Reinforcement Learning-based anti-Jamming Solution for Aerial RIS-aided Dense Dynamic Multi-User Environments
2024 International Wireless Communications and Mobile Computing (IWCMC)(2024)
摘要
In the 5G Advanced and 6G era, wireless communication systems face security challenges, notably adversarial interference from unknown jammers in multi-user scenarios. Reconfigurable Intelligent Surfaces (RIS) present a cost-effective solution due to their low power consumption and easy deployment. Existing RIS techniques typically address simple jamming scenarios with a single static jammer, focusing on a single objective. This study introduces a multi-objective optimization approach deploying UAV-mounted RIS to counter jamming threats in wireless communications within a densely populated smart city environment. The proposed solution aims to safeguard essential services from potential disruptions caused by malicious jamming attacks during public events. We employ Proximal Policy Optimization (PPO), a lightweight Deep Reinforcement Learning (DRL) technique, to concurrently optimize the trajectory of UAV and RIS passive beamforming to address computational complexity. The objectives include maximizing the average sum rate and minimizing energy consumption. Our experiments highlight the efficacy of the PPO-based strategy, demonstrating significant improvements in average sum rates and energy efficiency amid numerous mobile devices and moving jammers. Importantly, our proposed system model outperforms a baseline from related works in maximizing the sum rate and minimizing overall energy consumption.
更多查看译文
关键词
Wireless Communications,Reinforcement Learning,Proximal Policy Optimization,PPO,Jamming,Anti-Jamming,Reconfigurable Intelligent Surfaces,RIS
AI 理解论文
溯源树
样例
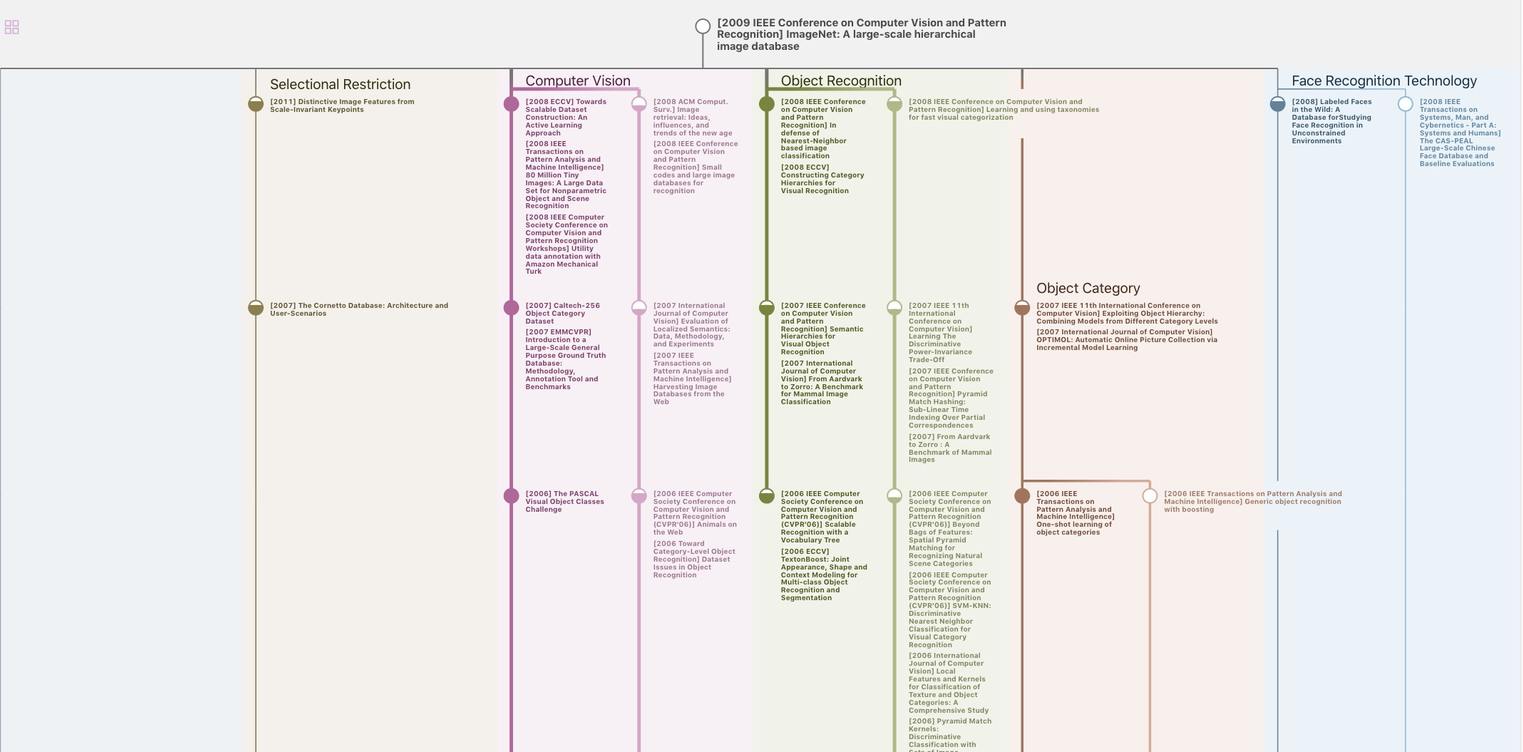
生成溯源树,研究论文发展脉络
Chat Paper
正在生成论文摘要