Resistive Processing Unit-based On-chip ANN Training with Digital Memory.
International Conference on Artificial Intelligence Circuits and Systems(2024)
Abstract
Artificial Neural Networks (ANNs) are popular for classification and regression tasks. Several in-memory computing architectures have been proposed to accelerate forward and backward passes in ANN training. However, the traditional ANN training operation (with backpropagation algorithm) is energy, area, and time-hungry due to separate and sequential computation units for the weight gradient calculation followed by weight update. A Resistive Processing Unit (RPU) architecture was explicitly proposed for the acceleration of weight gradient calculation and update for analog non-volatile memories. Despite valuable properties that enable RPU, the analog non-volatile memories suffer from issues like drift, non-linearity, asymmetry, variability, and high write energy, causing an increase in the array peripherals’ cost and accuracy degradation. In this work, we propose an adaptation of RPU to SRAM-based multi-bit weights for the ANN training acceleration. A simple combinational weight update control logic is proposed to facilitate the weight update. The proposed architecture shows an improvement in the linearity and symmetry for weight update, which further improves the training accuracy of the system.
MoreTranslated text
Key words
Artificial neural network (ANN),resistive processing unit (RPU),in-memory computation (IMC),static random access memory (SRAM),stochastic weight update
AI Read Science
Must-Reading Tree
Example
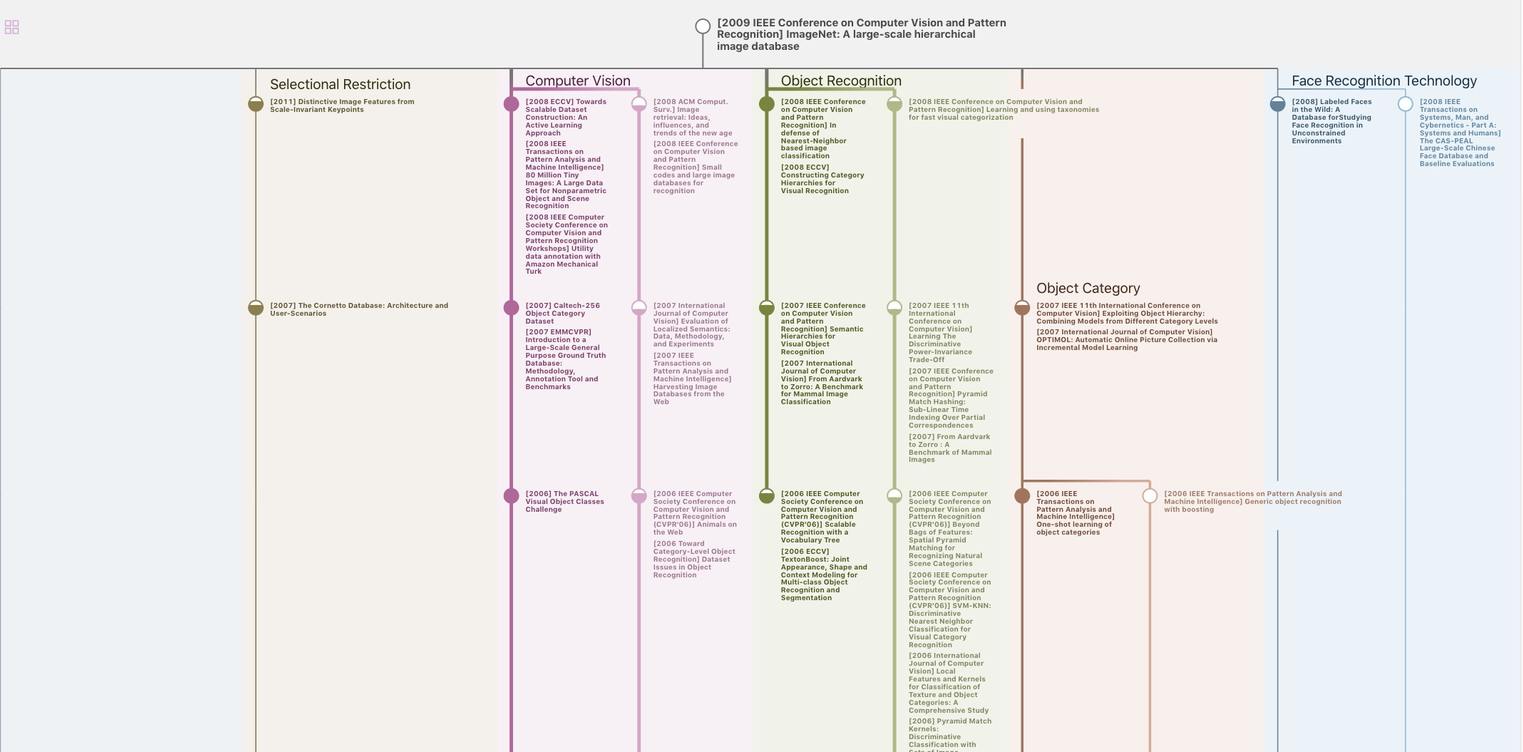
Generate MRT to find the research sequence of this paper
Chat Paper
Summary is being generated by the instructions you defined