Dynamic Graph Embedding via Self-Attention in the Lorentz Space.
International Conference on Computer Supported Cooperative Work in Design(2024)
摘要
Graph Neural Networks (GNNs) are popular for learning node representations in complex graph structures. Traditional methods use Euclidean space but struggle to capture hierarchical structures in real-world graphs. Besides, it’s important to note that in practical applications, many graphs are dynamic and undergo continuous evolution over time. To investigate the characteristics of complex temporal networks, we have introduced a dynamic graph embedding model in the Lorentz space, building upon the foundation of the previously proposed DynHAT model. More specially, our model divides the dynamic graph into multiple discrete static graphs, maps each static graph to the Lorentz space, and then learns informative node representations over time using a self-attention mechanism. We have conducted link prediction experiments on two types of graphs: communication networks and rating networks. Through comprehensive experiments conducted on five real-world datasets, we have demonstrated the superiority of our model in embedding dynamic graphs within Lorentz space.
更多查看译文
关键词
lorentz,dynamic graph,self-attention,
AI 理解论文
溯源树
样例
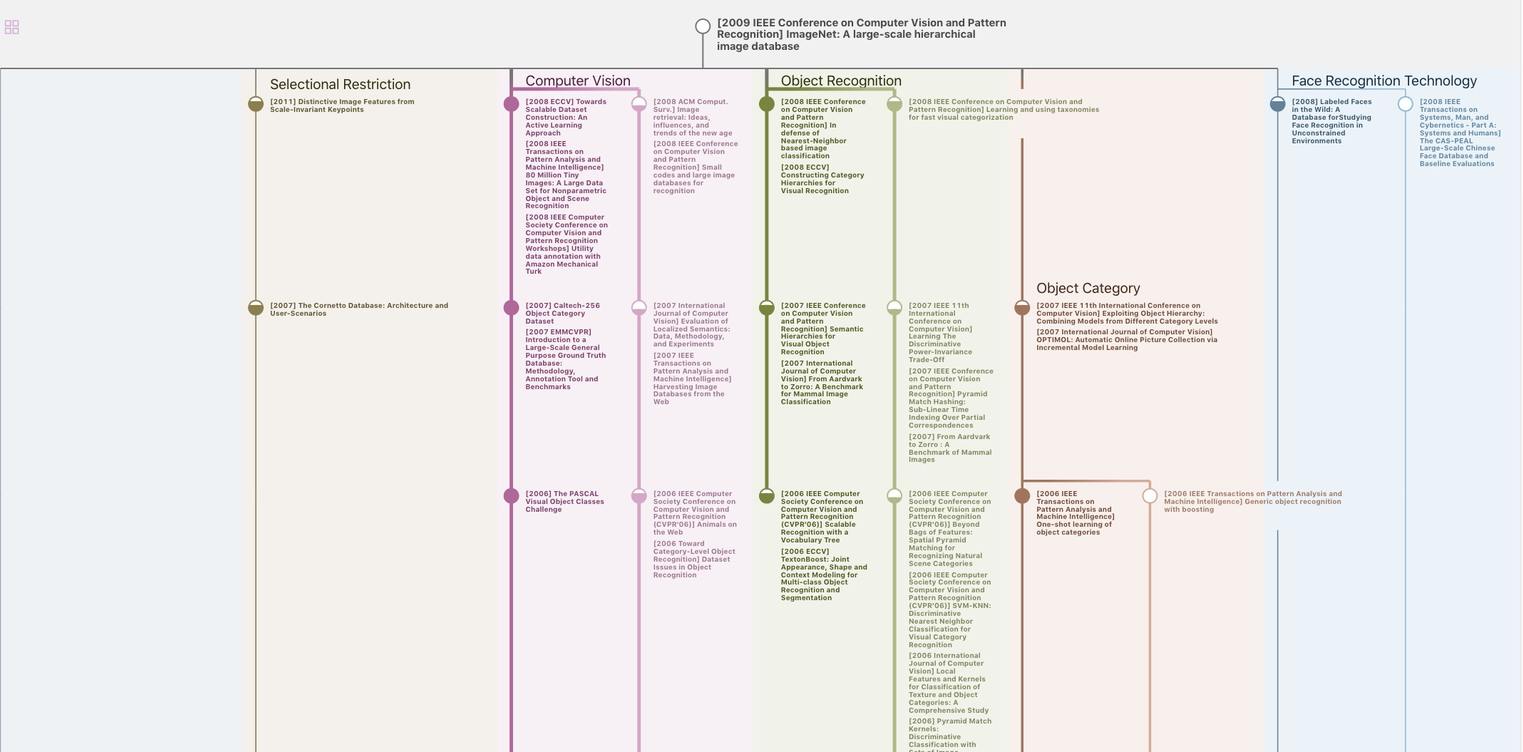
生成溯源树,研究论文发展脉络
Chat Paper
正在生成论文摘要