OMG-Net: A Deep Learning Framework Deploying Segment Anything to Detect Pan-Cancer Mitotic Figures from Haematoxylin and Eosin-Stained Slides
arxiv(2024)
Abstract
Mitotic activity is an important feature for grading several cancer types. Counting mitotic figures (MFs) is a time-consuming, laborious task prone to inter-observer variation. Inaccurate recognition of MFs can lead to incorrect grading and hence potential suboptimal treatment. In this study, we propose an artificial intelligence (AI)-aided approach to detect MFs in digitised haematoxylin and eosin-stained whole slide images (WSIs). Advances in this area are hampered by the limited number and types of cancer datasets of MFs. Here we establish the largest pan-cancer dataset of mitotic figures by combining an in-house dataset of soft tissue tumours (STMF) with five open-source mitotic datasets comprising multiple human cancers and canine specimens (ICPR, TUPAC, CCMCT, CMC and MIDOG++). This new dataset identifies 74,620 MFs and 105,538 mitotic-like figures. We then employed a two-stage framework (the Optimised Mitoses Generator Network (OMG-Net) to classify MFs. The framework first deploys the Segment Anything Model (SAM) to automate the contouring of MFs and surrounding objects. An adapted ResNet18 is subsequently trained to classify MFs. OMG-Net reaches an F1-score of 0.84 on pan-cancer MF detection (breast carcinoma, neuroendocrine tumour and melanoma), largely outperforming the previous state-of-the-art MIDOG++ benchmark model on its hold-out testing set (e.g. +16 superior accuracy in detecting MFs on various types of tumours obtained with different scanners.
MoreTranslated text
AI Read Science
Must-Reading Tree
Example
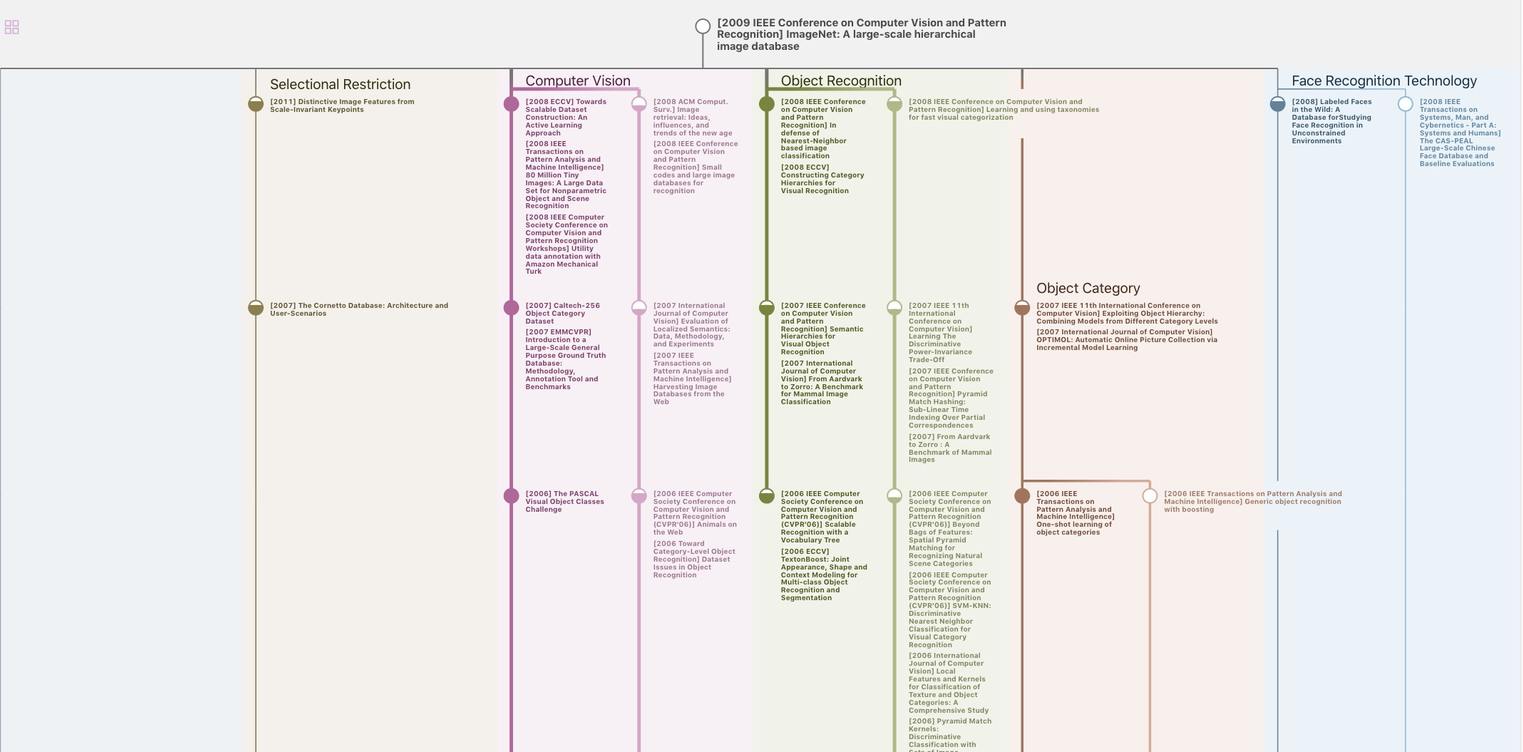
Generate MRT to find the research sequence of this paper
Chat Paper
Summary is being generated by the instructions you defined