Representation Learning and Information Retrieval.
Annual International ACM SIGIR Conference on Research and Development in Information Retrieval(2024)
摘要
How to best represent words, documents, queries, entities, relations, and other variables in information retrieval (IR) and related applications has been a fundamental research question for decades. Early IR systems relied on the independence assumptions about words and documents for simplicity and scalability, which were clearly sub-optimal from a semantic point of view. The rapid development of deep neural networks in the past decade has revolutionized the representation learning technologies for contextualized word embedding and graph-enhanced document embedding, leading to the new era of dense IR. This talk highlights such impactful shifts in representation learning for IR and related areas, the new challenges coming along and the remedies, including our recent work in large-scale dense IR [1, 9], in graph-based reasoning for knowledge-enhanced predictions [10], in self-refinement of large language models (LLMs) with retrieval augmented generation (RAG)[2,7] and iterative feedback [3,4], in principle-driven self-alignment of LLMs with minimum human supervision [6], etc. More generally, the power of such deep learning goes beyond IR enhancements, e.g., for significantly improving the state-of-the-art solvers for NP-Complete problems in classical computer science [5,8].
更多查看译文
AI 理解论文
溯源树
样例
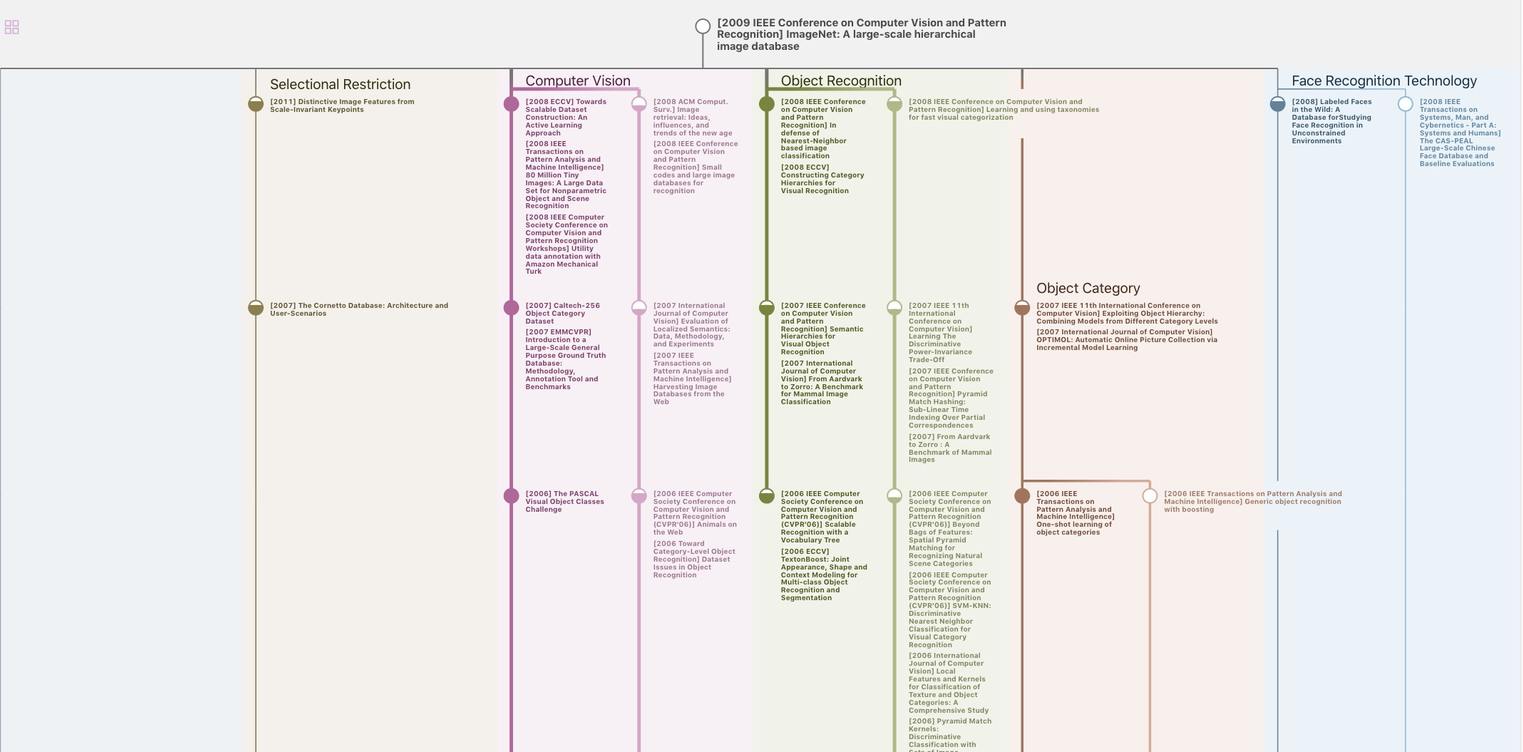
生成溯源树,研究论文发展脉络
Chat Paper
正在生成论文摘要