Study on State of Health of Power Battery Prediction Using Denoising Autoencoder and Bidirectional Long Short-Term Memory Networks
2024 IEEE 7th International Electrical and Energy Conference (CIEEC)(2024)
摘要
The capacity of a power battery gradually decreases with long-term use and is reflected by the state of health. Accurately predicting the state of health of a power battery is critical to ensuring reliability in battery use. To improve the accuracy of state of health prediction for power batteries, a hybrid model is proposed in this paper to cope with the effects of noise and nonlinear degradation in the data on state of health prediction. Firstly, feature learning and extraction of the power battery data are performed using a denoising auto encoder, which is able to extract key information and capture variations over time from the data. Subsequently, the extracted features were modeled using a bidirectional long short-term memory network to capture complex patterns and nonlinear dependencies. The model proposed in this paper has been tested and validated on a public dataset, and the experimental results show that none of the evaluation metrics exceed the 3% error range, which aligns with the accuracy requirements of the battery health state prediction task. Therefore, it is verified that the model proposed in this paper can effectively improve the accuracy of the state of health prediction of power batteries.
更多查看译文
关键词
power battery,state of health,denoising autoen-coder,bidirectional long short-term memory,deep learning
AI 理解论文
溯源树
样例
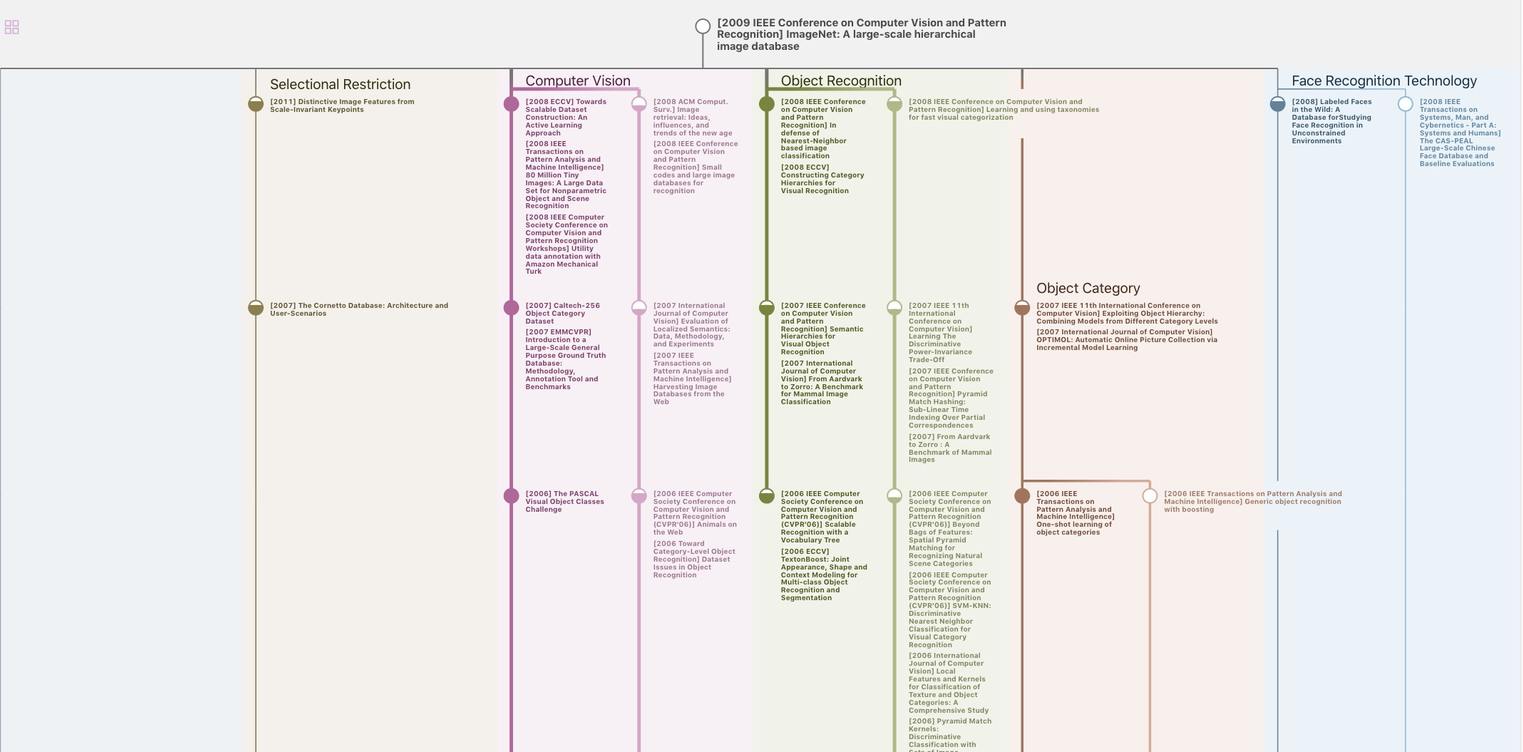
生成溯源树,研究论文发展脉络
Chat Paper
正在生成论文摘要