Reciprocal Federated Learning Framework: Balancing Incentives for Model and Data Owners
Future Generation Computer Systems(2024)
摘要
In the evolving landscape of Web 3.0, 5G/6G, and real-world applications, federated learning faces unique challenges. Traditional incentive mechanisms struggle to address the need to motivate both data owners to provide high-quality data and model experts to optimize model performance. To navigate this complex scenario, we introduce the Reciprocal Federated Learning Framework (RFLF). This innovative approach fosters a fair and dynamic reward structure that incentivizes both high-quality data contributions and optimal model development. Extensive experiments on benchmark datasets demonstrate that the RFLF significantly enhances fairness and efficiency within federated learning. These results showcase the RFLF’s potential to transform data-driven technologies, promoting both efficiency and equitable outcomes.
更多查看译文
关键词
Reciprocal,Federated learning,Incentive mechanism
AI 理解论文
溯源树
样例
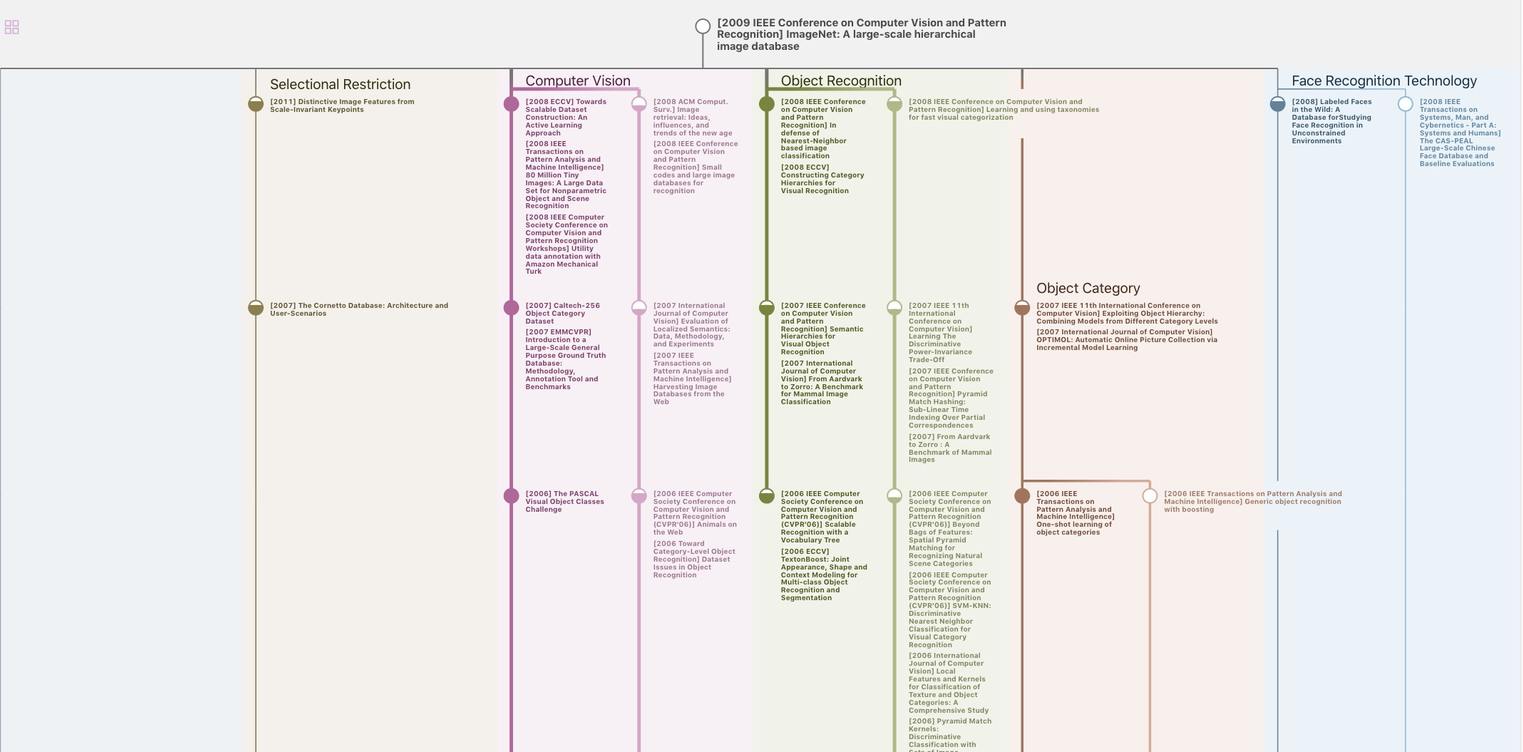
生成溯源树,研究论文发展脉络
Chat Paper
正在生成论文摘要