Speed Up! Cost-Effective Large Language Model for ADAS Via Knowledge Distillation
2024 IEEE Intelligent Vehicles Symposium (IV)(2024)
摘要
This paper presents a cost-effective approach to utilizing large language models (LLMs) as part of advanced driver-assistance systems (ADAS) through a knowledge-distilled model for driving assessment. LLMs have recently been employed across various domains. However, due to their size, they require sufficient computing infrastructure for deployment and ample time for generation. These characteristics make LLMs challenging to integrate into applications requiring real-time feedback, including ADAS. An existing study employed a vector database containing responses generated from an LLM to act as a surrogate model. However, this approach is limited when handling out-of-distribution (OOD) scenarios, which LLMs excel at. We propose a novel approach that utilizes a distilled model obtained from an established knowledge distillation technique to perform as a surrogate model for a target LLM, offering high resilience in handling OOD situations with substantially faster inference time. To assess the performance of the proposed approach, we also introduce a new dataset for driving scenarios and situations (DriveSSD), containing 124,248 records. Additionally, we augment randomly selected 12,425 records, 10% of our DriveSSD, with text embeddings generated from an embedding model. We distill the model using 10,000 augmented records and test all approaches on the remaining 2,425 records. We find that the distilled model introduced in this study has better performance across metrics, with half of the inference time used by the previous approach. We make our source code and data publicly available
1
.
更多查看译文
关键词
Alternative Models,Language Model,Inference Time,Advanced Driver Assistance Systems,Computational Infrastructure,Data Distribution,Data Generation,Environmental Data,Teacher Model,Small Model,Latent Space,Autonomous Vehicles,Exact Match,Generalization Capability,Distance Metrics,Subset Of Dataset,Binary String,Student Model,Vehicle State,Distillation Process,Feedback Messages
AI 理解论文
溯源树
样例
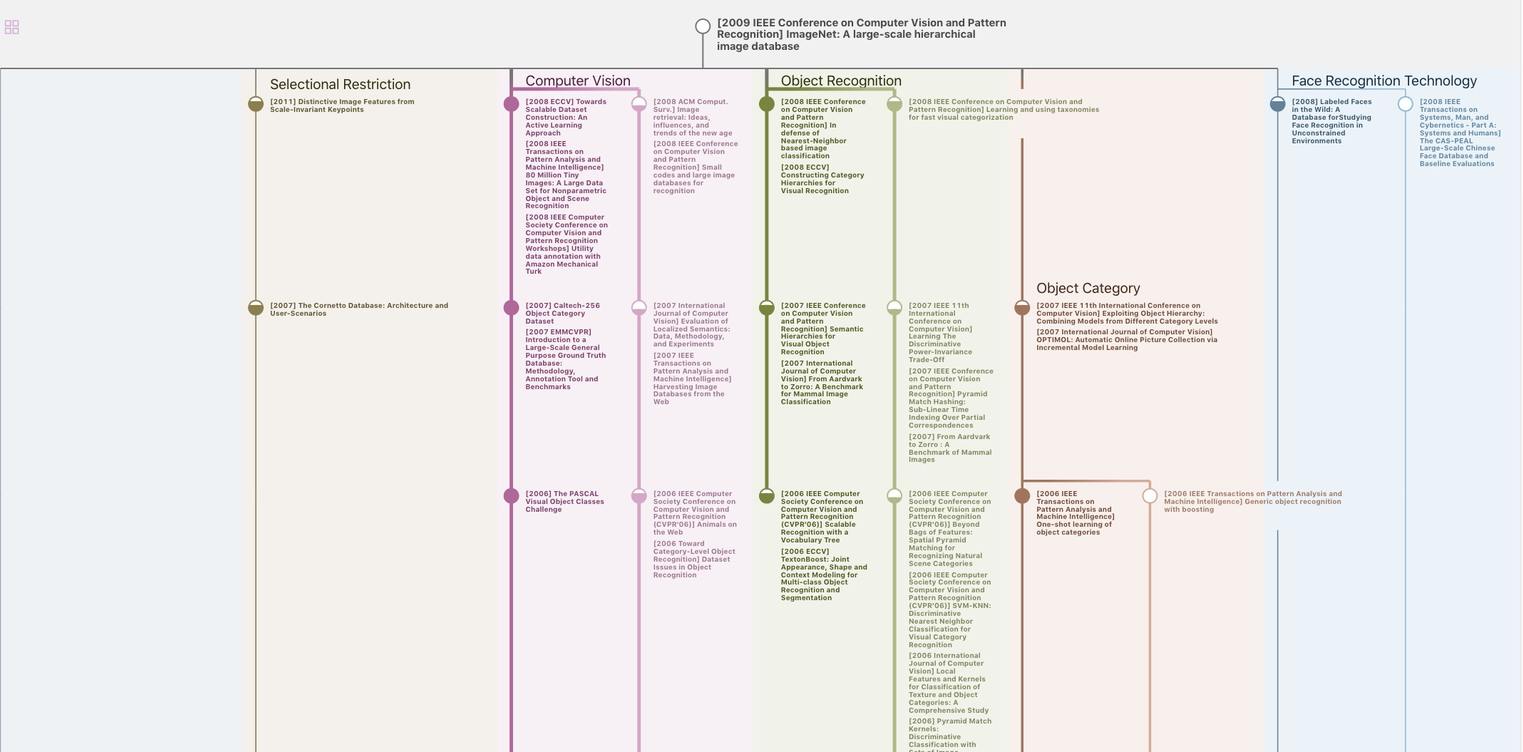
生成溯源树,研究论文发展脉络
Chat Paper
正在生成论文摘要