Machine learning based prediction models for uniaxial ratchetting of extruded AZ31 magnesium alloy
Extreme Mechanics Letters(2024)
Abstract
The detrimental effect of ratchetting on the fatigue life of materials requires precise prediction models to guarantee the safety of engineering structures. This study focuses on predicting the uniaxial ratchetting of extruded AZ31 magnesium (Mg) alloy using machine learning (ML) based approaches. At first, the evolution and deformation mechanisms of ratchetting are summarized based on the existing experimental results of the Mg alloy. Subsequently, a semi-empirical prediction model, tailored for engineering applications, is developed to describe the evolution of ratchetting strain. Then, a pure data-driven ML based prediction model is proposed to overcome the shortcoming existed in the semi-empirical model and improve the prediction accuracy to the uniaxial ratchetting of the Mg alloy. Finally, a physics-informed ML based model, incorporating the physical information derived from the semi-empirical one, is proposed to further enhance its prediction accuracy and generalization ability. The comparison with correspondent experimental data demonstrates that the proposed physics-informed ML based model exhibits high prediction accuracy and generalization ability.
MoreTranslated text
Key words
Extruded Mg alloy,Ratchetting,Deformation mechanism,Machine learning,Physics-informed machine learning
AI Read Science
Must-Reading Tree
Example
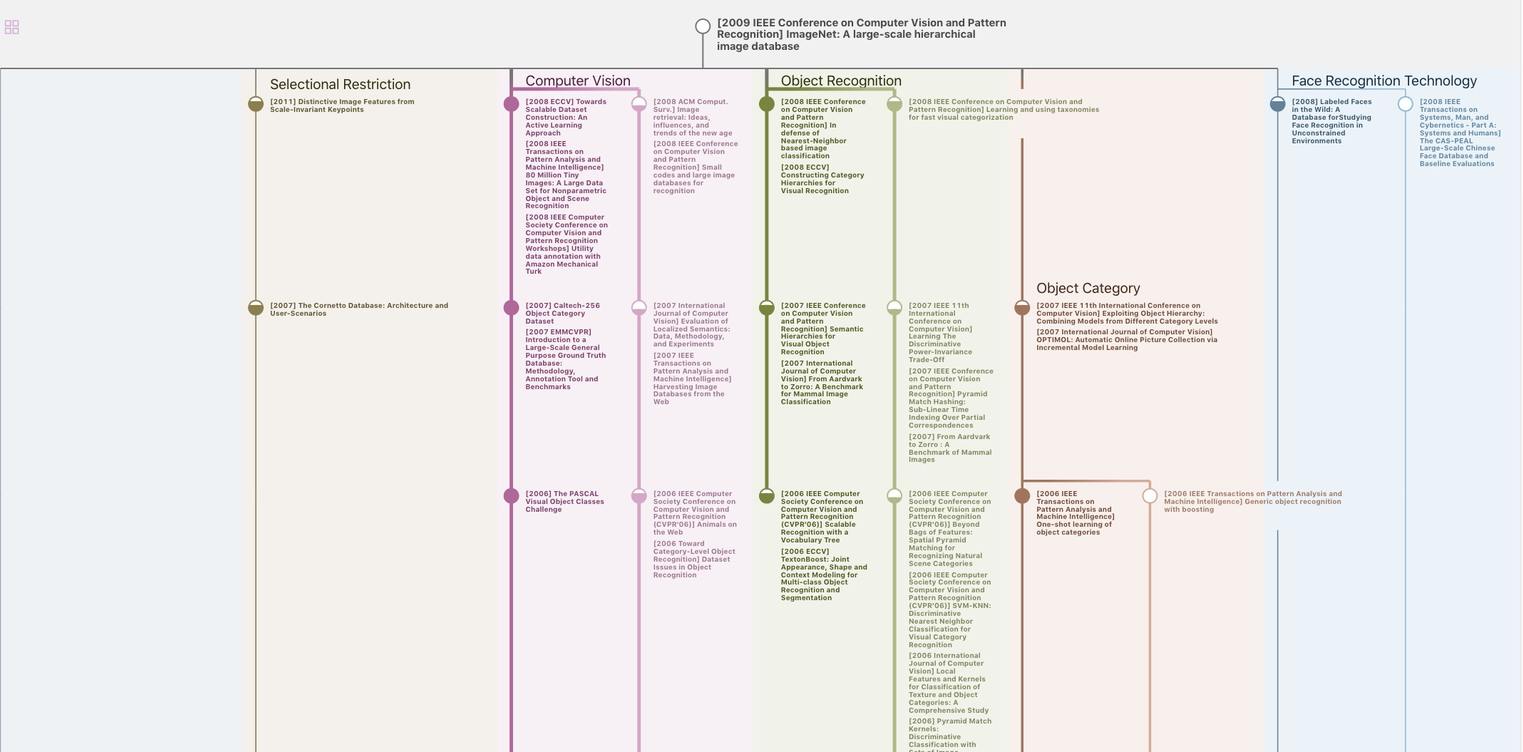
Generate MRT to find the research sequence of this paper
Chat Paper
Summary is being generated by the instructions you defined