A Review of Graph Neural Network Applications in Mechanics-Related Domains
arxiv(2024)
Abstract
Mechanics-related problems often present unique challenges in achieving accurate geometric and physical representations, particularly for non-uniform structures. Graph neural networks (GNNs) have emerged as a promising tool to tackle these challenges by adeptly learning from graph data with irregular underlying structures. Consequently, recent years have witnessed a surge in complex mechanics-related applications inspired by the advancements of GNNs. Despite this process, there is a notable absence of a systematic review addressing the recent advancement of GNNs in solving mechanics-related problems. To bridge this gap, this review article aims to provide an in-depth overview of the GNN applications in mechanics-related domains while identifying key challenges and outlining potential future research directions. In this review article, we begin by introducing the fundamental algorithms of GNNs that are widely employed in mechanics-related applications. We provide a concise explanation of their underlying principles to establish a solid understanding that will serve as a basis for exploring the applications of GNNs in mechanics-related domains. The scope of this paper is intended to cover the categorisation of literature into solid mechanics, fluid mechanics, and interdisciplinary mechanics-related domains, providing a comprehensive summary of graph representation methodologies, GNN architectures, and further discussions in their respective subdomains. Additionally, open data and source codes relevant to these applications are summarised for the convenience of future researchers. This article promotes an interdisciplinary integration of GNNs and mechanics and provides a guide for researchers interested in applying GNNs to solve complex mechanics-related problems.
MoreTranslated text
AI Read Science
Must-Reading Tree
Example
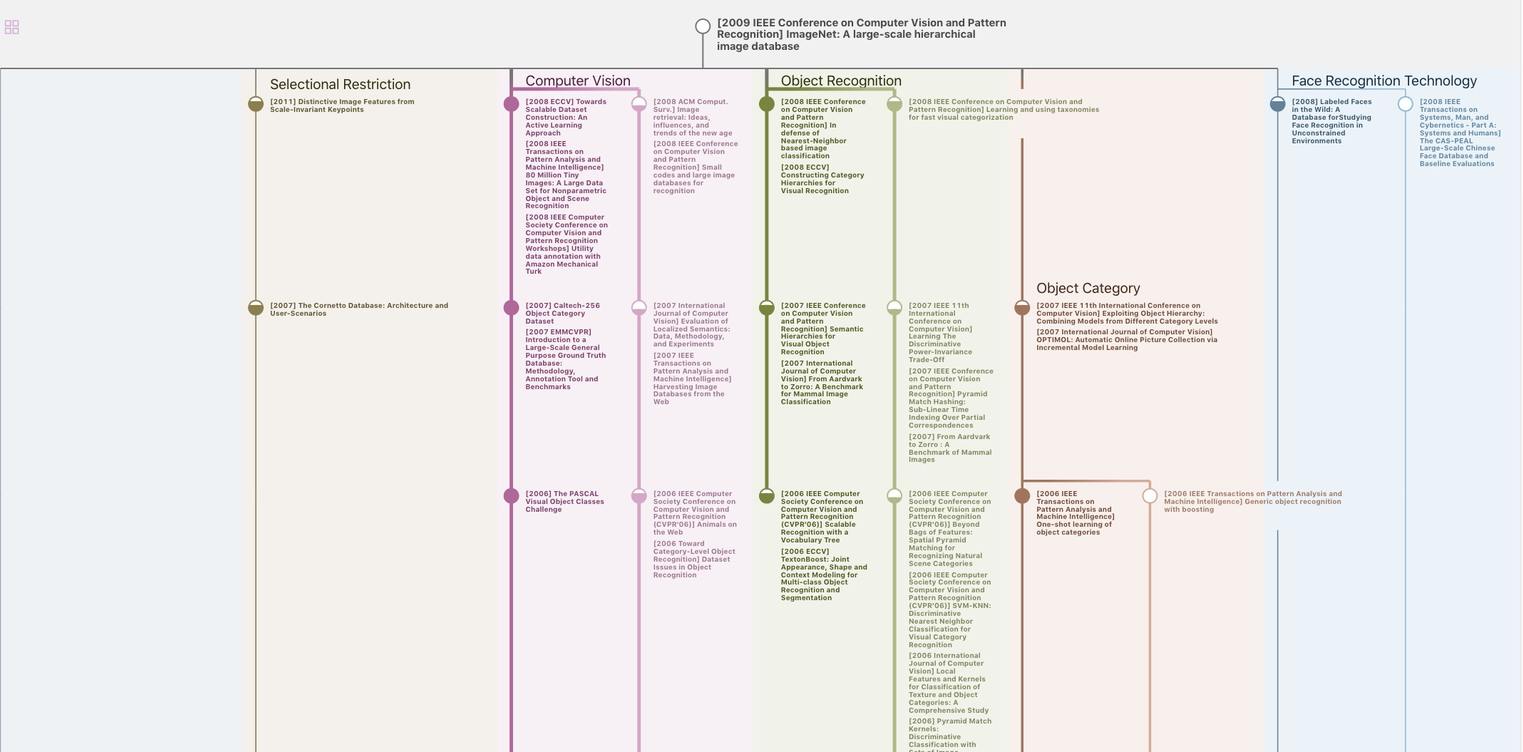
Generate MRT to find the research sequence of this paper
Chat Paper
Summary is being generated by the instructions you defined