Deep Dive into Insider Threats: Malicious Activity Detection within Enterprise.
International Conference on Computer Supported Cooperative Work in Design(2024)
摘要
With the digital transformation of enterprises, the increasing complexity of their internal information systems poses a growing challenge in terms of insider threats. Most existing research focuses on user-level and session-level insider threat detection, neglecting activity-level detection, leading to a lack of fine-grained insider threat detection. To tackle the aforementioned issue, we propose MADE, a novel method for detecting malicious activities within enterprise environments. MADE first encodes user multi-source activity logs into activity sequences and learns the semantic representations of activities within the sequences through embedding. Following this, we design an activity detection network based on Bidirectional Long Short-Term Memory (BiLSTM), Convolutional Neural Network (CNN), and Conditional Random Field (CRF). Combining adversarial training, our activity detection network learns the embedded activity sequences and identifies malicious activities. Extensive experimental results on the CERT R4.2 and R5.2 datasets demonstrate the effectiveness of our proposed MADE method.
更多查看译文
关键词
insider threat detection,malicious activity detection,adversarial training,deep learning,enterprise security
AI 理解论文
溯源树
样例
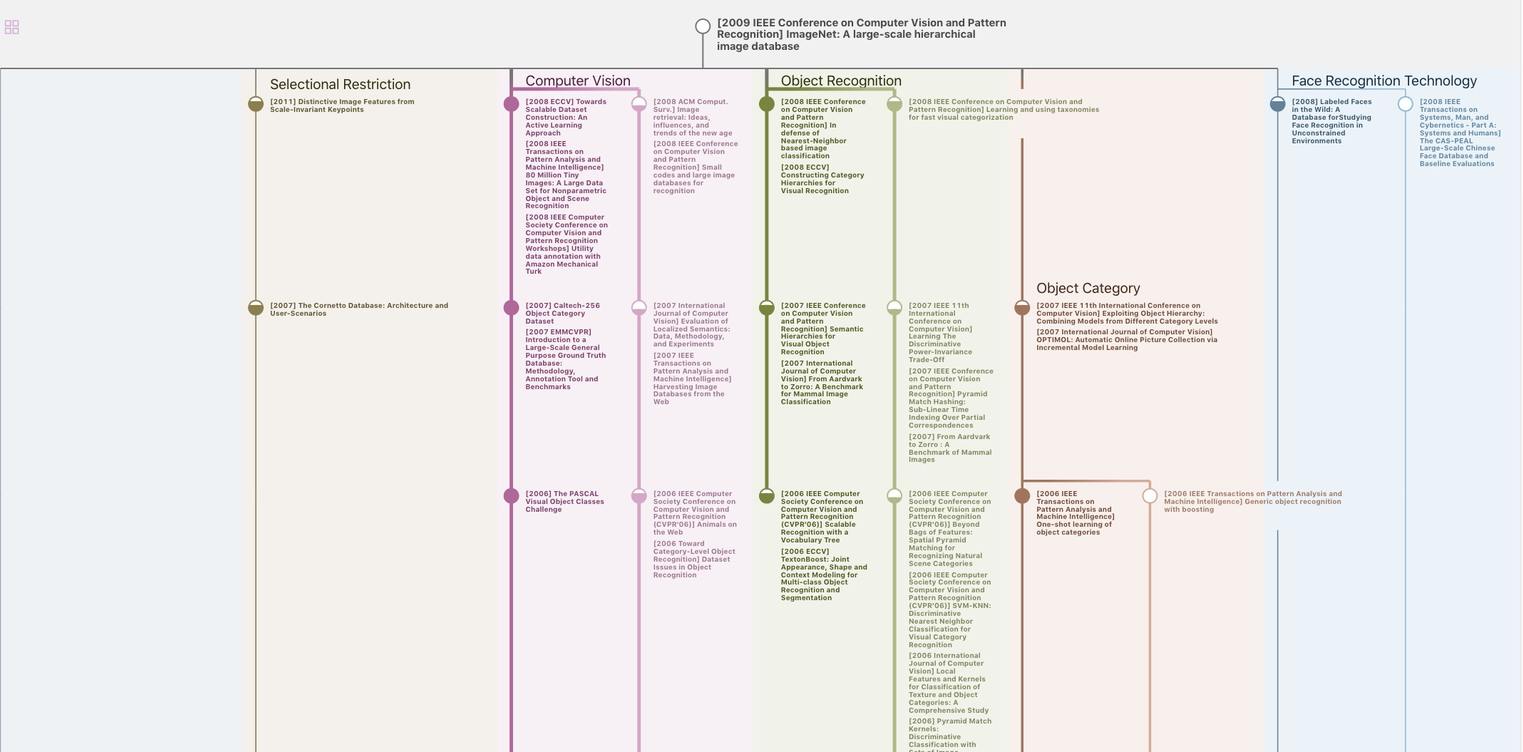
生成溯源树,研究论文发展脉络
Chat Paper
正在生成论文摘要