Physics-Informed Machine Learning for Tribological Properties Prediction of S32750/CFRPEEK Tribopair under Seawater Lubrication Via PISSA-CNN-LSTM
Tribology International(2024)
摘要
Tribological properties and wear mechanisms of S32750 stainless steel/carbon fiber-reinforced polyetheretherketone (CFRPEEK) tribopair in seawater under different salinities, sliding speeds and loads were experimentally studied. A novel Physics-Informed constraint strategy has been originally developed and combined with sparrow search algorithm (SSA) to optimize a Convolutional Neural Network-Long Short-Term Memory (CNN-LSTM) model for predicting friction coefficient. The results indicate that an increase in seawater salinity and sliding speed enhances the tribological performance. Under high load, an increase in the PEEK transfer film leads to a slight decrease in friction coefficient. The Physics-Informed SSA (PISSA) offers enhanced performance through faster convergence, higher solution quality, and less computation time. Additionally, CNN-LSTM model optimized by PISSA (PISSA-CNN-LSTM) provides more accurate predictions of friction coefficient.
更多查看译文
关键词
Seawater lubrication,Friction coefficient prediction,Physics-Informed,PISSA-CNN-LSTM
AI 理解论文
溯源树
样例
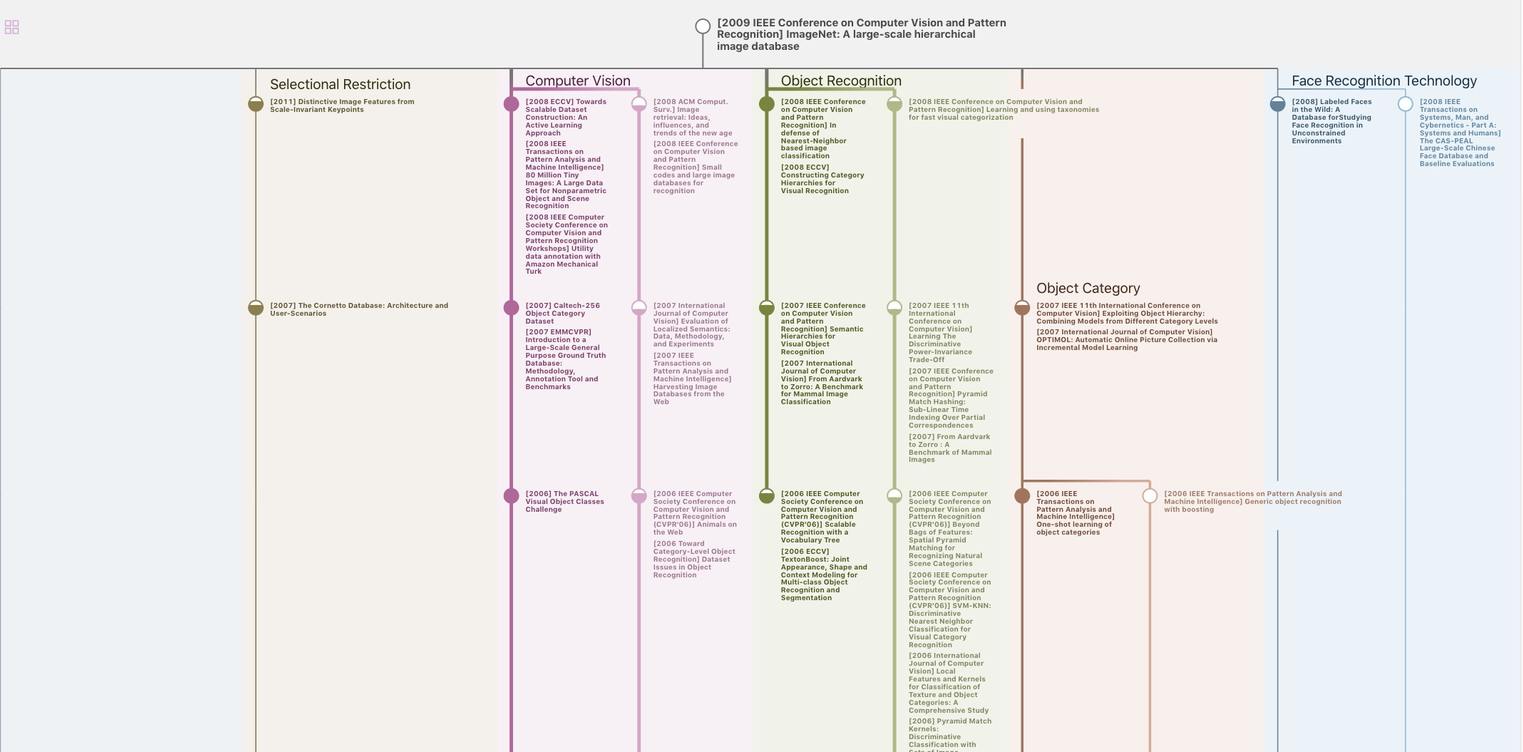
生成溯源树,研究论文发展脉络
Chat Paper
正在生成论文摘要