A High-Performance Rolling Bearing Fault Diagnosis Method Based on Adaptive Feature Mode Decomposition and Transformer
Applied Acoustics(2024)
Abstract
The fault diagnosis problems of rolling bearings commonly found in modern industrial equipment are becoming increasingly complex and challenging, and traditional fault diagnosis methods are unable to meet the requirements for high accuracy and strong robustness. To address this problem, this study proposes a high-performance rolling bearing fault diagnosis method based on adaptive feature mode decomposition (AFMD) and Transformer. Aiming at the problem that the decomposition performance of the traditional feature mode decomposition (FMD) is easily affected by its parameter settings, the coati optimization algorithm (COA) is applied to adaptively optimize the two key parameters of the FMD (the number of modes and the filter length). Further, due to the problems of slow convergence and easy to fall into local optimal solutions in the traditional COA, Tent chaotic mapping, dynamic refraction inverse learning and Levy flight strategy are introduced to improve the convergence speed and global search capability of the algorithm. Combining the above two methods and the excellent global feature learning ability of Transformer, the AFMD-Transformer fault diagnosis framework with high accuracy and strong robustness is constructed. The average experimental results on the two fault diagnosis datasets are 99.52% and 99.75%, respectively. The results show that our proposed diagnostic method outperforms currently popular methods in terms of accuracy and robustness.
MoreTranslated text
Key words
Adaptive feature mode decomposition,Parameter optimization,Bearing fault diagnosis,Transformer
AI Read Science
Must-Reading Tree
Example
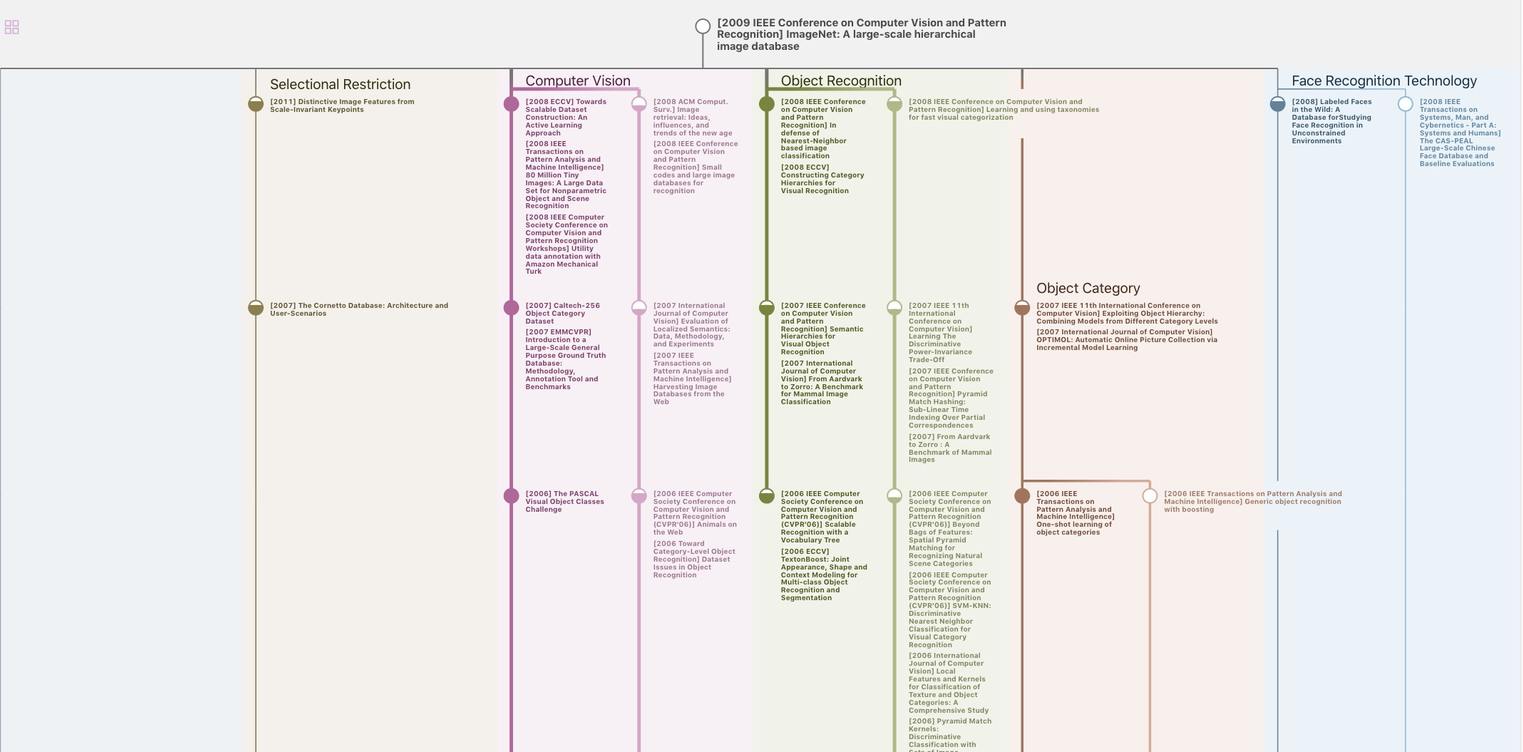
Generate MRT to find the research sequence of this paper
Chat Paper
Summary is being generated by the instructions you defined