EUNet: Edge-UNet for Accurate Building Extraction and Edge Emphasis in Gaofen-7 Images
Remote Sensing(2024)
Abstract
Deep learning is currently the mainstream approach for building extraction tasks in remote-sensing imagery, capable of automatically learning features of buildings in imagery and yielding satisfactory extraction results. However, due to the diverse sizes, irregular layouts, and complex spatial relationships of buildings, extracted buildings often suffer from incompleteness and boundary issues. Gaofen-7 (GF-7), as a high-resolution stereo mapping satellite, provides well-rectified images from its rear-view imagery, which helps mitigate occlusions in highly varied terrain, thereby offering rich information for building extraction. To improve the integrity of the edges of the building extraction results, this paper proposes a dual-task network (Edge-UNet, EUnet) based on UNet, incorporating an edge extraction branch to emphasize edge information while predicting building targets. We evaluate this method using a self-made GF-7 Building Dataset, the Wuhan University (WHU) Building Dataset, and the Massachusetts Buildings Dataset. Comparative analysis with other mainstream semantic segmentation networks reveals significantly higher F1 scores for the extraction results of our method. Our method exhibits superior completeness and accuracy in building edge extraction compared to unmodified algorithms, demonstrating robust performance.
MoreTranslated text
Key words
building extraction,semantic segmentation networks,GF-7,UNet,deep learning
AI Read Science
Must-Reading Tree
Example
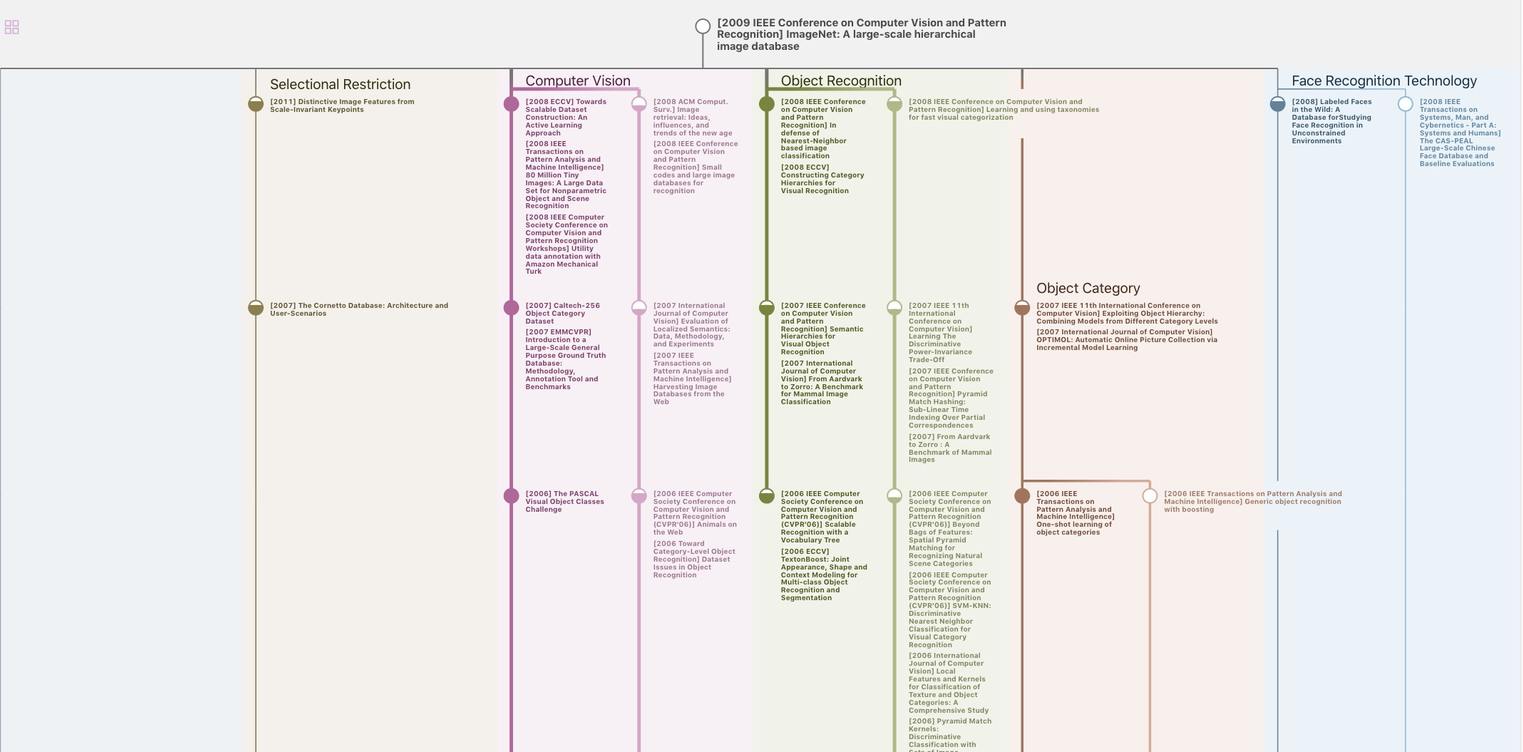
Generate MRT to find the research sequence of this paper
Chat Paper
Summary is being generated by the instructions you defined