Parameter-efficient Fine-Tuning of Whisper for Low-Resource Speech Recognition
2024 5th International Seminar on Artificial Intelligence, Networking and Information Technology (AINIT)(2024)
摘要
Limited data availability remains a significant challenge for Whisper's low-resource speech recognition performance, falling short of practical application requirements. While previous studies have successfully reduced the recognition error rates of target language speech through fine-tuning, a comprehensive exploration and analysis of Whisper's parameter-efficient fine-tuning capabilities, the advantages and disadvantages are still lacking. This paper aims to fill this gap by conducting comprehensive experimental exploration for Whisper's low-resource speech recognition performance using two parameter-efficient fine-tuning strategies with limited supervised data from multiple low-resource languages. The results and analysis demonstrate that all fine-tuning strategies explored in this paper significantly enhance Whisper's performance. However, different strategies vary in their suitability and practical effectiveness, highlighting the need for careful selection based on specific use cases and resources available.
更多查看译文
关键词
Whisper,parameter-efficient fine-tuning,low-resource ASR
AI 理解论文
溯源树
样例
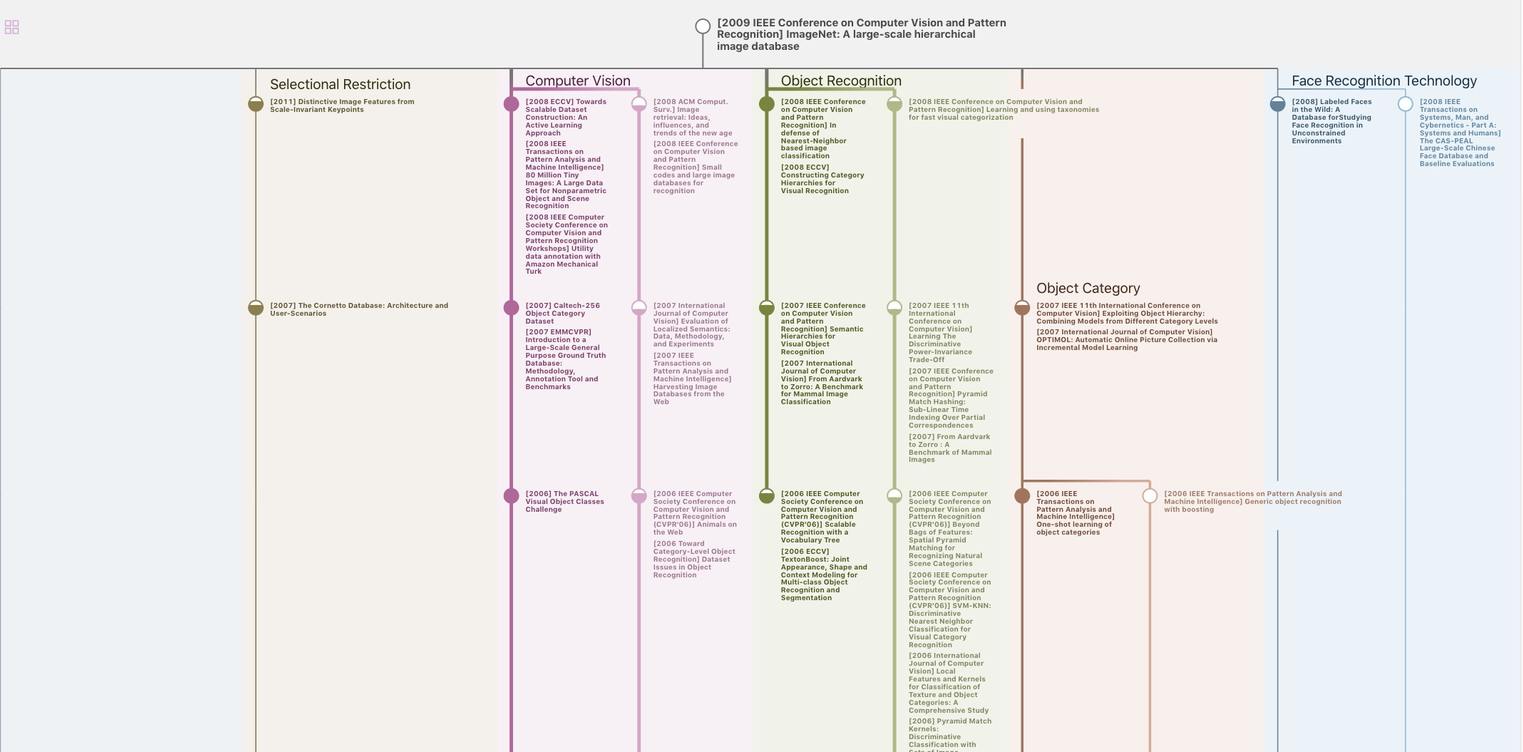
生成溯源树,研究论文发展脉络
Chat Paper
正在生成论文摘要