The ensemble multi-scale convolution neural network for visual target detection EEG-based brain-computer interfaces
Biomedical Signal Processing and Control(2024)
摘要
Human visual target detection based on electroencephalography (EEG) signals has been widely used to categorize target and non-target images, especially visual event-related potentials (ERPs), showing great promise. However, the methods based on deep learning of image-like input EEG signals seriously lose the original brain topographic information of EEG signals. Furthermore, current methods are limited by the extremely unbalanced distribution of target and non-target classes, resulting in insensitivity to feature learning for minority classes. In this paper, we propose a new approach to extract multi-scale spatial–temporal features of EEG signals using stacked multi-scale convolutional neural networks (MS_CNN) with raw spatially distributed EEG signals as input. The proposed novel MS_CNN extracts spatial features based on a two-dimensional (2D) grid distribution of electrode locations on the human scalp by stacking multi-scale 2D convolutions and extracting temporal features by convolutions with different window lengths. In addition, we adopt the fine-tuning ensemble method based on dynamic class weights to address minority target class samples being overwhelmed in the training process. Experimental evaluations on datasets with different class distributions show consistent improvements in performance both cross-session and cross-subject by incorporating the fine-tuning ensemble method into existing deep learning models. The combination of the fine-tuning ensemble architecture and MS_CNN is called the ensemble multi-scale convolution neural network (E_MS_CNN). Extensive experimental results show that the proposed E_MS_CNN method outperforms existing methods in cross-subject and cross-session situations.
更多查看译文
关键词
Electroencephalography (EEG),Event-related potentials (ERPs),Spatial–temporal features,Convolutional neural network (CNN)
AI 理解论文
溯源树
样例
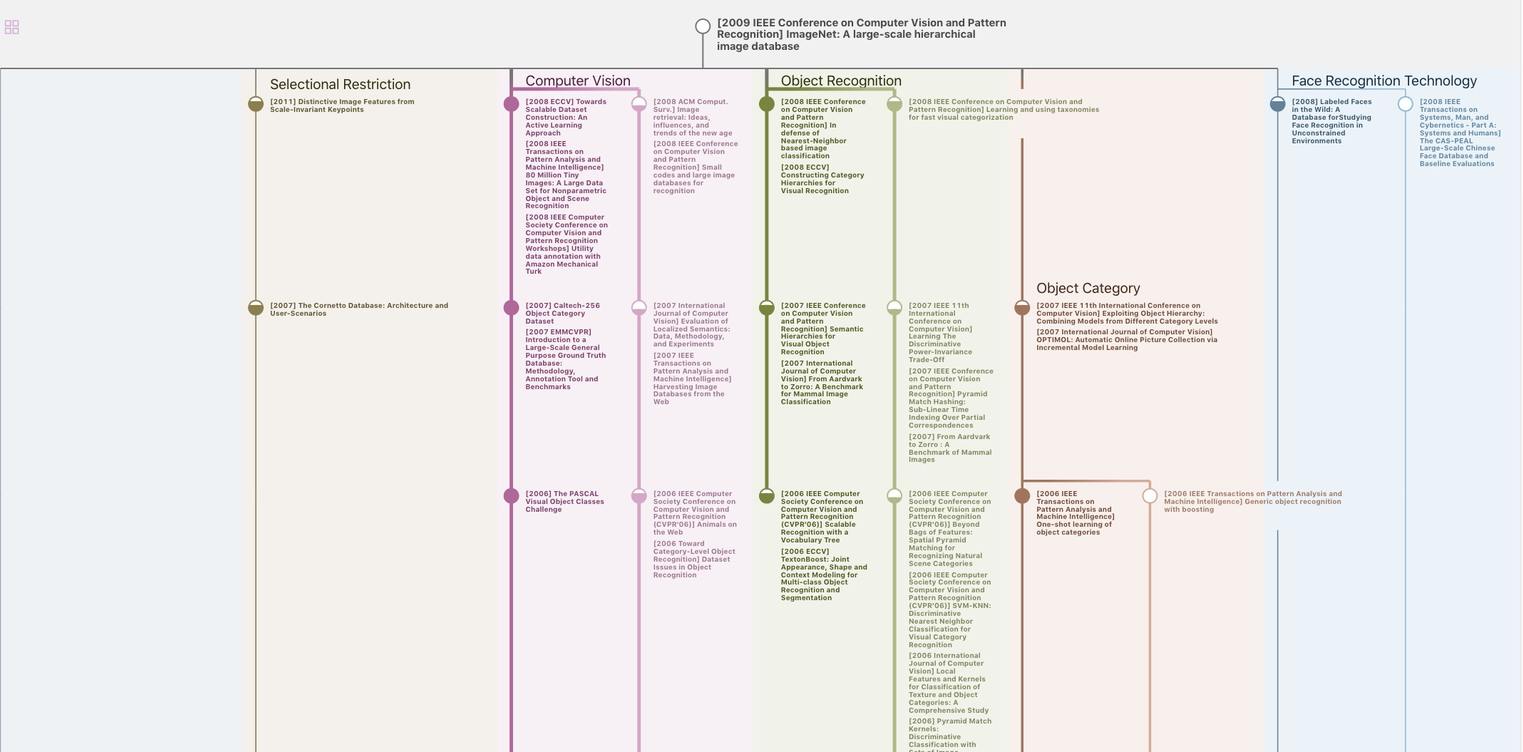
生成溯源树,研究论文发展脉络
Chat Paper
正在生成论文摘要