Algorithm-driven estimation of household cooking activity and its impact on indoor PM2.5 assessments
Indoor Environments(2024)
摘要
Background
Household PM2.5 exposures have adverse health effects, and cooking behaviors are an important source of PM2.5 in the home. There is a need for accurate measures of cooking activity to better understand its associations with household PM2.5 since self-reported surveys are often subject to recall bias and misreporting of cooking events.
Objective
In this study, we aimed to address limitations associated with a self-reported cooking metric, by using temperature data to estimate cooking activity.
Methods
We developed an algorithm to identify cooking events at the 5-minute level using real-time temperature data measured near the stove and in the living room, across 148 households in Chelsea and Dorchester, MA. We compared the number of cooking events identified by this algorithm with cooking events self-reported by participants in daily activity logs and survey responses, and further assessed how these metrics differed with respect to their associations with occurrence of peak PM2.5, in mixed effects logistic regression models.
Results
We found that 65 % of the cooking events identified by the algorithm were not reported by participants. Furthermore, households classified as frequent vs infrequent cooking households using the algorithm had a larger difference in indoor PM2.5 levels, compared to households classified by self-report. In mixed effects logistic regression models for elevated household PM2.5 levels, we observed much stronger associations between household PM2.5 and algorithm-derived cooking activity (OR: 2.85 [95 % CI: 2.76, 2.95]) as compared to the association between household PM2.5 and self-reported cooking activity (OR: 1.22 [95 % CI: 1.17, 1.27] for stove use and OR: 1.67 [95 % CI: 1.58, 1.76] for grill use/frying/broiling/sauteing).
Significance
Overall, the algorithm developed in this study presents a data-driven approach to collecting cooking activity data in U.S. households, that may be more indicative of actual cooking events and also more predictive of household PM2.5 in indoor environmental models.
更多查看译文
关键词
Indoor air pollution,particulate matter,cooking activity,temperature algorithm,stove usage
AI 理解论文
溯源树
样例
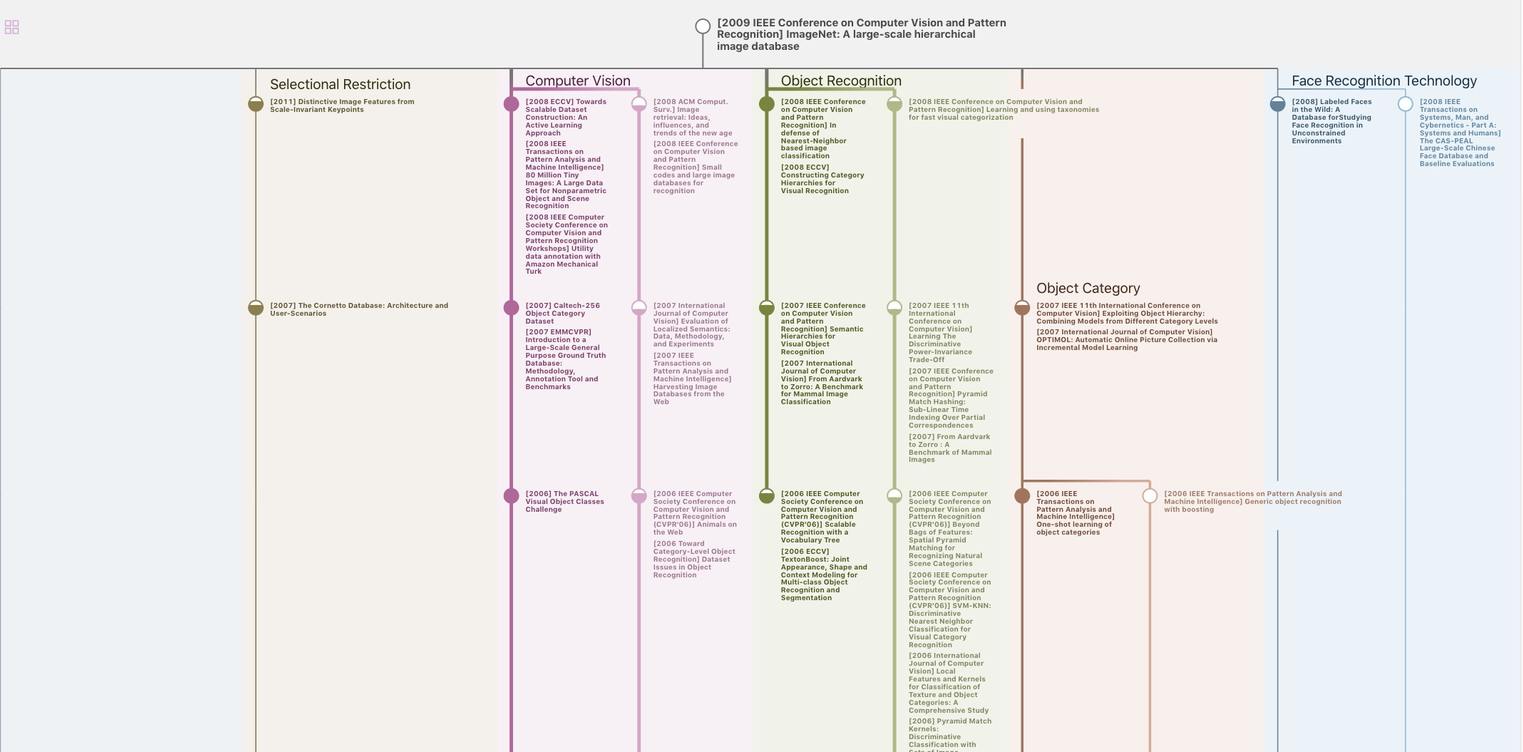
生成溯源树,研究论文发展脉络
Chat Paper
正在生成论文摘要