Gradual Batch Alternation for Effective Domain Adaptation in LiDAR-Based 3D Object Detection.
IEEE Intelligent Vehicles Symposium(2024)
摘要
We address the challenge of domain adaptation in LiDAR-based 3D object detection by introducing a simple yet effective training strategy known as Gradual Batch Alternation. This method enables adaptation from a well-labeled source domain to an insufficiently labeled target domain. Initially, training commences with alternating batches of samples from both the source and target domains. As the training progresses, we gradually reduce the number of samples from the source domain. Consequently, the model undergoes a gradual transition towards the target domain, resulting in improved adaptation. Domain adaptation experiments for 3D object detection on four benchmark autonomous driving datasets, namely ONCE, PandaSet, Waymo, and nuScenes, demonstrate significant performance gains over prior works and strong baselines.
更多查看译文
关键词
Object Detection,Domain Adaptation,3D Object Detection,LiDAR-based 3D Object Detection,Batch Of Samples,Target Domain,Source Domain,Strong Baseline,Labeled Target Domain,Data Sources,Point Cloud,Target Sample,Bounding Box,Baseline Methods,Performance Gap,Training Step,3D Point,3D Point Cloud,Prior Methods,LiDAR Sensor,3D Detection,Source Domain Data,Domain Adaptation Methods,Unsupervised Domain Adaptation Methods,Target Domain Data,Alternative Training,LiDAR Point Clouds,Domain Adaptation Techniques,Distribution Of Training Data,Target Data
AI 理解论文
溯源树
样例
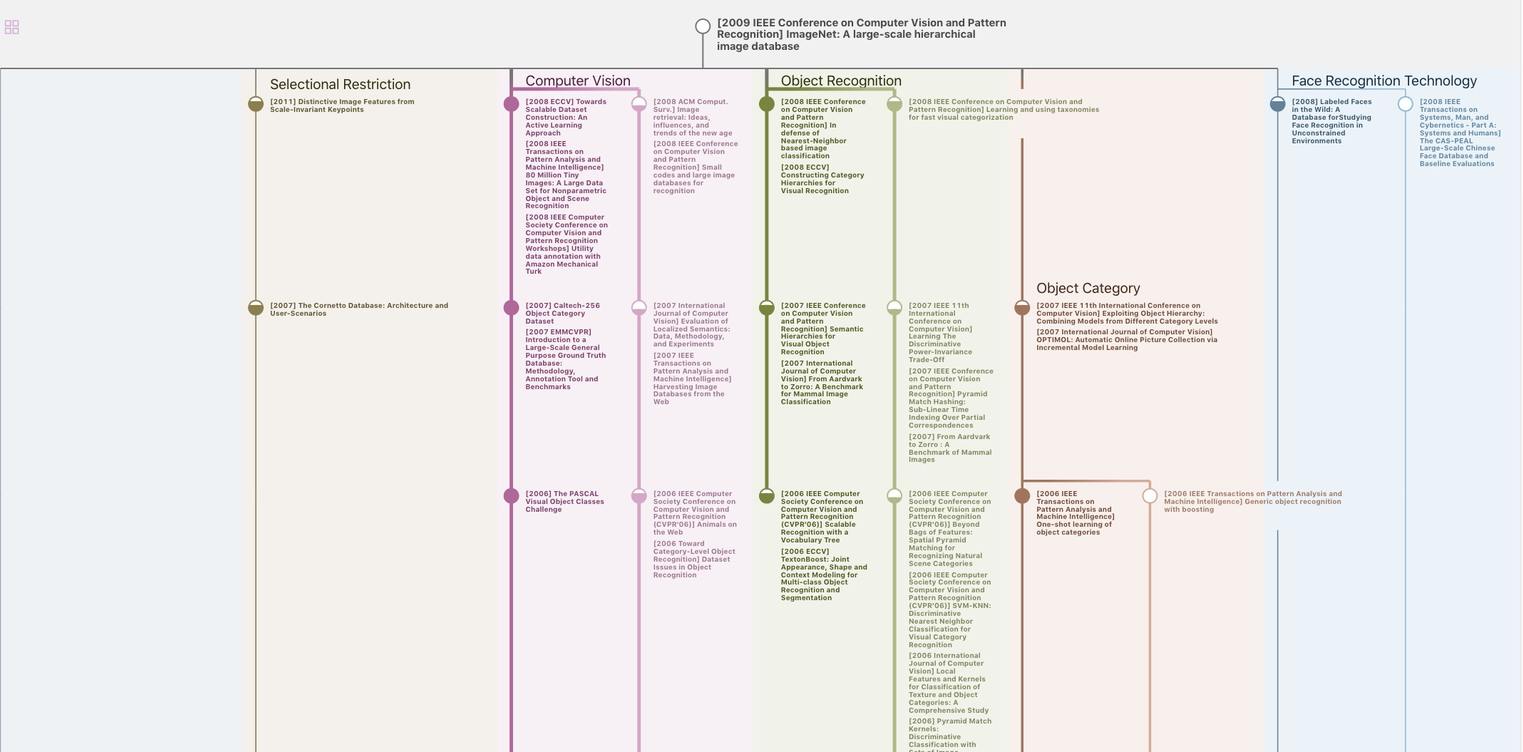
生成溯源树,研究论文发展脉络
Chat Paper
正在生成论文摘要