Finding Meaning in Points: Weakly Supervised Semantic Segmentation for Event Cameras
arxiv(2024)
Abstract
Event cameras excel in capturing high-contrast scenes and dynamic objects, offering a significant advantage over traditional frame-based cameras. Despite active research into leveraging event cameras for semantic segmentation, generating pixel-wise dense semantic maps for such challenging scenarios remains labor-intensive. As a remedy, we present EV-WSSS: a novel weakly supervised approach for event-based semantic segmentation that utilizes sparse point annotations. To fully leverage the temporal characteristics of event data, the proposed framework performs asymmetric dual-student learning between 1) the original forward event data and 2) the longer reversed event data, which contain complementary information from the past and the future, respectively. Besides, to mitigate the challenges posed by sparse supervision, we propose feature-level contrastive learning based on class-wise prototypes, carefully aggregated at both spatial region and sample levels. Additionally, we further excavate the potential of our dual-student learning model by exchanging prototypes between the two learning paths, thereby harnessing their complementary strengths. With extensive experiments on various datasets, including DSEC Night-Point with sparse point annotations newly provided by this paper, the proposed method achieves substantial segmentation results even without relying on pixel-level dense ground truths. The code and dataset are available at https://github.com/Chohoonhee/EV-WSSS.
MoreTranslated text
AI Read Science
Must-Reading Tree
Example
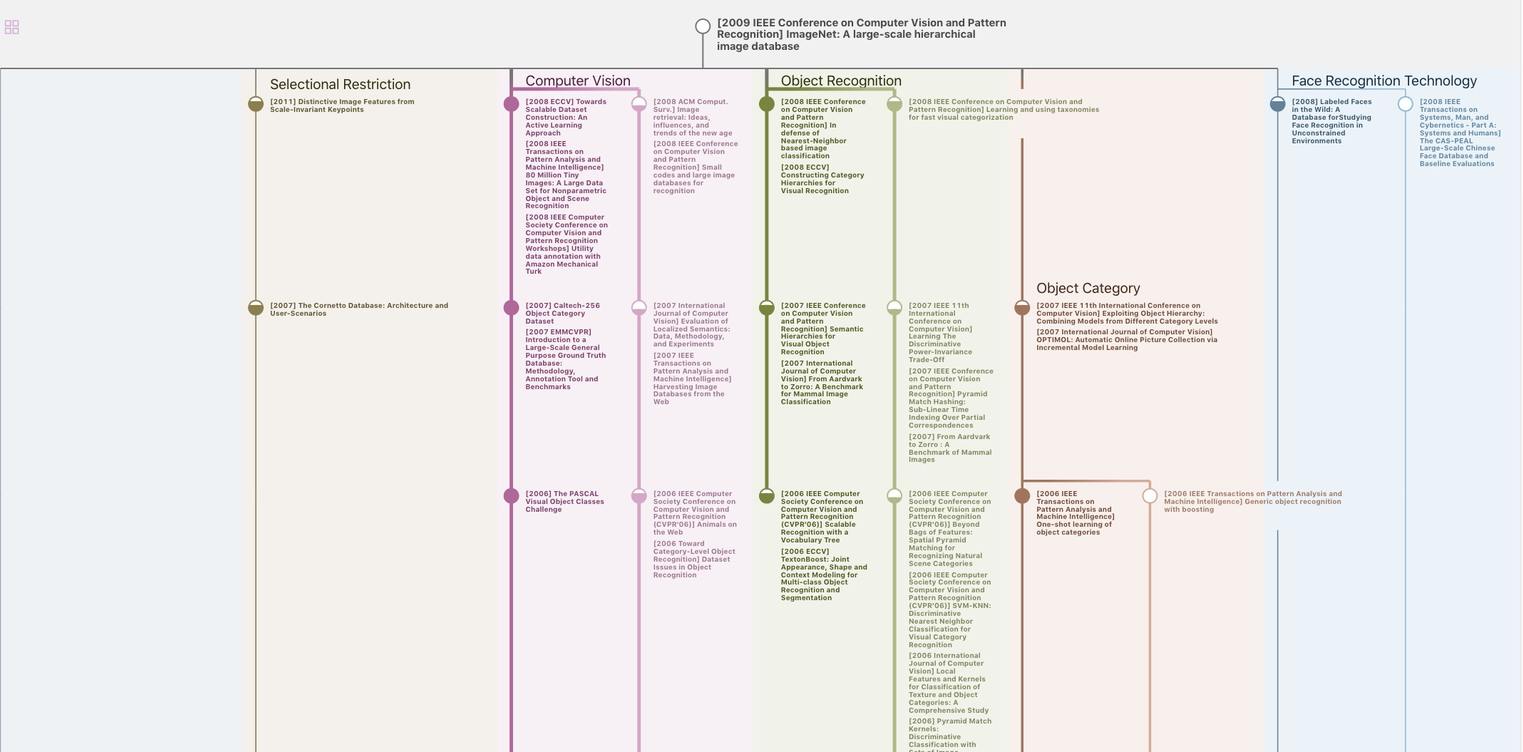
Generate MRT to find the research sequence of this paper
Chat Paper
Summary is being generated by the instructions you defined