Screening Targets and Therapeutic Drugs for Alzheimer's Disease Based on Deep Learning Model and Molecular Docking.
Journal of Alzheimer's disease : JAD(2024)
摘要
Background:Alzheimer's disease (AD) is a neurodegenerative disorder caused by a complex interplay of various factors. However, a satisfactory cure for AD remains elusive. Pharmacological interventions based on drug targets are considered the most cost-effective therapeutic strategy. Therefore, it is paramount to search potential drug targets and drugs for AD.
Objective:We aimed to provide novel targets and drugs for the treatment of AD employing transcriptomic data of AD and normal control brain tissues from a new perspective.
Methods:Our study combined the use of a multi-layer perceptron (MLP) with differential expression analysis, variance assessment and molecular docking to screen targets and drugs for AD.
Results:We identified the seven differentially expressed genes (DEGs) with the most significant variation (ANKRD39, CPLX1, FABP3, GABBR2, GNG3, PPM1E, and WDR49) in transcriptomic data from AD brain. A newly built MLP was used to confirm the association between the seven DEGs and AD, establishing these DEGs as potential drug targets. Drug databases and molecular docking results indicated that arbaclofen, baclofen, clozapine, arbaclofen placarbil, BML-259, BRD-K72883421, and YC-1 had high affinity for GABBR2, and FABP3 bound with oleic, palmitic, and stearic acids. Arbaclofen and YC-1 activated GABAB receptor through PI3K/AKT and PKA/CREB pathways, respectively, thereby promoting neuronal anti-apoptotic effect and inhibiting p-tau and Aβ formation.
Conclusions:This study provided a new strategy for the identification of targets and drugs for the treatment of AD using deep learning. Seven therapeutic targets and ten drugs were selected by using this method, providing new insight for AD treatment.
更多查看译文
AI 理解论文
溯源树
样例
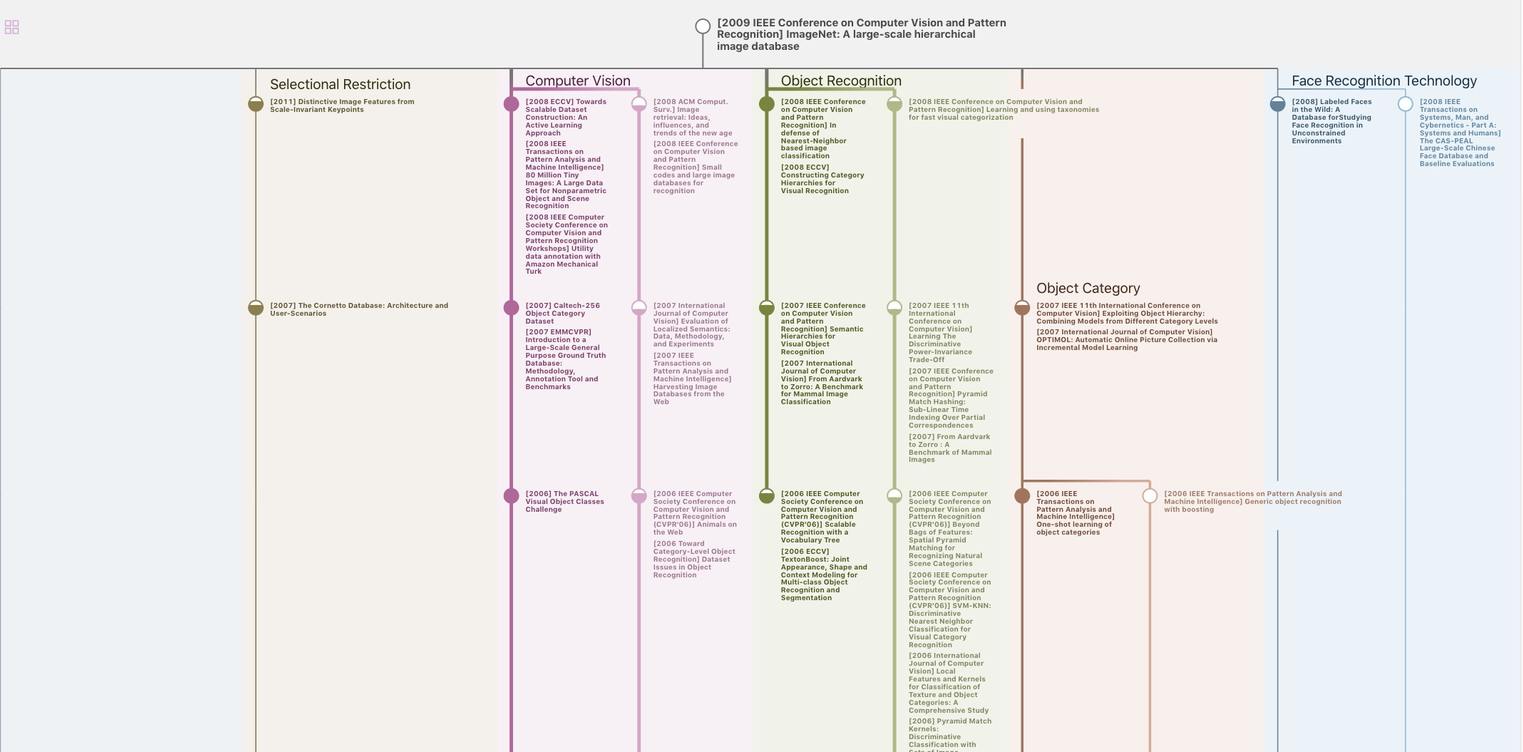
生成溯源树,研究论文发展脉络
Chat Paper
正在生成论文摘要