A Novel Causal Discovery Method in Linear SEM with Structure Priors
2024 9th International Conference on Control and Robotics Engineering (ICCRE)(2024)
摘要
Causal discovery from observational data is a crucial but challenging task, and learning directed acyclic graphs (DAGs) is its foundation. The causal discovery method in linear structural equation models (SEMs) is one of the hot spots. However, almost all the existing methods have certain limitations, and an exact solution cannot always be identified. In this paper, a new heuristic algorithm for discovering causality was proposed, which provides a reasonable solution for combining structured priors or possible expert knowledge with heuristic search. We first identify the partial v-structures through partial correlation analysis as the structural priors of the following heuristic search algorithm. Second, through partial correlation analysis, we can also limit the search space we want to search for. Finally, to adapt to structural priors, an efficient particle swarm optimization (PSO) algorithm with an enhanced local search and a dynamic double-swarm strategy is proposed to improve the search capability. The experimental results demonstrate the effectiveness of the proposed methods when compared to the earlier state-of-the-art methods on six standard networks.
更多查看译文
关键词
causal discovery,structural equation models,partial correlation,particle swarm optimization
AI 理解论文
溯源树
样例
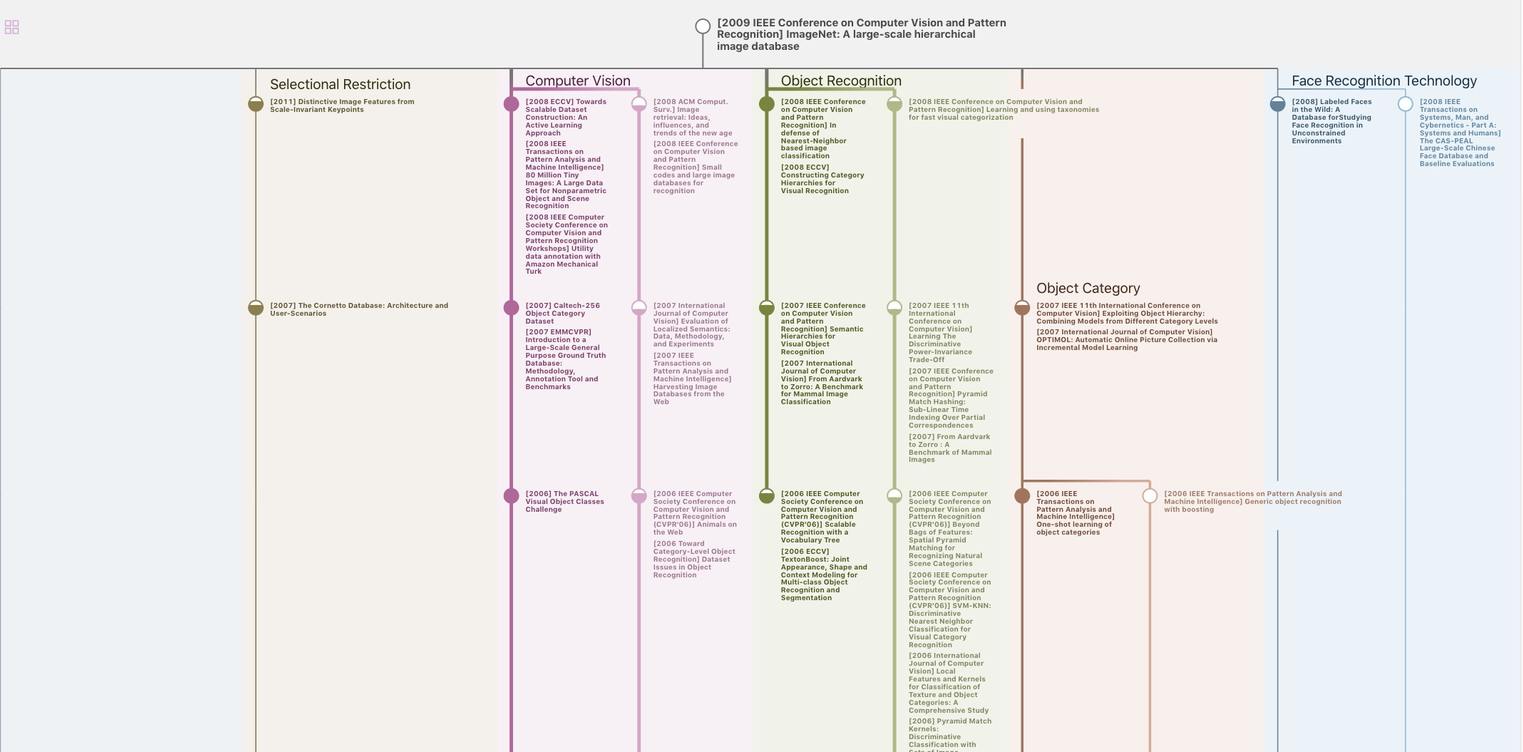
生成溯源树,研究论文发展脉络
Chat Paper
正在生成论文摘要