End-to-end Cooperative Localization via Neural Feature Sharing
2024 IEEE Intelligent Vehicles Symposium (IV)(2024)
摘要
Cooperative driving automation attracts great attention for its potential to improve traffic safety. Knowing each vehicle’s accurate position serves as the cornerstone for the information fusion necessary in cooperative driving tasks. However, inherent errors within a vehicle’s self-localization system often necessitate correction to facilitate cooperative perception and downstream tasks. Leveraging intermediate features shared among other Connected Automated Vehicles (CAVs), we propose an end-to-end learning localization framework aimed at estimating the relative pose error between the ego vehicle and the CAV. We investigate factors that may influence learning performance and validate the algorithm’s performance using a simulation dataset. The proposed method is compared with the traditional point cloud matching-based relative localization method. Remarkably, our framework effectively corrects relative pose errors even when the vehicle exhibits significant initial localization inaccuracies, and it can be integrated into the cooperative perception system.
更多查看译文
关键词
Cooperative Localization,Localization Accuracy,Point Cloud,Local Method,Perceptual Task,Perceptual System,Intermediate Features,Information Fusion,Automated Vehicles,Relative Pose,Pose Error,Root Mean Square Error,Feature Maps,Voxel Size,Local System,Bounding Box,Transformation Matrix,Position Error,Localization Error,Localization Task,LiDAR Point Clouds,Intermediate Feature Maps,Feature Map Size,Detection Head,Pose Information,Local Module,Pose Estimation,Inertial Navigation,Lidar Data,2D Convolutional Layers
AI 理解论文
溯源树
样例
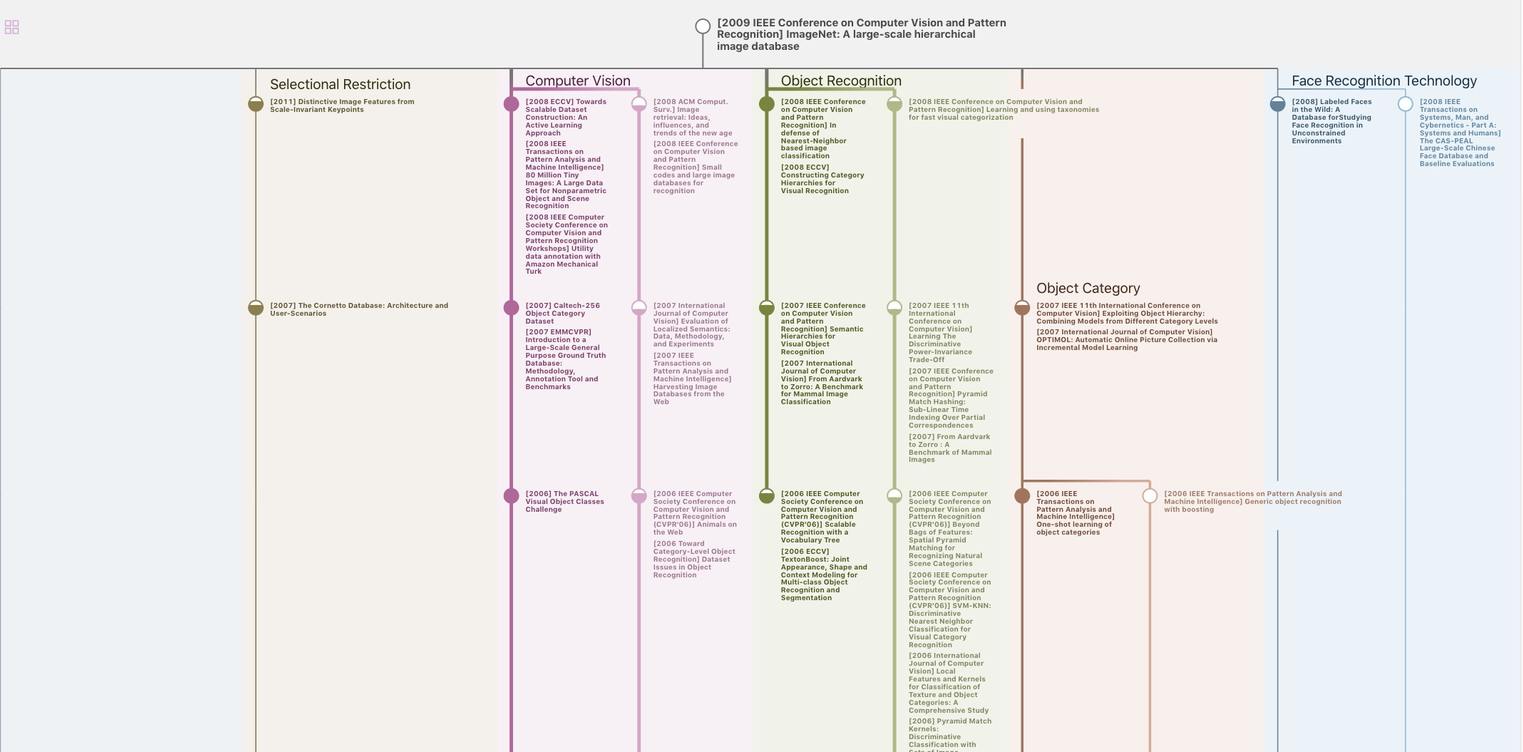
生成溯源树,研究论文发展脉络
Chat Paper
正在生成论文摘要