The effect of artificial intelligence evolving on hyperspectral imagery with different signal-to-noise ratio, spectral and spatial resolutions
Remote Sensing of Environment(2024)
摘要
Hyperspectral images are increasingly being used in classification and identification. Data users prefer hyperspectral imagery with high spatial resolution, finer spectral resolution, and high signal-to-noise ratio (SNR). However, tradeoffs exist in these core parameters in imagery acquired by different hyperspectral sensor systems. Data users may find it difficult to utilize all the advantages of hyperspectral imagery. How to select hyperspectral data with optimal parameter configuration has been one of the essential issues for data users, which also affects the back-end applications. With advancements in computer science, various artificial intelligence algorithms from conventional machine learning to deep learning have been utilized for hyperspectral images classification and identification. Few researchers study the mechanism between the core parameters of hyperspectral imaging spectrometers and advanced artificial intelligence algorithms, which affects the application efficiency and accuracy. In this paper, we delved into the evolution of machine learning and deep learning models applied to imagery acquired by different hyperspectral sensor systems having different SNR, spectral, and spatial resolutions. Additionally, we also considered the tradeoffs among the core parameters of hyperspectral imagers. We used two conventional machine learning models, including the classification and regression tree (CART) and random forest (RF), two deep learning methods based on convolution neural network architectures—3D convolutional neural network (3D-CNN) and hamida, and two deep learning methods based on vision transformers architectures—transformer models vision transformer (VIT) and robust vision transformer (RVT), to compare the characteristics of different algorithms. In addition, five hyperspectral datasets with different species categories and scene distributions and aggregated datasets with different spatial resolutions, spectral resolutions, and SNRs were used to validate our study. The experimental results indicate that: (1) The overall accuracy (OA) using CART, RF, 3D-CNN, and VIT models decreased with coarser spectral resolution, but almost remained unchanged using the RVT classifier. The number of class and classification species affect the results. (2) The influence of spatial resolution on classification accuracy is related to the scene complexity, target size, and classification purpose. The coarser spatial resolution can achieve higher OA than the original spatial resolution for the uniform scene distribution. For the datasets with small objects and intersections of different species, OA first increased, plateaued, and then decreased with coarser spatial resolution. (3) The SNR has an obvious impact on OA for the CART and RF classifiers, and the impact decreased for deep learning models, especially for the VIT and RVT models, which were almost unaffected by SNR. Additionally, slight variations in experimental results were observed for datasets with different scene distributions and categories. Furthermore, we conducted a detailed analysis of the role of traditional machine learning and deep learning models in the experimental outcomes. The study can provide insights into understanding the relationship between the core parameters of hyperspectral imager and the artificial intelligence algorithms used for hyperspectral classification. It serves to bridge the knowledge gap between the front-end hyperspectral imager, mid-end model, and back-end applications, and further promote the development of hyperspectral imaging technology.
更多查看译文
关键词
Hyperspectral imaging spectrometer,Core parameters,Tradeoff,Hyperspectral classification,Artificial intelligence
AI 理解论文
溯源树
样例
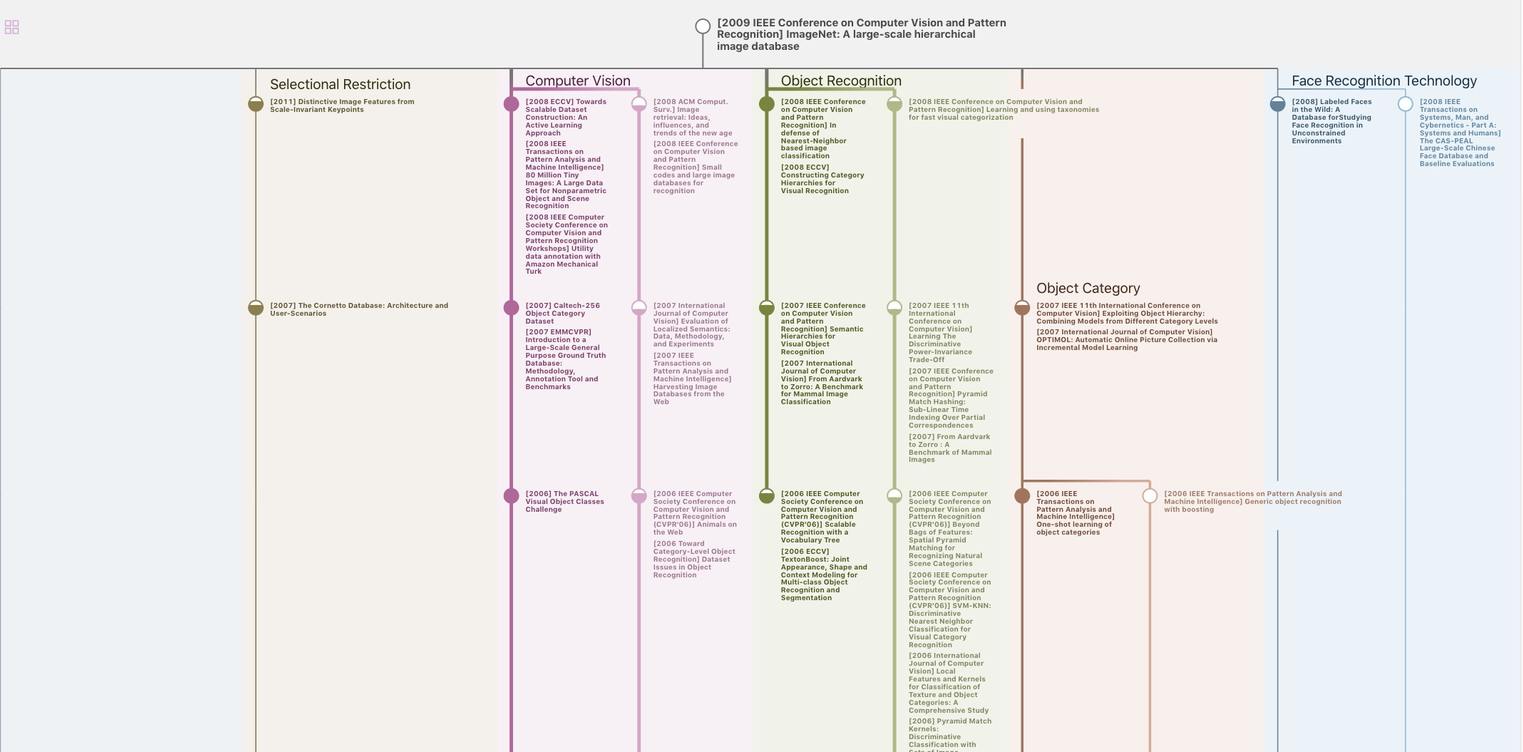
生成溯源树,研究论文发展脉络
Chat Paper
正在生成论文摘要