SemGO: Goal-Oriented Semantic Policy Based on MHSA for Object Goal Navigation
2024 27th International Conference on Computer Supported Cooperative Work in Design (CSCWD)(2024)
摘要
Object Goal Navigation is a task that seeks to allow intelligent agents to locate and navigate to a particular object goal in an unfamiliar environment. However, the current goal-oriented semantic policy, which is based on deep reinforcement learning (DRL), has difficulty in retaining long-term object semantic information and lacks adequate goal-oriented ability. This leads to intelligent agents having to extensively explore their environment in order to locate a goal, resulting in inefficient navigation. To address these challenges, this paper proposes a goal-oriented semantic policy based on multi-headed self-attention (MHSA) to improve the efficiency of object navigation. By using the self-attention mechanism, the policy can automatically learn and extract features relevant to goal navigation without the need for manual feature extractor design. Multiple attention heads can simultaneously focus on various semantic features to extract vital information about the objective goal. We propose a novel object-goal navigation model called SemGO based on this policy. The SemGO model is proficient at managing environments with intricate semantic structures. It can detect the correlation between global and local information, which improves navigation accuracy significantly. Additionally, it has superior generalization capabilities, making it adaptable to changes in different object goals and environments. The experimental results show that the SemGO model achieves a SPL of 0.324, a success rate of 0.635, and a reduction of DTS to 1.601m in the Gibson dataset for object-goal navigation.
更多查看译文
关键词
Semantic Policy,Object Goal Navigation,Multi-headed Self-Attention,Deep Reinforcement Learning
AI 理解论文
溯源树
样例
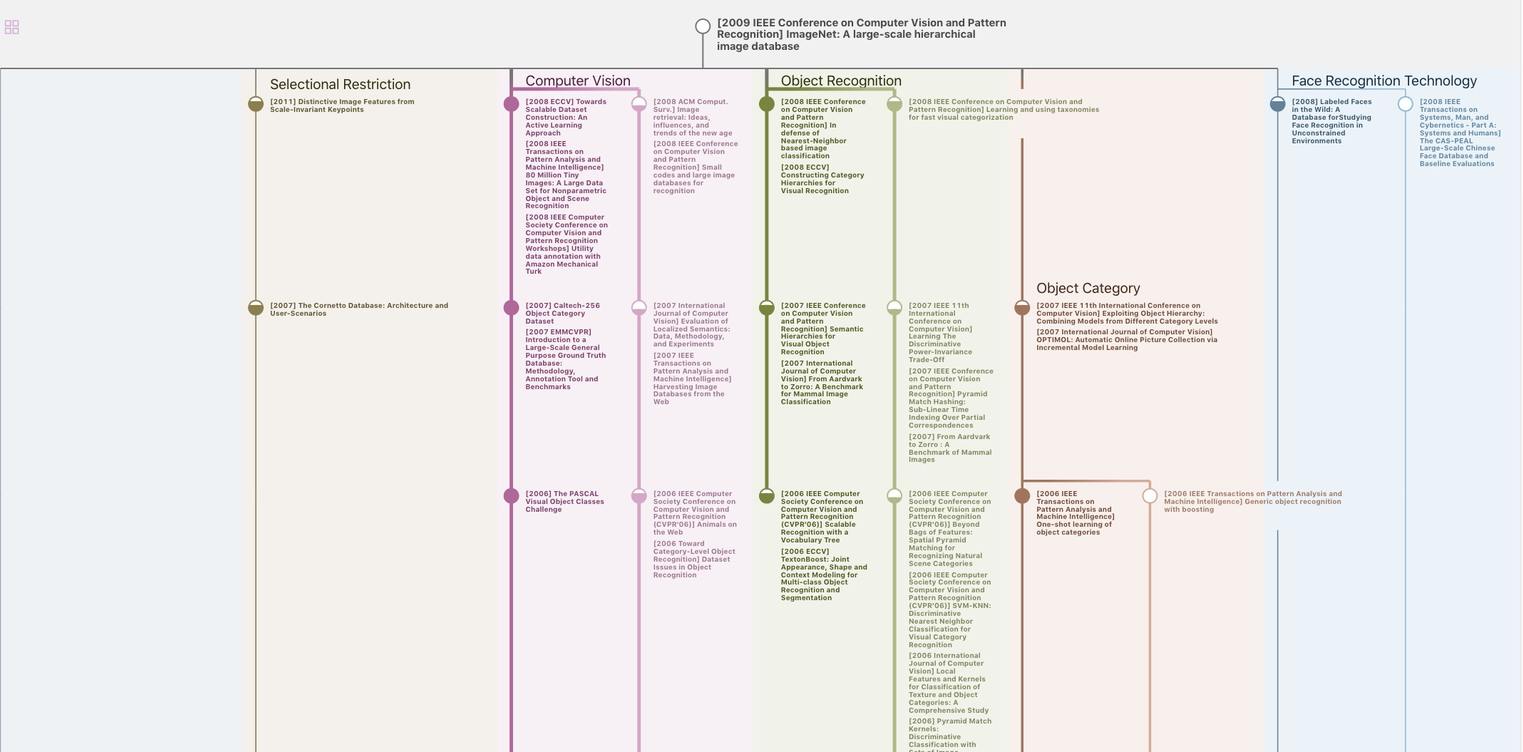
生成溯源树,研究论文发展脉络
Chat Paper
正在生成论文摘要