A two-stage CFD-GNN approach for efficient steady-state prediction of urban airflow and airborne contaminant dispersion
Sustainable Cities and Society(2024)
摘要
Urban design requires accurate prediction of wind, gusts, and contaminant dispersion to create sustainable cities. While computational fluid dynamics (CFD) models like large-eddy simulation (LES) provide accurate predictions, they are computationally expensive. Steady Reynolds-averaged Navier-Stokes (SRANS) is fast but inaccurate due to inherent limitations. This study proposes a two-stage CFD-graph neural network (GNN) framework for efficient steady-state prediction. A CFD solver provides the initial state, and then a GNN directly corrects the CFD outputs towards a high-fidelity target in a single inference step, bypassing SRANS flaws. LES results for parametrically generated urban cases provided training data. The proposed framework is thus distinguished from previous GNN applications, which were unsteady RANS surrogates that are still constrained by RANS inaccuracy. SRANS with the k-ε model provided an informative initial state for GNN (termed KE-GNN). Less detailed initializations increased prediction difficulty. Uneven sampling of building density and heights introduced dataset bias, improving specialization but reducing generalizability. For an actual urban case, KE-GNN employing a coarse mesh achieved considerable agreement with the fine LES result and was thousands of times faster. On the same coarse mesh, KE-GNN was seven times faster than a tightly converged SRANS and 280 times faster than an under-resolved LES.
更多查看译文
关键词
Graph neural network,Computational fluid dynamics,Urban airflow,Pollutant dispersion
AI 理解论文
溯源树
样例
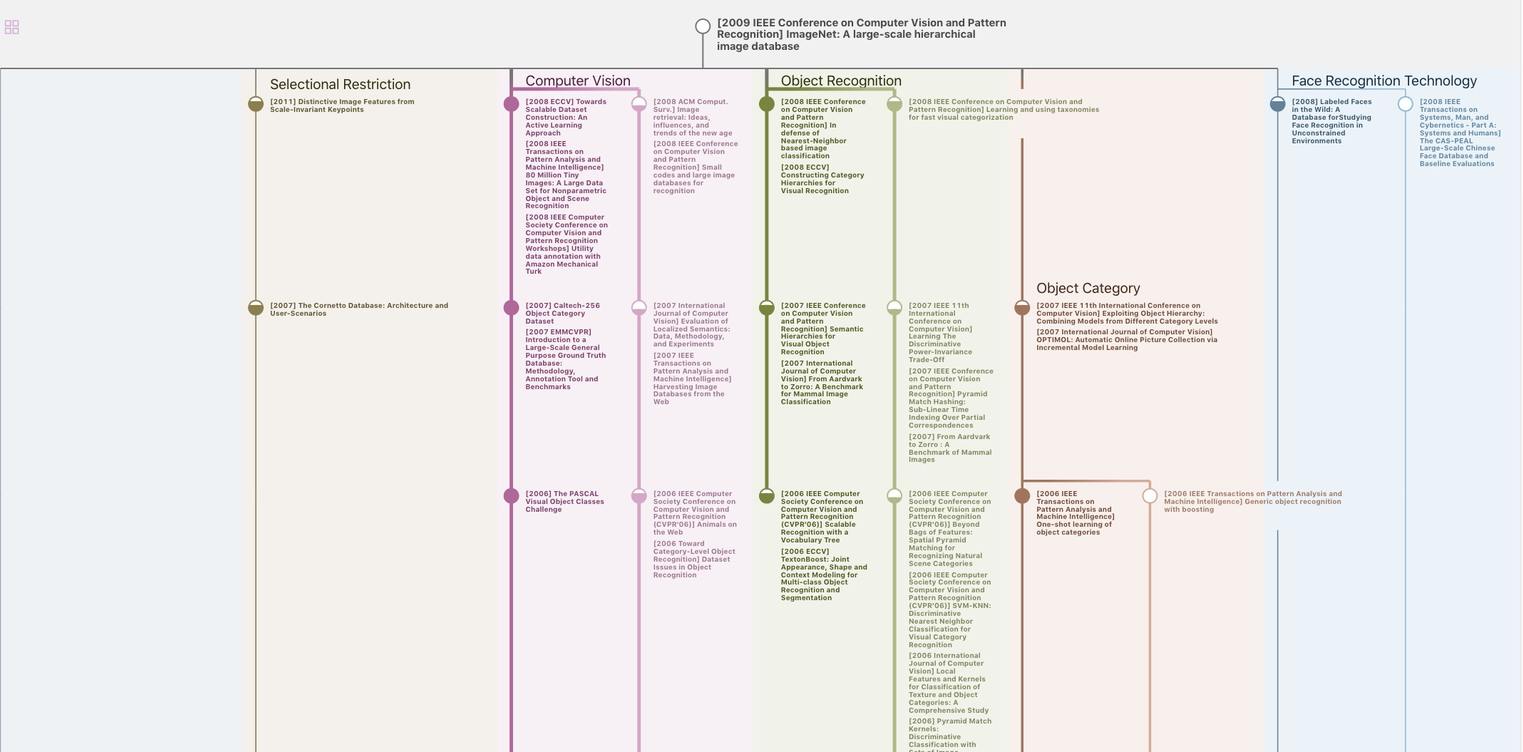
生成溯源树,研究论文发展脉络
Chat Paper
正在生成论文摘要