Improving Flood Forecasting Skill by Combining Ensemble Precipitation Forecasts and Multiple Hydrological Models in a Mountainous Basin
Water(2024)
摘要
Ensemble precipitation forecasts (EPFs) derived from single numerical weather predictions (NWPs) often miss extreme events, and individual hydrological models (HMs) often fail to accurately capture all types of flows, including flood peaks. To address these shortcomings, this study introduced four “EPF + HM” schemes for ensemble flood forecasting (EFF) by combining two EPFs and two HMs. A generator-based post-processing (GPP) method was applied to correct biases and under-dispersion within the raw EPF data. The effectiveness of these schemes in delivering high-quality flood forecasts was assessed using both deterministic and probabilistic metrics. The results indicate that, once post-processed by GPP, all proposed schemes show improvements in both deterministic and probabilistic performances, with skillful flood forecasts for 1–7 lead days. The deterioration in forecast performance with extended lead times is also lessened. Notably, the results indicate that uncertainty within hydrological models has a more pronounced impact on capturing flood peaks than uncertainty in precipitation inputs. This study recommends combining individual EPF with multiple hydrological models for reliable flood forecasting. In conclusion, effective flood forecasting necessitates employing post-processing techniques to correct EPFs and accounting for the uncertainty inherent in hydrological models, rather than relying solely on the uncertainty of the input data.
更多查看译文
关键词
ensemble flood forecasting,grand ensemble,post-processing,multiple hydrological models,evaluation
AI 理解论文
溯源树
样例
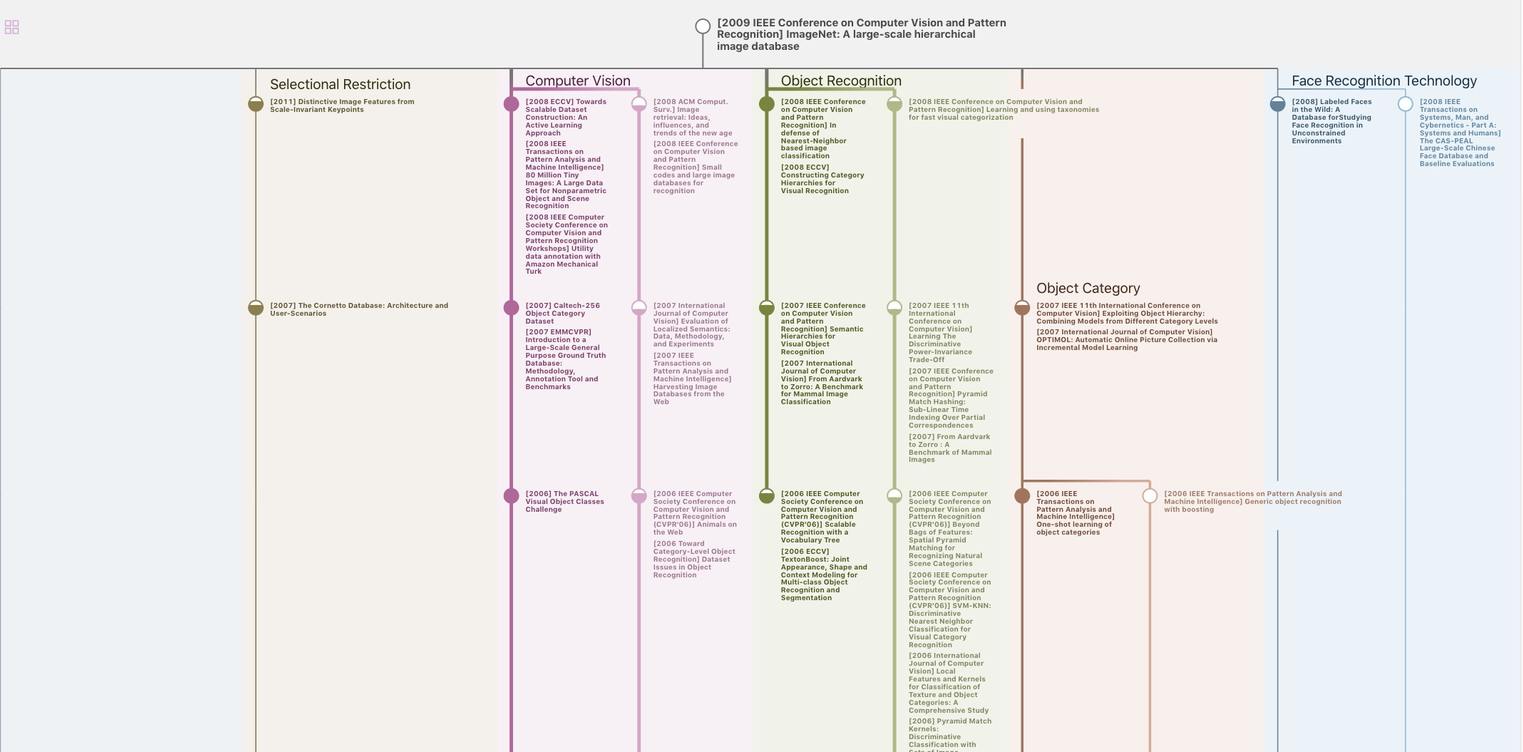
生成溯源树,研究论文发展脉络
Chat Paper
正在生成论文摘要