational Science and Computational Intelligence (CSCI)(2023)
摘要
With the rise of internet usage, code-switching, where multiple languages or dialects intermingle, has surged. Traditional linguistic analysis struggles with this mixed text, as they're typically monolingual-focused. This paper delves into two core tasks for analyzing code-switched data: Token Level Language Identification (LID) and our newly proposed Language Pair Identification (LPI). We benchmarked and compared current state-of-art transformer models across both tasks to gauge their applicability to the tasks. Our results showed that state-of-the-art multilingual transformer models could achieve state-of-the-art performance on both tasks. The impressive performance on LPI suggests that this will be the first step to utilizing Language Pair Identification to assist in various facets related to Code-Switched corpora and classification performance.
更多查看译文
关键词
Language identification,Token Level Analysis,Language Pair Recognition,BERT,Transformer
AI 理解论文
溯源树
样例
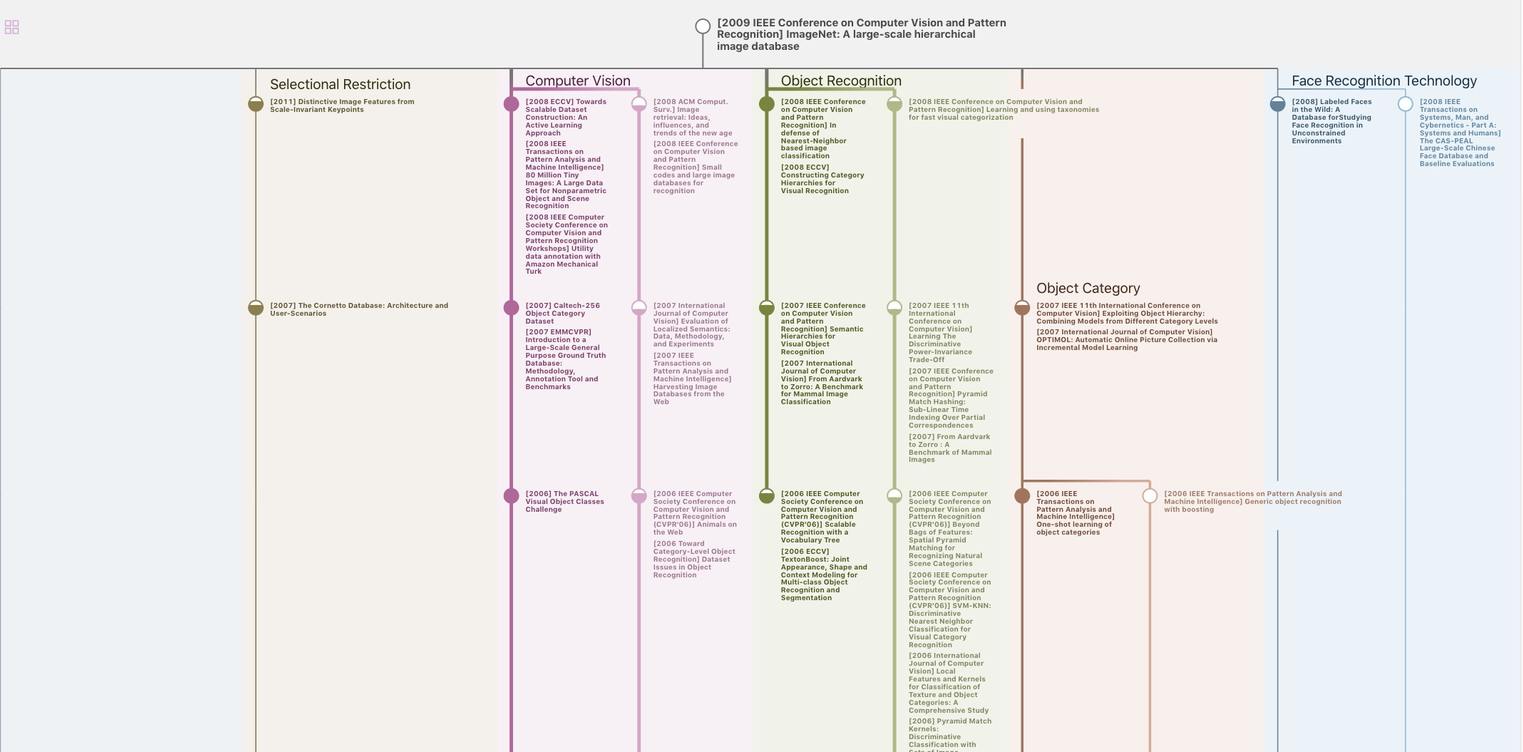
生成溯源树,研究论文发展脉络
Chat Paper
正在生成论文摘要