Fast Universal Azimuth Signal Modeling for Maneuvering-Platform BFSAR Imaging.
IEEE Trans. Geosci. Remote. Sens.(2024)
摘要
Appropriate modeling and processing of echoes are the foundations for high-precision frequency-domain synthetic aperture radar (SAR) imaging. The complex geometry makes it challenging to accurately characterize and cope with the 2-D spatial variation of Doppler modulation in maneuvering-platform translational-variant bistatic forward-looking SAR (MTV-BFSAR), resulting in that the azimuth processing method by means of setting reference points on the Cartesian coordinate axis significantly impairs the performance in terms of robustness, accuracy, and efficiency. This article proposes a comprehensive MTV-BFSAR imaging algorithm based on universal frequency-domain azimuth signal modeling (UFDASM). The presented methodology utilizes the bistatic bisector to form the azimuth reference line (ARL) and develops two expeditious ARL and range isoline (RIL) coordinates’ solving techniques, which substantially augments the reliability and efficiency of the space-variant Doppler modulation coefficient (DMC) representation, reduces the order, and improves the robustness of the entire algorithm. Subsequently, thanks to UFDASM, a modified nonlinear chirp scaling (NLCS) method with orthogonal impulse response function (IRF) is derived to eliminate the spatial variation of the quadratic DMC in the range-Doppler domain while omitting that of the cubic one. Furthermore, one may find that the equalization of the second-order spatial variation introduces a cubic phase error (CPE) term. However, boundary analyses manifest that this error is small enough not to affect the imaging performance in MTV-BFSAR. Finally, the accuracy, robustness, and efficiency of the approach are validated through numerical simulation and raw data processing.
更多查看译文
关键词
Bistatic forward-looking synthetic aperture radar (BFSAR),nonlinear chirp scaling (NLCS),universal frequency-domain azimuth signal modeling (UFDASM)
AI 理解论文
溯源树
样例
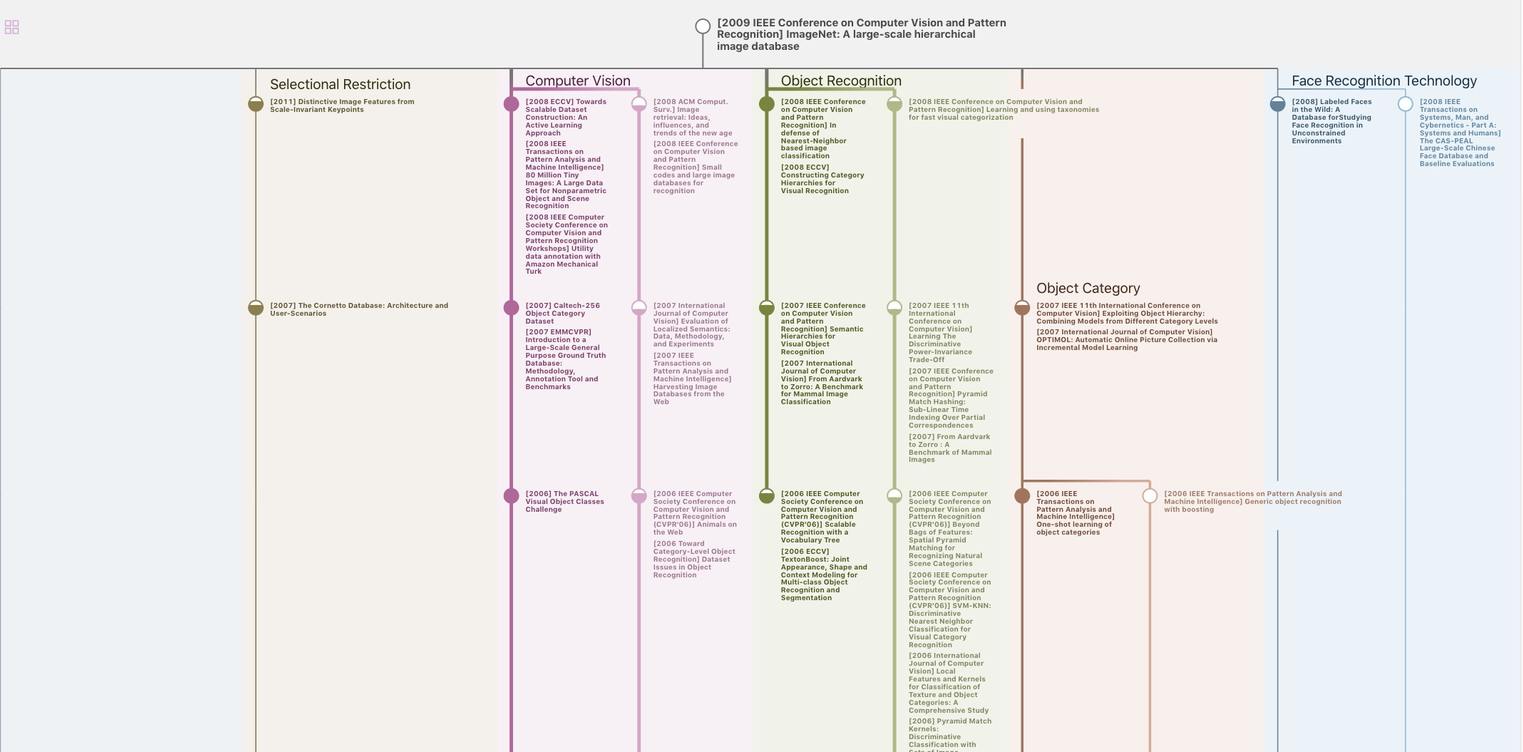
生成溯源树,研究论文发展脉络
Chat Paper
正在生成论文摘要