Social-MAE: A Transformer-Based Multimodal Autoencoder for Face and Voice
2024 IEEE 18th International Conference on Automatic Face and Gesture Recognition (FG)(2024)
摘要
Human social behaviors are inherently multi-modal necessitating the development of powerful audiovisual models for their perception. In this paper, we present Social-MAE, our pre-trained audiovisual Masked Autoencoder based on an extended version of Contrastive Audio-Visual Masked Auto-Encoder (CAV-MAE), which is pre-trained on audiovisual social data. Specifically, we modify CAV-MAE to receive a larger number of frames as input and pre-train it on a large dataset of human social interaction (VoxCeleb2) in a self-supervised manner. We demonstrate the effectiveness of this model by fine-tuning and evaluating the model on different social and affective downstream tasks, namely, emotion recognition, laughter detection and apparent personality estimation. The model achieves state-of-the-art results on multimodal emotion recognition and laughter recognition and competitive results for apparent personality estimation, demonstrating the effectiveness of in-domain self-supervised pre-training. Code and model weight are available here https://github.com/HuBohy/SocialMAE.
更多查看译文
关键词
Human Behavior,Emotion Recognition,Social Task,Learning Rate,Personality Traits,Utterances,Mean Absolute Error,Input Sequence,Representation Learning,Visual Input,Human Faces,Happy Faces,Linear Layer,Self-supervised Learning,Relevant Tasks,Multiple Frames,Reconstruction Loss,Contrastive Loss,Multimodal Model,Transformer Layers,Audio Input,Decoder Output,Pretext Task,Cross-entropy Loss,Normalization Layer
AI 理解论文
溯源树
样例
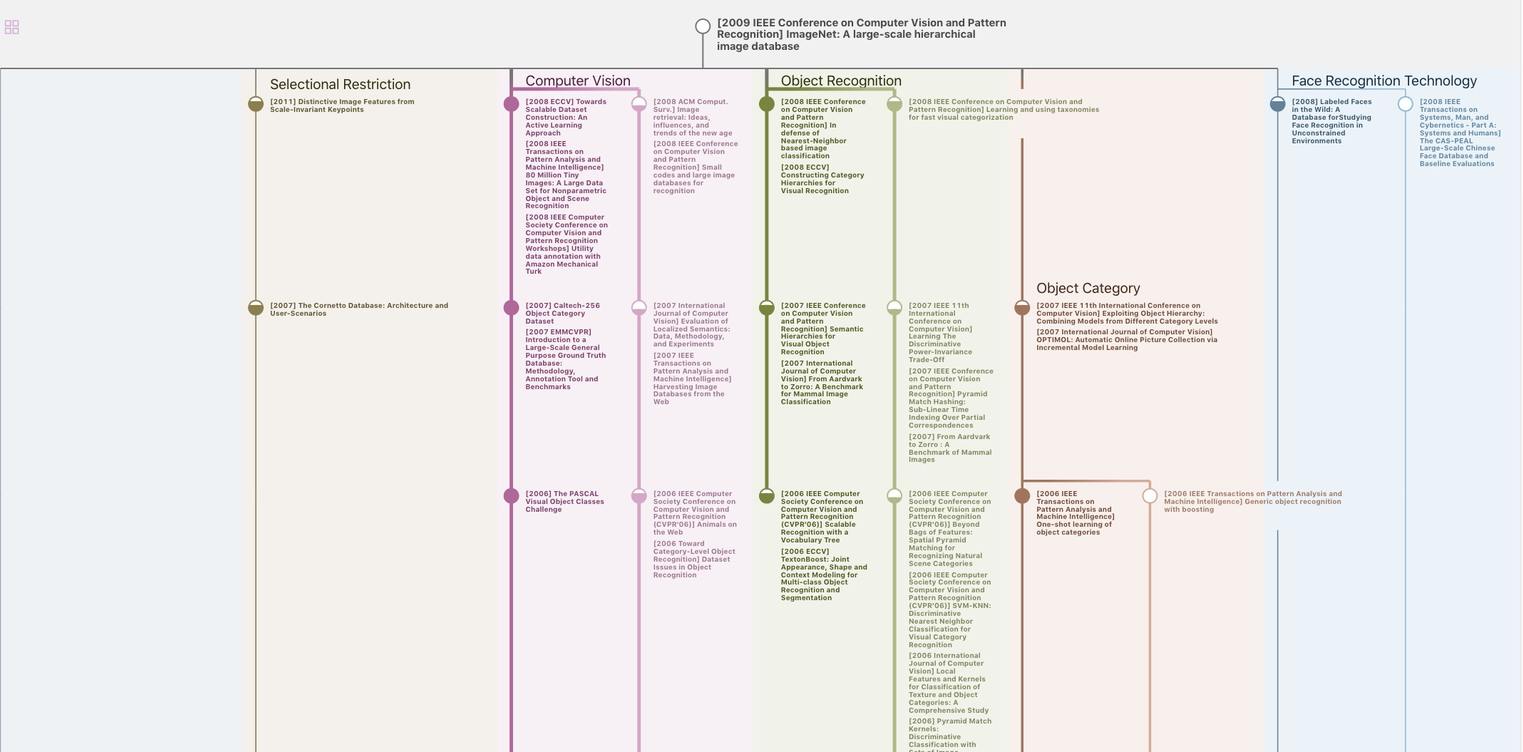
生成溯源树,研究论文发展脉络
Chat Paper
正在生成论文摘要