The importance of cycle-by-cycle data in performing rapid battery technology development and validation
Journal of Energy Storage(2024)
Abstract
Lithium-ion battery (LiB) technology is playing a crucial role in transforming the predominantly fossil fuel-based transportation and stationary storage sectors to achieve a low-carbon economy. Rapid innovation in the LiB materials to electrode to cell design is happening to satisfy the performance, life, and safety metrics required by those myriads of applications. Lately, advanced analytics, such as machine-learning or artificial intelligence (ML/AI) techniques, are being used more frequently to aid in expedited LiB technology development, performance validation, and life prediction. The success of these techniques often relies on a large volume of well-defined and high-quality battery test data. On the other hand, most battery developers and research and development (R&D) communities are still following a classical approach to develop batteries, which is running calendar- and/or cycle-aging tests, performing reference performance tests (RPTs), and conducting post-mortem analyses periodically without paying attention to the wealth of data often not collected during the calendar or cycle life aging tests. This sparse data collection approach is time- and resource-intensive, requiring data capture and evaluation of months to years of RPT data to diagnose accurate battery state of performance, health, and safety. Even so, the underlying aging modes and mechanisms can be missed. If collected properly, battery test data during cycling or calendaring can be efficiently combined with ML/AI techniques to create powerful tools in the rapid diagnosis of battery state of performance, health, and safety along with insights into underlying aging modes and mechanisms. In this article, we discuss the importance of effective cycle-by-cycle (CBC) data collection with example case studies. Within a reasonable timeframe, RPT data are often inadequate in capturing many of the crucial battery aging dynamics, which often predominantly show up in CBC test data. Finally, we also show examples of ML/AI techniques that use CBC data in rapid diagnosis and projection of LiB state of health (SOH) to motivate the scientific community in collecting and using CBC data to facilitate expeditious technology development and validation.
MoreTranslated text
Key words
Lithium-ion battery,Cycle-by-cycle data,Rapid operational validation,Machine-learning,Artificial intelligence
AI Read Science
Must-Reading Tree
Example
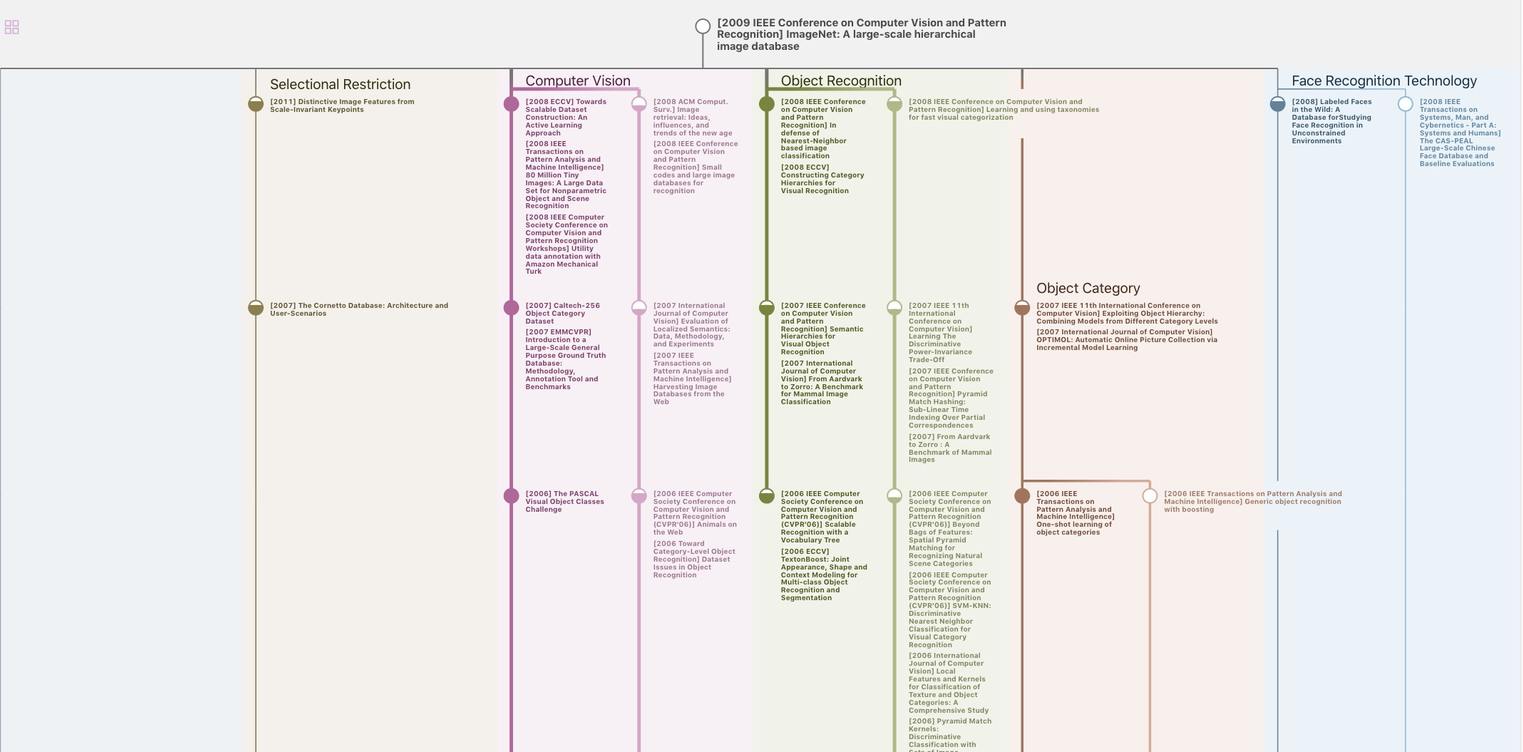
Generate MRT to find the research sequence of this paper
Chat Paper
Summary is being generated by the instructions you defined