3D Reconstruction and Rendering Based on Improved Neural Radiance Field
2024 3rd International Conference on Image Processing and Media Computing (ICIPMC)(2024)
摘要
In this paper, we propose a 3D reconstruction and rendering method based on the improved neural radiance fields. To address the inefficiency of the spatial sampling process in the neural radiance fields, we employ a depth-guided light sampling method to obtain more accurate 3D points. We propose a monocular geometrically supervised volume rendering method to address the missing depth and normal information. This method uses the depth map and normal map estimated by the dual-stream network as input, allowing the neural radiance fields to learn more depth and normal information. Simultaneously, to tackle the issue of imprecise pose estimation, we propose a strategy for inter-frame constraints to enhance the optimization of the camera pose by utilizing geometric consistency and photometric consistency losses. Compared with other algorithms based on neural radiance fields, this method improves the new view rendering index PSNR by at least 0.355 on average and the pose estimation index ATE by at least 0.025m on average in the ScanNet and Tanks and Temples datasets. Additionally, Compared with Nerfacto, a neural radiance fields improvement algorithm, this method improves the F-score index of the 3D point cloud in the Tanks and Temples dataset by 1.49.
更多查看译文
关键词
Neural radiance fields,3D Reconstruction,Dual-stream network,Inter-frame constraints
AI 理解论文
溯源树
样例
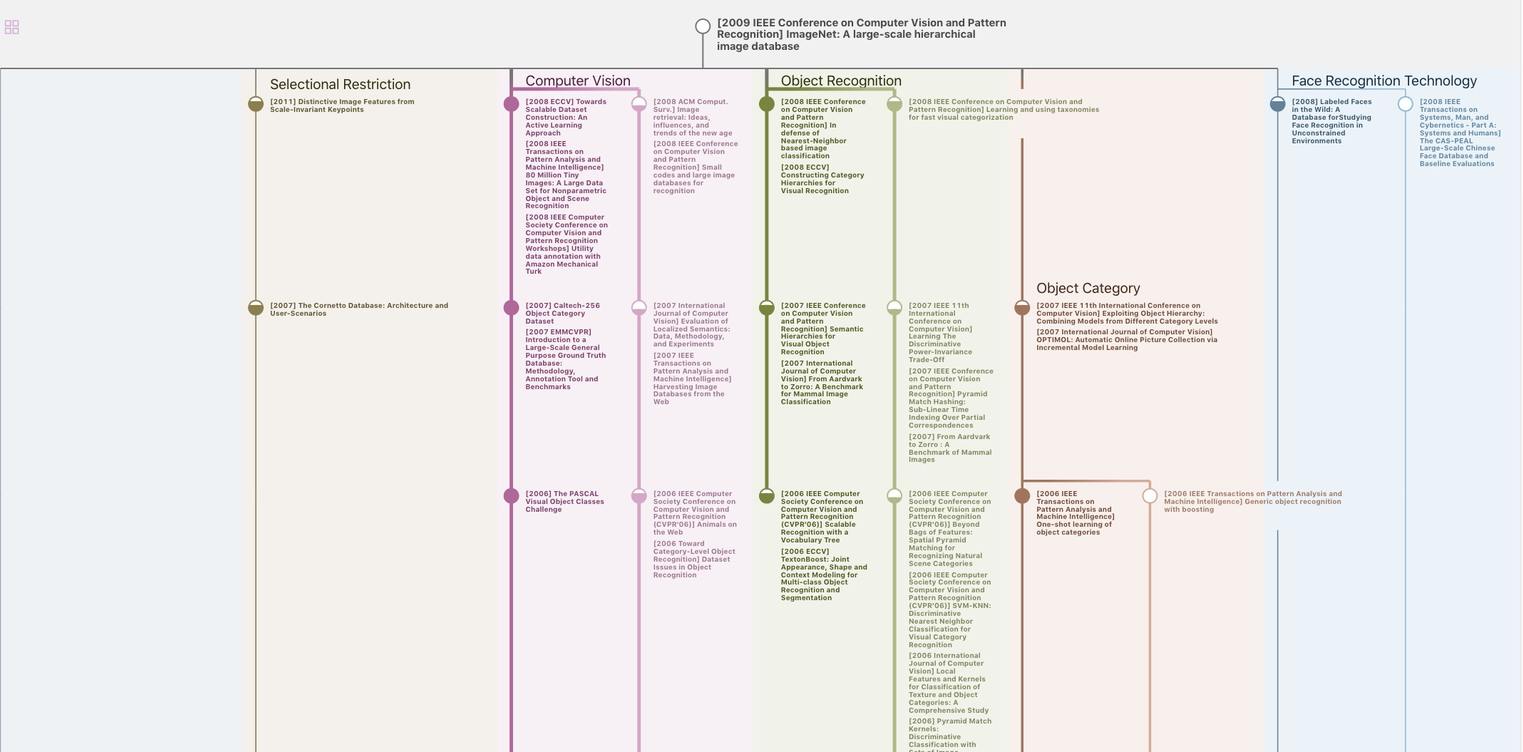
生成溯源树,研究论文发展脉络
Chat Paper
正在生成论文摘要