CA-GCN: A Confidence-aware uncertain knowledge graph embedding model based on graph convolutional networks
2024 27th International Conference on Computer Supported Cooperative Work in Design (CSCWD)(2024)
摘要
Compared with deterministic knowledge graphs, uncertain knowledge graphs can better describe the inherent uncertainty of relations facts in the real world. However, existing embedding models for uncertain knowledge graphs can not capture the direct effect of the confidence score on information propagation between different entities across different relations. This paper proposes a confidence-aware knowledge graph embedding model based on graph convolutional networks (CA-GCN). It enables the propagation of confidence information within the graph structure, allowing confidence scores to directly influence message passing, and ultimately improves the performance of unseen relation facts confidence prediction. To mitigate the adverse effects of false-negative samples during training, we design a collaborative mechanism between a confidence generator and a relation discriminator. The relation discriminator is used to determine if a given triplet is valid, and the confidence generator is used to generate confidence scores. In this way, confidence information of positive and negative samples can play unique roles in different encoders at different stages to avoid the influence of false negatives. Experiments on three widely used datasets show promising results with an obvious performance improvement compared to existing uncertain knowledge embedding models.
更多查看译文
关键词
Knowledge Graph Embedding,Confidence Modeling,Graph Convolutional Networks
AI 理解论文
溯源树
样例
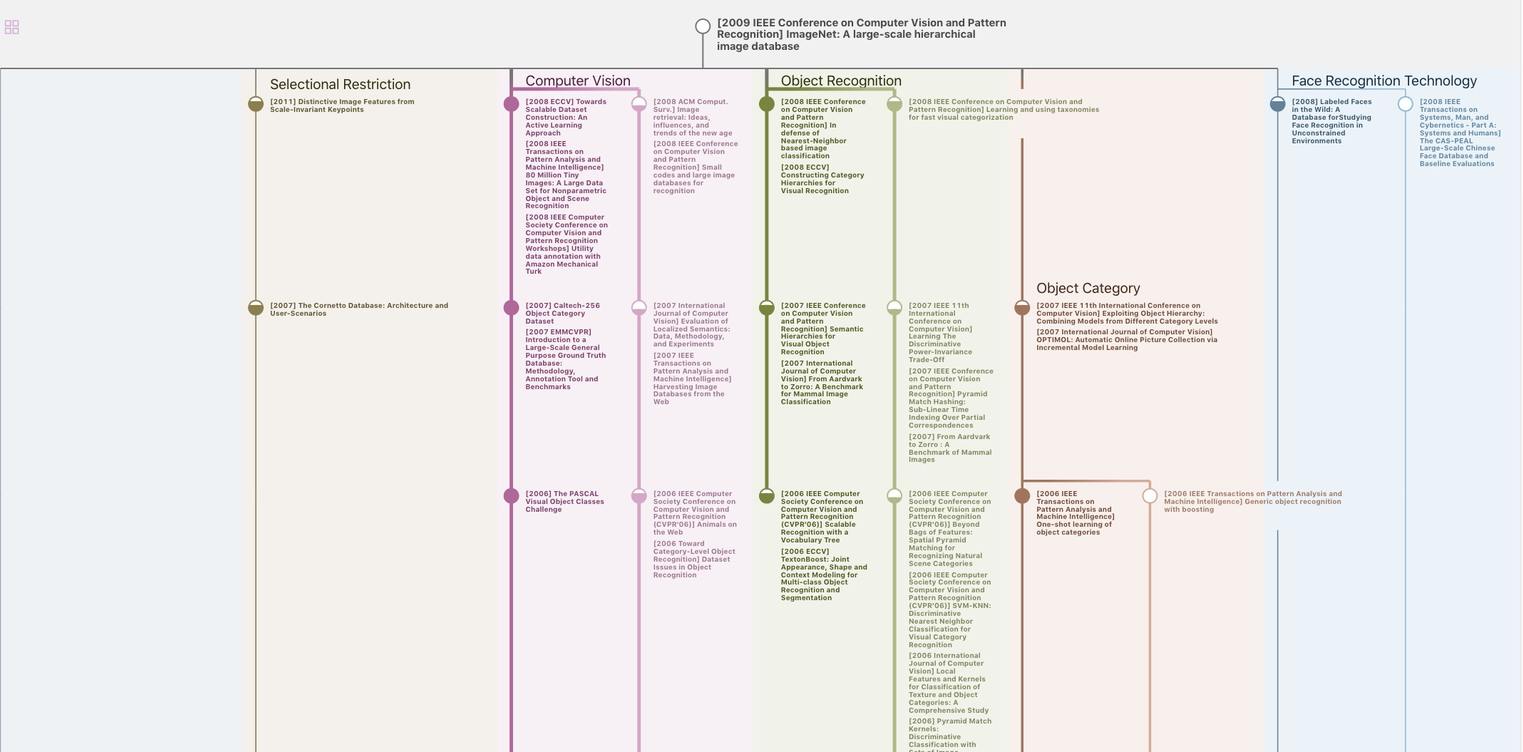
生成溯源树,研究论文发展脉络
Chat Paper
正在生成论文摘要