Single-Image Shadow Removal Using Deep Learning: A Comprehensive Survey
arxiv(2024)
Abstract
Shadow removal aims at restoring the image content within shadow regions,
pursuing a uniform distribution of illumination that is consistent between
shadow and non-shadow regions. Comparing to other image restoration tasks,
there are two unique challenges in shadow removal: 1) The patterns of shadows
are arbitrary, varied, and often have highly complex trace structures, making
“trace-less” image recovery difficult. 2) The degradation caused by shadows
is spatially non-uniform, resulting in inconsistencies in illumination and
color between shadow and non-shadow areas. Recent developments in this field
are primarily driven by deep learning-based solutions, employing a variety of
learning strategies, network architectures, loss functions, and training data.
Nevertheless, a thorough and insightful review of deep learning-based shadow
removal techniques is still lacking. In this paper, we are the first to provide
a comprehensive survey to cover various aspects ranging from technical details
to applications. We highlight the major advancements in deep learning-based
single-image shadow removal methods, thoroughly review previous research across
various categories, and provide insights into the historical progression of
these developments. Additionally, we summarize performance comparisons both
quantitatively and qualitatively. Beyond the technical aspects of shadow
removal methods, we also explore potential future directions for this field.
MoreTranslated text
AI Read Science
Must-Reading Tree
Example
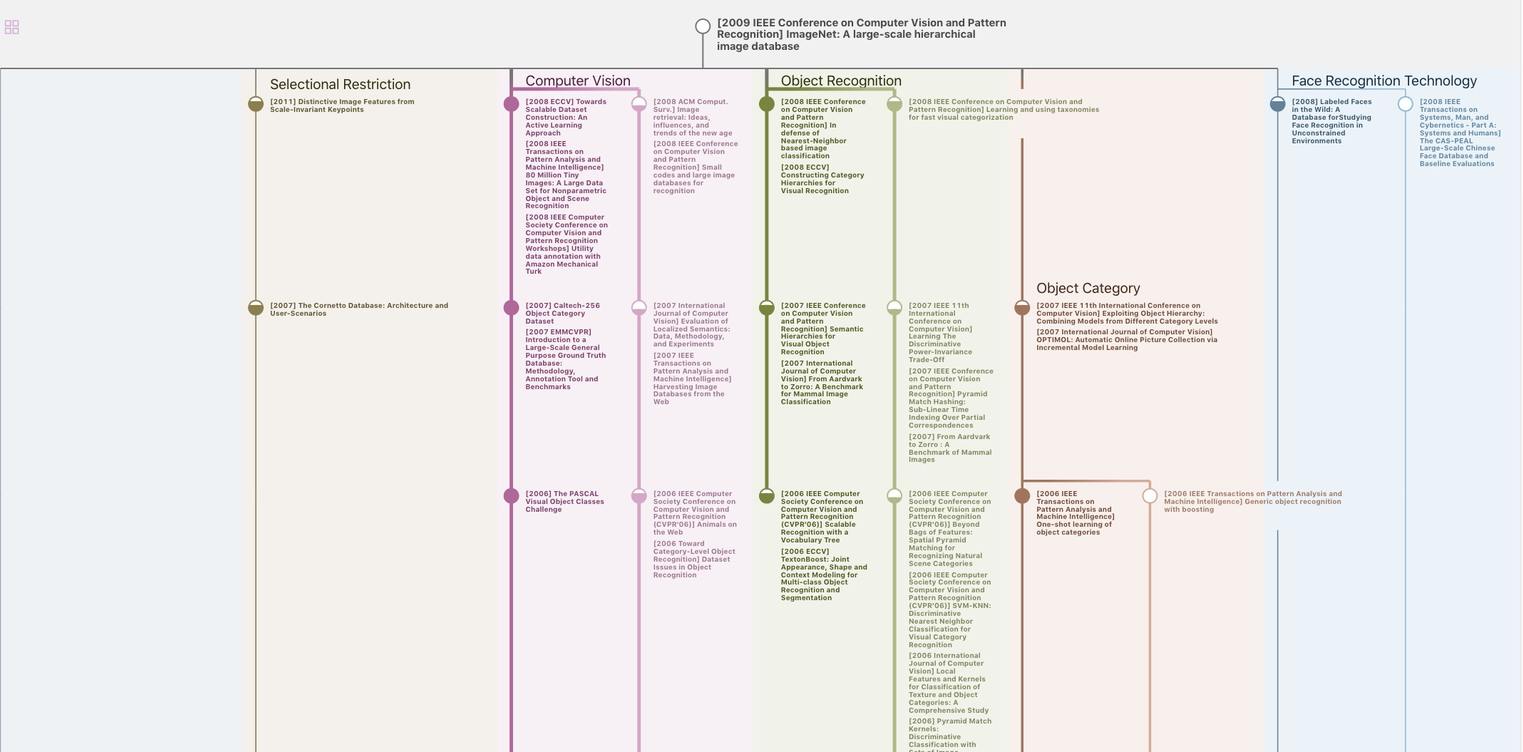
Generate MRT to find the research sequence of this paper
Chat Paper
Summary is being generated by the instructions you defined