MRAU-net: Multi-scale residual attention U-shaped network for medical image segmentation
Computers and Electrical Engineering(2024)
Abstract
Medical image segmentation is a pivotal technology that assists doctors in making correct diagnoses and provides reliable evidence for pathological research. To address the insufficient attention to medical image details and the weak generalization ability of convolutional neural networks, we propose a novel multi-scale residual attention U-shaped network (MRAU-Net) for medical image segmentation. MRAU-Net employs a channel-wise compression strategy to construct the squeezed double convolution in the encoder, significantly reducing the network parameters while maintaining segmentation performance. In the decoder, a multi-scale residual attention block is designed to learn rich context information with multiple receptive fields, which effectively focuses on the target area and compensates for the lost information during the upsampling process through skip connections, enhancing the network's feature extraction capability. Experimental results on four biomedical image datasets show that our MRAU-Net performs better than the existing methods in terms of segmentation accuracy and generalization ability. mDices of MRAU-Net on JSUAHCerebellum, Kvasir-SEG, ISIC 2018 Challenge, and 2018 Data Science Bowl are 92.81 %, 88.64 %, 91.88 %, and 93.14 %, respectively, which are 7.61 %, 7.48 %, 6.34 %, and 2.34 % higher than that of the original U-Net.
MoreTranslated text
Key words
Medical image segmentation,Multi-scale residual attention,U-shaped network
AI Read Science
Must-Reading Tree
Example
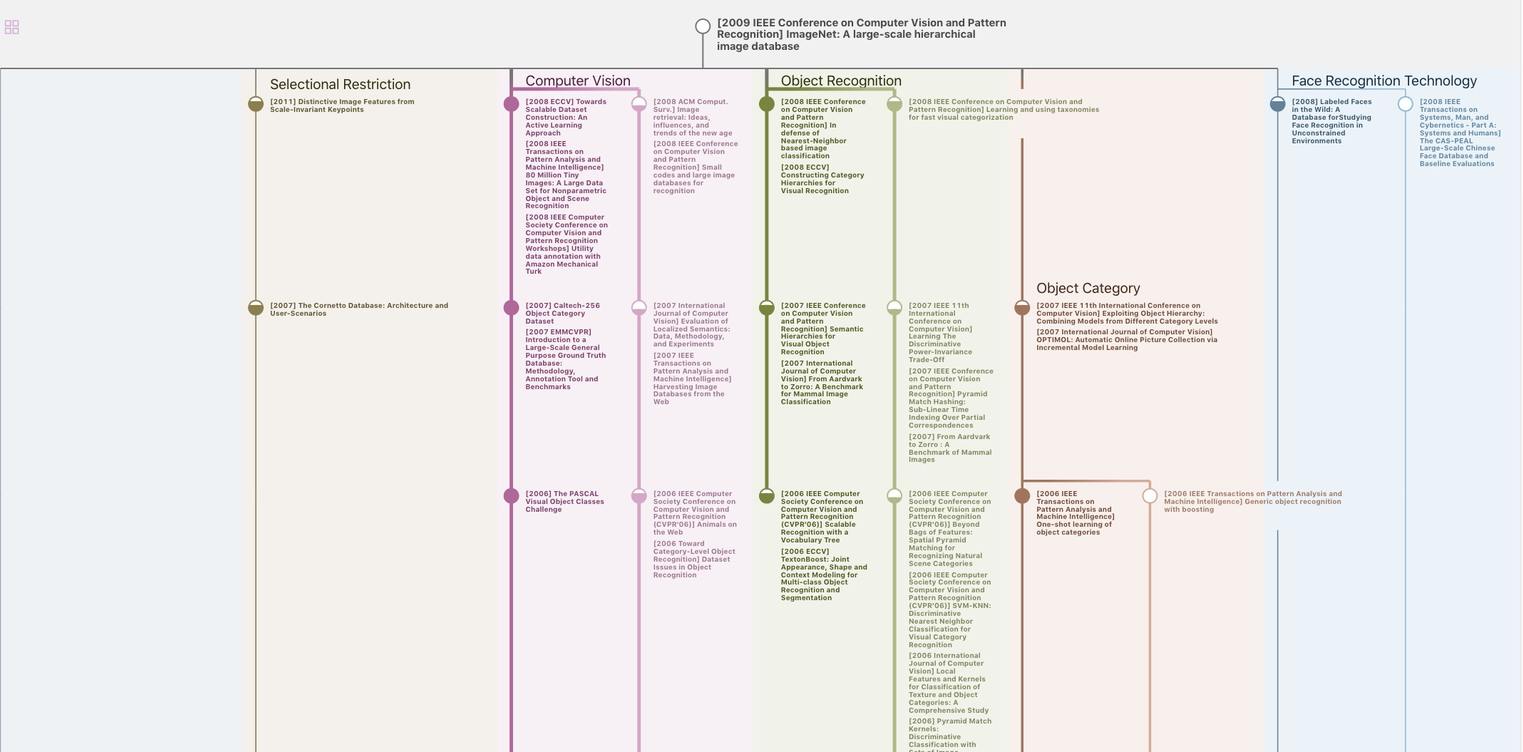
Generate MRT to find the research sequence of this paper
Chat Paper
Summary is being generated by the instructions you defined