Motion-guided spatiotemporal multitask feature discrimination for self-supervised video representation learning
Pattern Recognition(2024)
摘要
Powerful self-supervised representation models are able to step out of the traditional supervised paradigm and rely merely on unlabeled data to achieve a deep understanding of visual semantic features. However, previous approaches may suffer from background scene bias, making it difficult to perform a comprehensive exploration of video spatiotemporal structure. To address this challenge, this paper proposes a self-supervised video representation learning framework of motion-guided spatiotemporal multitask feature discrimination (MSMFD). The method mainly utilizes the consistency of motion cues between different views to guide the model for spatial and temporal feature similarity discrimination. Specifically, the model first selects video clips with large motion amplitudes based on the collected optical flow maps. Subsequently, the model introduces an instance discrimination task for overall spatiotemporal structure perception of the video, while a shuffled triplet and an augmented quadruple task are created to further enhance the exploration of intraframe sequence order and local spatial fine-grained. Furthermore, we propose joint motion alignment of spatial, temporal, and spatiotemporal dimensions under different views as a powerful compensation for acquiring motion features. Experimental results demonstrate that our self-supervised method is effective for learning video representations and achieves competitive performance in action recognition and video retrieval tasks compared to other state-of-the-art methods.
更多查看译文
关键词
Unsupervised learning,Self-supervised learning,Cross-view learning,Multitask discrimination,Video action understanding
AI 理解论文
溯源树
样例
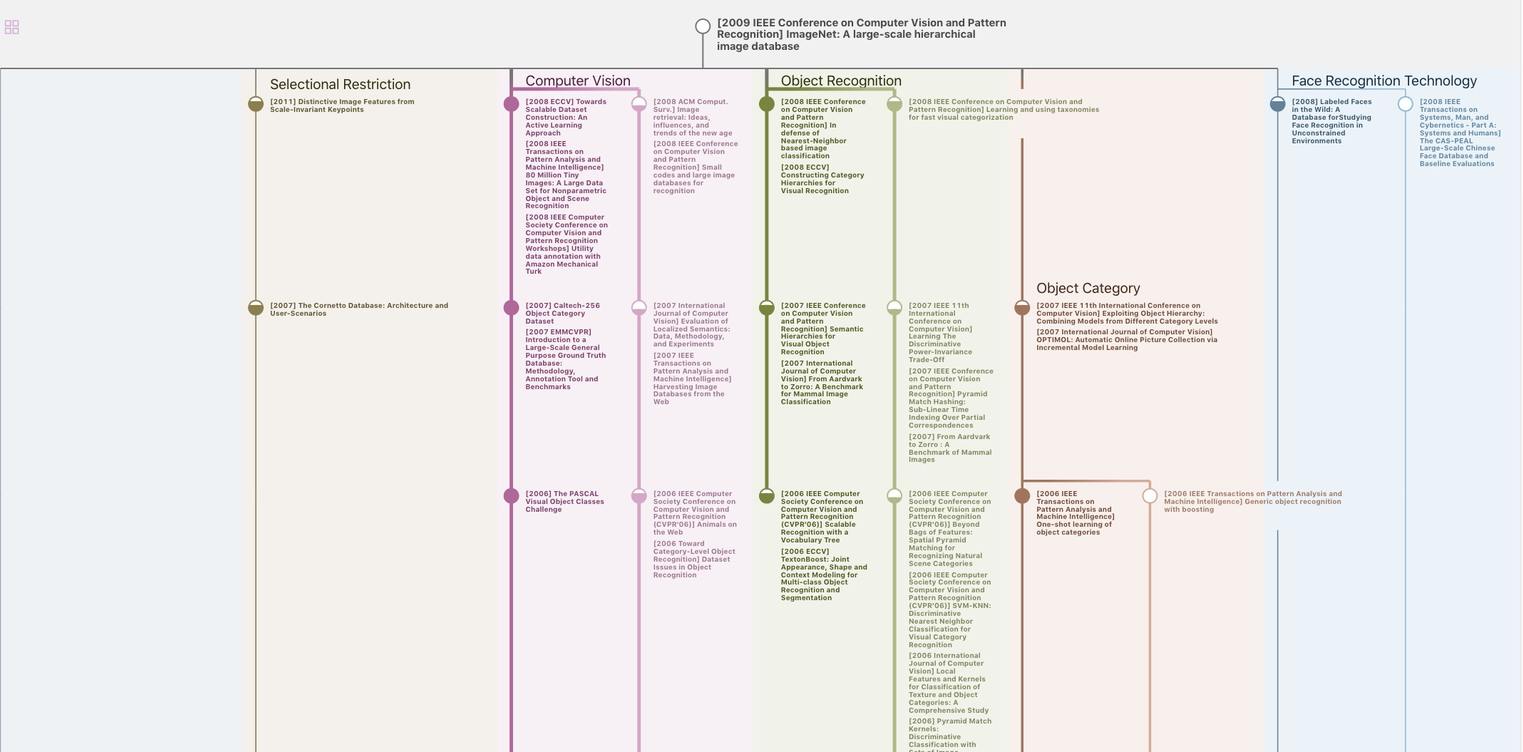
生成溯源树,研究论文发展脉络
Chat Paper
正在生成论文摘要