Misspecified Q-Learning with Sparse Linear Function Approximation: Tight Bounds on Approximation Error
arxiv(2024)
摘要
The recent work by Dong Yang (2023) showed for misspecified sparse linear
bandits, one can obtain an O(ϵ)-optimal policy using a
polynomial number of samples when the sparsity is a constant, where ϵ
is the misspecification error. This result is in sharp contrast to misspecified
linear bandits without sparsity, which require an exponential number of samples
to get the same guarantee. In order to study whether the analog result is
possible in the reinforcement learning setting, we consider the following
problem: assuming the optimal Q-function is a d-dimensional linear function
with sparsity k and misspecification error ϵ, whether we can obtain
an O(ϵ)-optimal policy using number of samples polynomially
in the feature dimension d. We first demonstrate why the standard approach
based on Bellman backup or the existing optimistic value function elimination
approach such as OLIVE (Jiang et al., 2017) achieves suboptimal guarantees for
this problem. We then design a novel elimination-based algorithm to show one
can obtain an O(Hϵ)-optimal policy with sample complexity
polynomially in the feature dimension d and planning horizon H. Lastly, we
complement our upper bound with an Ω(Hϵ)
suboptimality lower bound, giving a complete picture of this problem.
更多查看译文
AI 理解论文
溯源树
样例
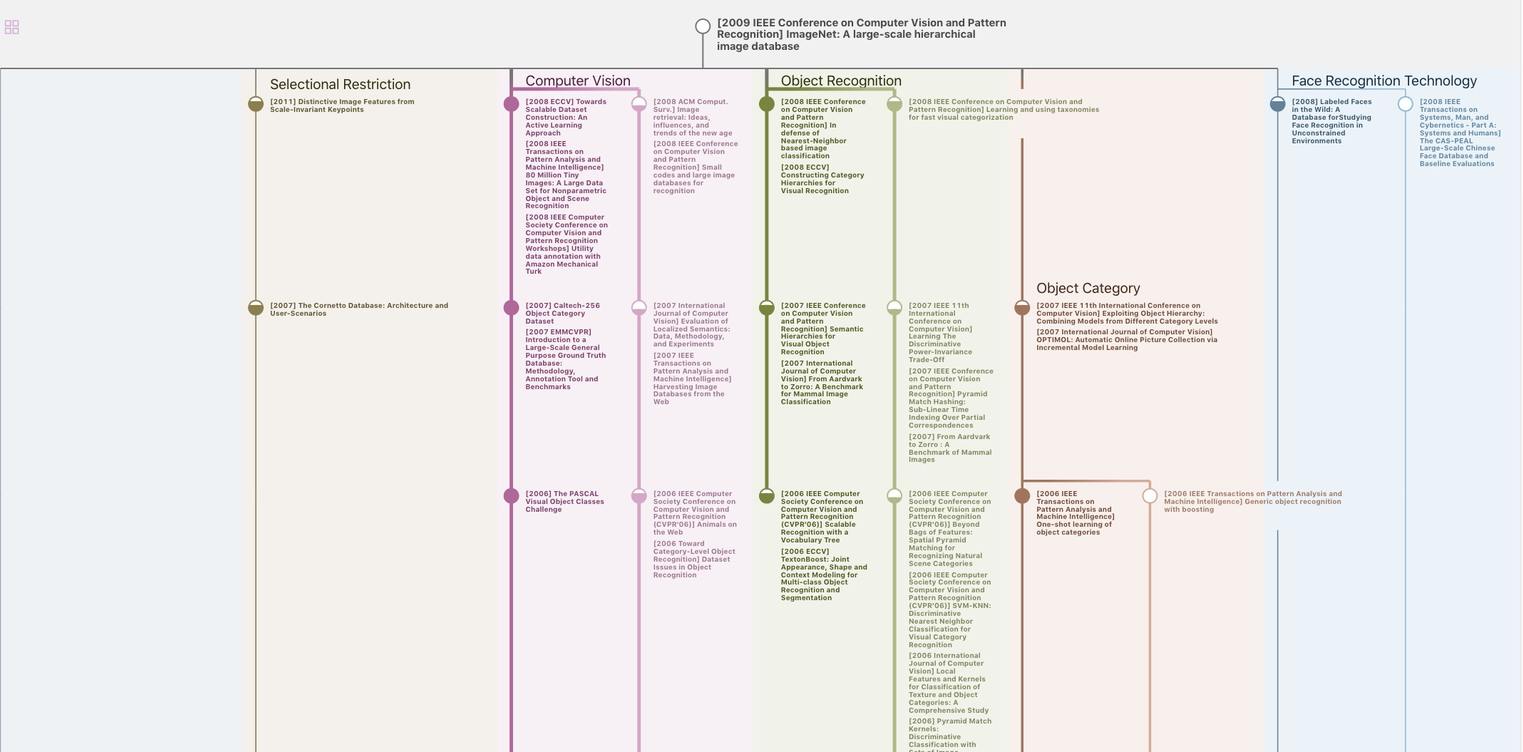
生成溯源树,研究论文发展脉络
Chat Paper
正在生成论文摘要