SWO: A Lightweight Window Spatiotemporal Attention Network Reconstructs Subsurface Temperature Structure
IEEE Journal of Selected Topics in Applied Earth Observations and Remote Sensing(2024)
摘要
Satellite remote sensing enables the extensive, long-term observation of oceanic changes. To achieve transparent ocean observation, artificial intelligence is innovatively used to reconstruct the three-dimensional ocean structure from remote sensing data with spatiotemporal attention. However, high-resolution satellite imagery imposes high computational demands. This study proposes Spatiotemporal Window Ocean (SWO), which is an efficient network that uses a special calculation strategy and spatiotemporal window attention to reduce complexity. The model integrates multiple satellite data sources, including sea surface temperature (SST), absolute dynamic topography (ADT), and sea surface salinity (SSS). Our experimental results demonstrate that SWO achieves lower computational costs and superior performance compared with recent commonly used spatiotemporal sequence models. Specifically, SWO requires a training time equivalent to 1/3 of SimVP, 1/4 of PredRNN, and 1/5 of SA-ConvLSTM while achieving a root mean square error (RMSE) index that is 13.8%, 20.3%, and 13.2% better, respectively. The computational advantages of SWO offer an important technical means for the high-resolution reconstruction of ocean phenomena in the future.
更多查看译文
关键词
Temperature structure reconstruction,lightweight,Spatiotemporal Window Ocean,spatiotemporal attention mechanism,satellite observations
AI 理解论文
溯源树
样例
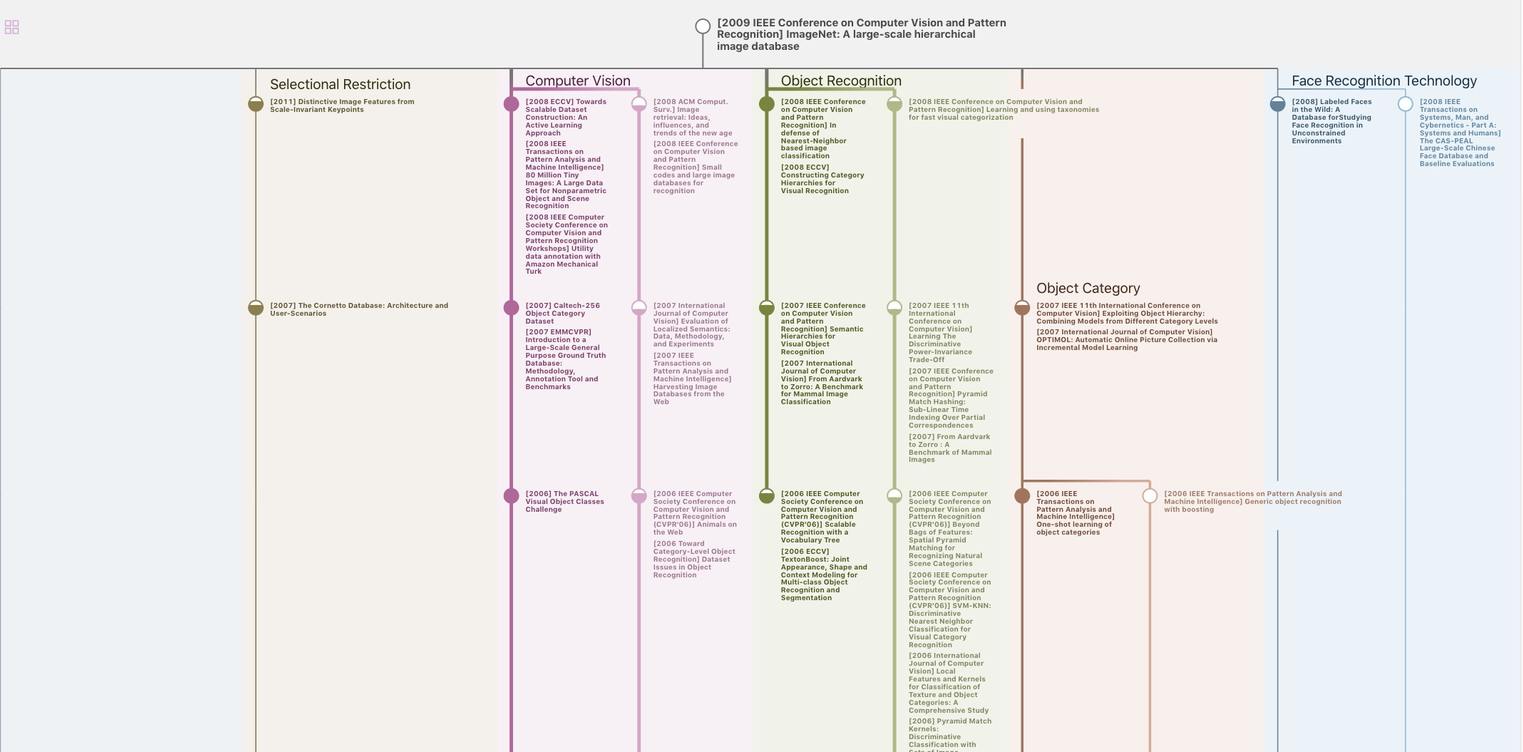
生成溯源树,研究论文发展脉络
Chat Paper
正在生成论文摘要