Universal Sound Separation with Self-Supervised Audio Masked Autoencoder
arxiv(2024)
Abstract
Universal sound separation (USS) is a task of separating mixtures of arbitrary sound sources. Typically, universal separation models are trained from scratch in a supervised manner, using labeled data. Self-supervised learning (SSL) is an emerging deep learning approach that leverages unlabeled data to obtain task-agnostic representations, which can benefit many downstream tasks. In this paper, we propose integrating a self-supervised pre-trained model, namely the audio masked autoencoder (A-MAE), into a universal sound separation system to enhance its separation performance. We employ two strategies to utilize SSL embeddings: freezing or updating the parameters of A-MAE during fine-tuning. The SSL embeddings are concatenated with the short-time Fourier transform (STFT) to serve as input features for the separation model. We evaluate our methods on the AudioSet dataset, and the experimental results indicate that the proposed methods successfully enhance the separation performance of a state-of-the-art ResUNet-based USS model.
MoreTranslated text
AI Read Science
Must-Reading Tree
Example
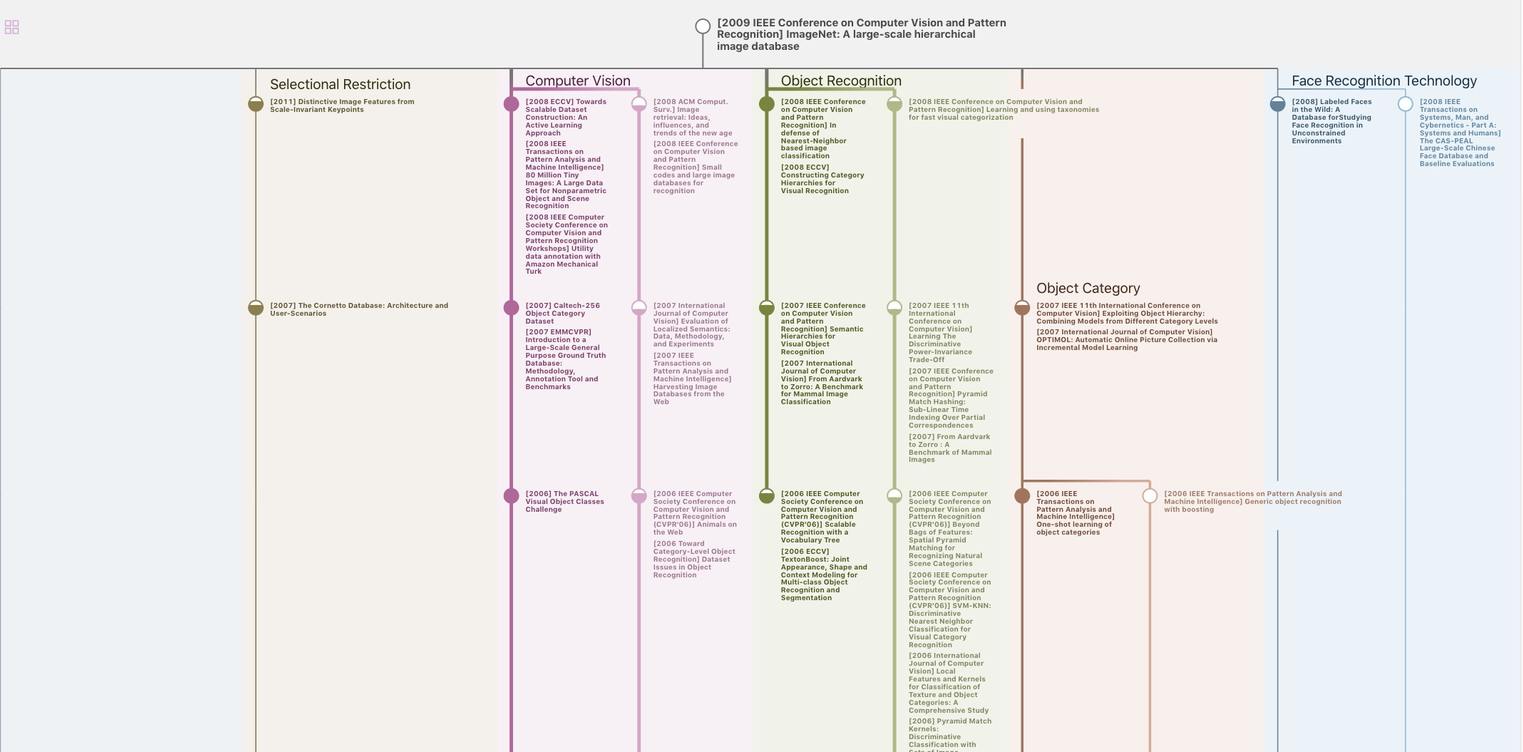
Generate MRT to find the research sequence of this paper
Chat Paper
Summary is being generated by the instructions you defined