Transferring Adult-like Phase Images for Robust Multi-view Isointense Infant Brain Segmentation
IEEE Transactions on Medical Imaging(2024)
摘要
Accurate tissue segmentation of infant brain in magnetic resonance (MR) images is crucial for charting early brain development and identifying biomarkers. Due to ongoing myelination and maturation, in the isointense phase (6-9 months of age), the gray and white matters of infant brain exhibit similar intensity levels in MR images, posing significant challenges for tissue segmentation. Meanwhile, in the adult-like phase around 12 months of age, the MR images show high tissue contrast and can be easily segmented. In this paper, we propose to effectively exploit adult-like phase images to achieve robustmulti-view isointense infant brain segmentation. Specifically, in one way, we transfer adult-like phase images to the isointense view, which have similar tissue contrast as the isointense phase images, and use the transferred images to train an isointense-view segmentation network. On the other way, we transfer isointense phase images to the adult-like view, which have enhanced tissue contrast, for training a segmentation network in the adult-like view. The segmentation networks of different views form a multi-path architecture that performs multi-view learning to further boost the segmentation performance. Since anatomy-preserving style transfer is key to the downstream segmentation task, we develop a Disentangled Cycle-consistent Adversarial Network (DCAN) with strong regularization terms to accurately transfer realistic tissue contrast between isointense and adult-like phase images while still maintaining their structural consistency. Experiments on both NDAR and iSeg-2019 datasets demonstrate a significant superior performance of our method over the state-of-the-art methods.
更多查看译文
关键词
Isointense infant brain segmentation,adult-like phase images,multi-view learning,disentangled cycle-consistent adversarial network
AI 理解论文
溯源树
样例
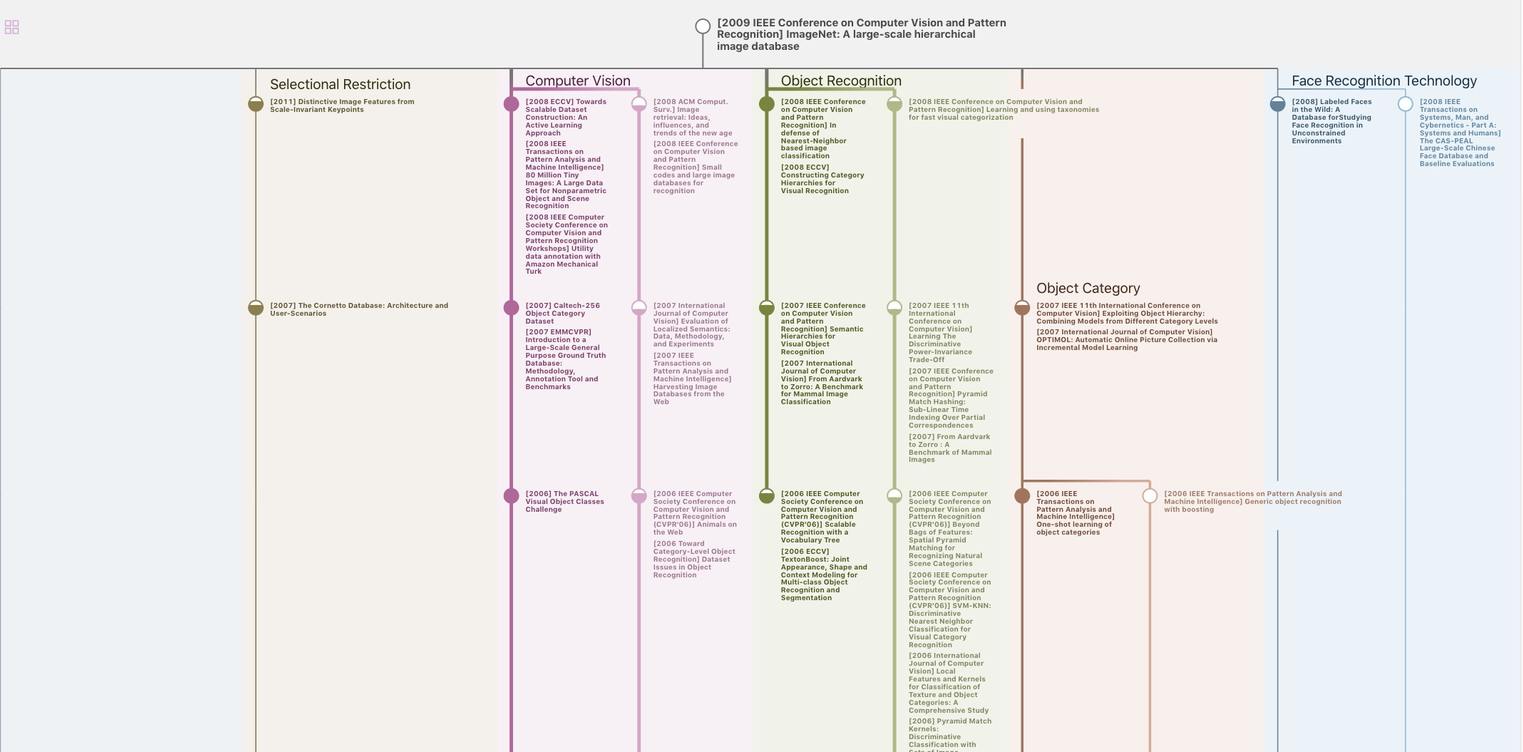
生成溯源树,研究论文发展脉络
Chat Paper
正在生成论文摘要